Facial Expression Recognition Based on Feature Fusion and Sparse Representation
ICMLC(2017)
摘要
Aiming at the limitation of single feature classification, this paper proposes a facial expression recognition algorithm based on feature fusion and sparse representation residual fusion. The proposed method is composed of three components. Firstly, a feature fusion method based on local binary quantization (LBP) and local phase quantization (LPQ) is proposed. The LBP/LPQ features are an expression feature with image space and frequency domain. Second, Curvelet transform is used to extract the global features of facial expression, which is a very effective multi-scale, band-pass and direction analysis tool. Finally, the LBP/LPQ features and the Curvelet feature are classified by the sparse representation based classification (SRC) with stronger discrimination and robustness respectively. According to the sparse residuals of LBP/LPQ+SRC and Curvelet+SRC, a classification method of residual fusion is proposed. Experiments on JAFFE database and Cohn-Kanade database show that the proposed method effectively improves the ability of facial expression recognition and achieves a high recognition rate.
更多查看译文
AI 理解论文
溯源树
样例
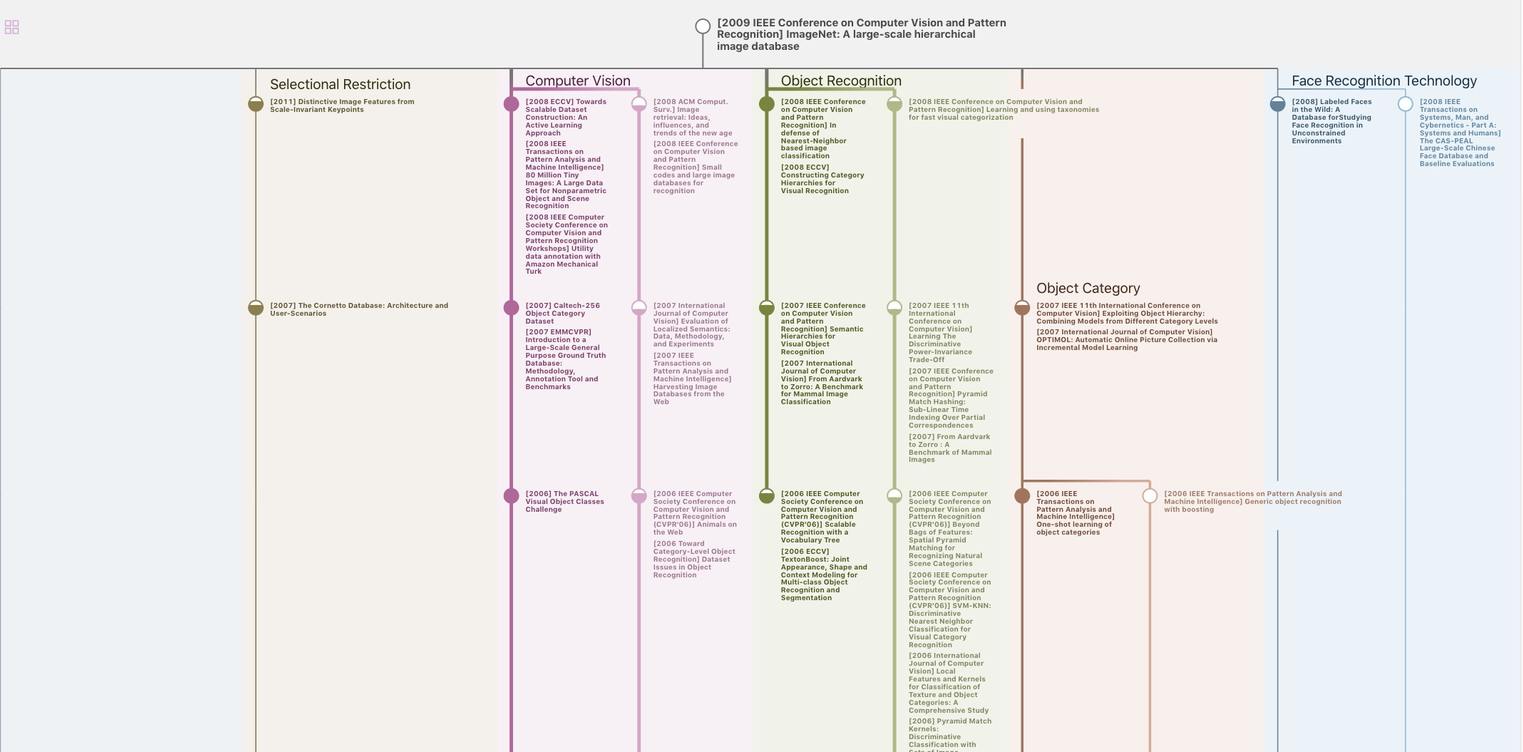
生成溯源树,研究论文发展脉络
Chat Paper
正在生成论文摘要