High-order boltzmann machine-based unsupervised feature learning for multi-atlas segmentation
2017 IEEE 14TH INTERNATIONAL SYMPOSIUM ON BIOMEDICAL IMAGING (ISBI 2017)(2017)
摘要
Multi-atlas based label fusion methods have been successfully used for medical image segmentation. In the field of brain region segmentation, multi-atlas based methods propagate labels from multiple atlases to target image by the similarity between patches in target image and atlases. Most of existing multi-atlas based methods usually use intensity feature, which is hard to capture high-order information in brain images. In light of this, in this paper, we endeavor to apply high-order restricted Boltzmann machines to represent brain images and use the learnt feature for brain region of interesting (ROIs) segmentation. Specifically, we firstly capture the covariance and the mean information from patches by high-order Boltzmann Machine. Then, we propagate the label by the similarity of the learnt high-order features. We validate our feature learning method on two well-known label fusion methods e.g., local-weighted voting (LWV) and non-local mean patch-based method (PBM). Experimental results on the NIREP dataset demonstrate that our method can improve the performance of both LWV and PBM by using the high-order features.
更多查看译文
关键词
Multi-atlas,Label fusion,High-order restricted Boltzmann machines
AI 理解论文
溯源树
样例
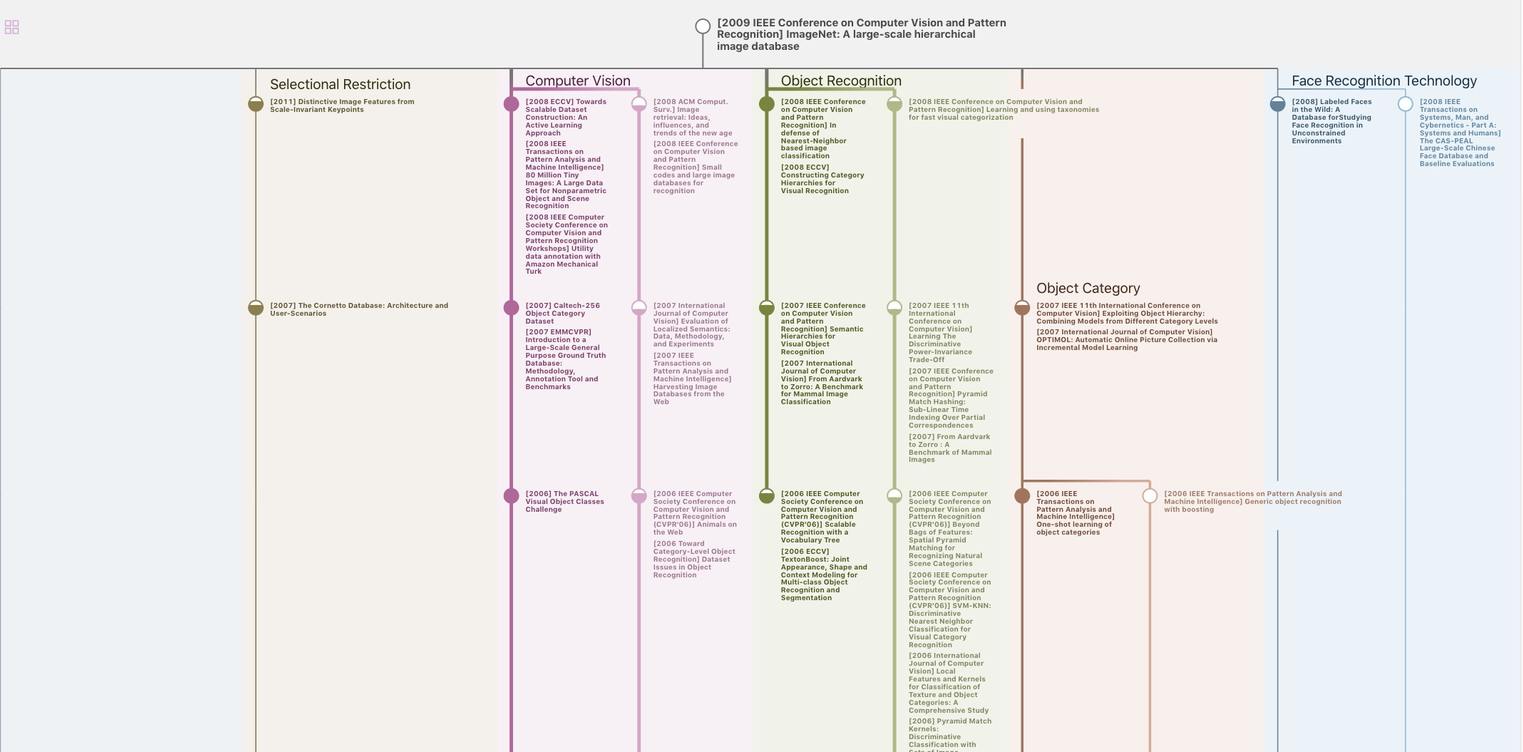
生成溯源树,研究论文发展脉络
Chat Paper
正在生成论文摘要