An efficient validity index method for datasets with complex-shaped clusters
2016 International Conference on Machine Learning and Cybernetics (ICMLC)(2016)
摘要
In this paper, a validity index method VDOGK, a variation of the index method VDO, for estimating the optimal number of clusters in datasets with concave-/elongated-shaped clusters is presented. The new index uses Gustafson-Kessel FCM to partition the dataset so that geometric-shape-sensitivity problem of FCM can be reduced. It is based on both dispersion and overlap measures, where the dispersion measure estimates the overall cluster compactness and the overlap measure estimates the total ambiguity degree of data belonging to any pair of clusters in the dataset. A good clustering result is expected to have both measures small. Examples of synthetic datasets comprising concave, elongated, spherical, and/or elliptical clusters are presented. Experimental results on various datasets including synthetic and real datasets from UCI Machine Learning Laboratory demonstrate that the proposed VDOGK made correct estimation on number of clusters for all nine tested datasets, whereas VDO only scored three real datasets.
更多查看译文
关键词
Cluster validity index,GKFCM,Dispersion measure,Overlap measure,Concave shape
AI 理解论文
溯源树
样例
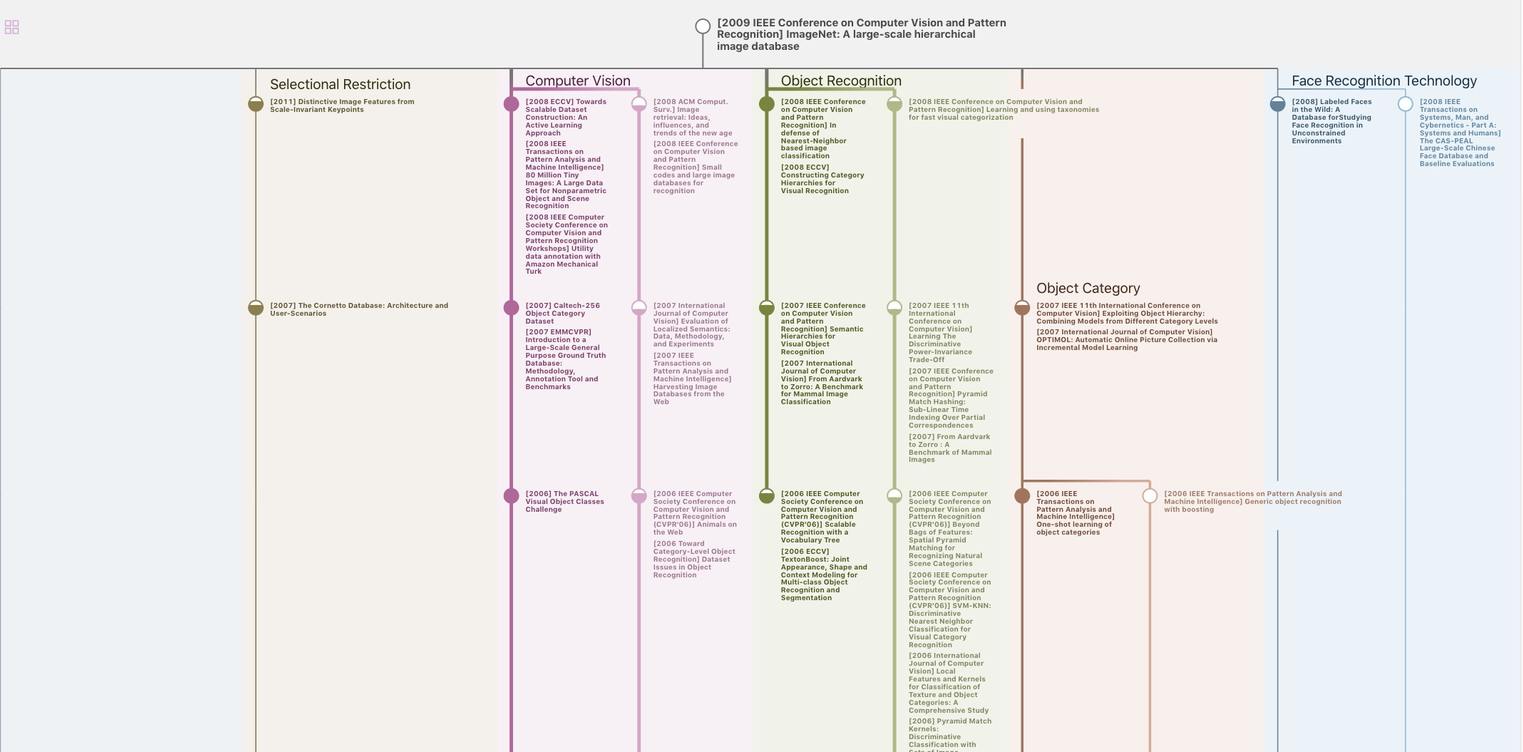
生成溯源树,研究论文发展脉络
Chat Paper
正在生成论文摘要