Handcrafted Features Vs Convnets In 2d Echocardiographic Images
2017 IEEE 14TH INTERNATIONAL SYMPOSIUM ON BIOMEDICAL IMAGING (ISBI 2017)(2017)
摘要
In this paper, we address the problem of automated pose classification and segmentation of the left ventricle (LV) in 2D echocardiographic images. For this purpose, we compare two complementary approaches. The first one is based on engineering ad-hoc features according to the traditional machine learning paradigm. Namely, we extract phase features to build an unsupervised LV pose estimator, as well as a global image descriptor for view type classification. We also apply the Supervised Descent Method (SDM) to iteratively refine the LV contour. The second approach follows the deep learning framework, where a Convolutional Network (ConvNet) learns the visual features automatically. Our experiments on a large database of apical sequences show that the two approaches yield comparable results on view classification, but SDM outperforms ConvNet on LV segmentation at a significantly lower training computational cost.
更多查看译文
关键词
2D ultrasound, left ventricle segmentation, phase features, Supervised Descent Method, ConvNets
AI 理解论文
溯源树
样例
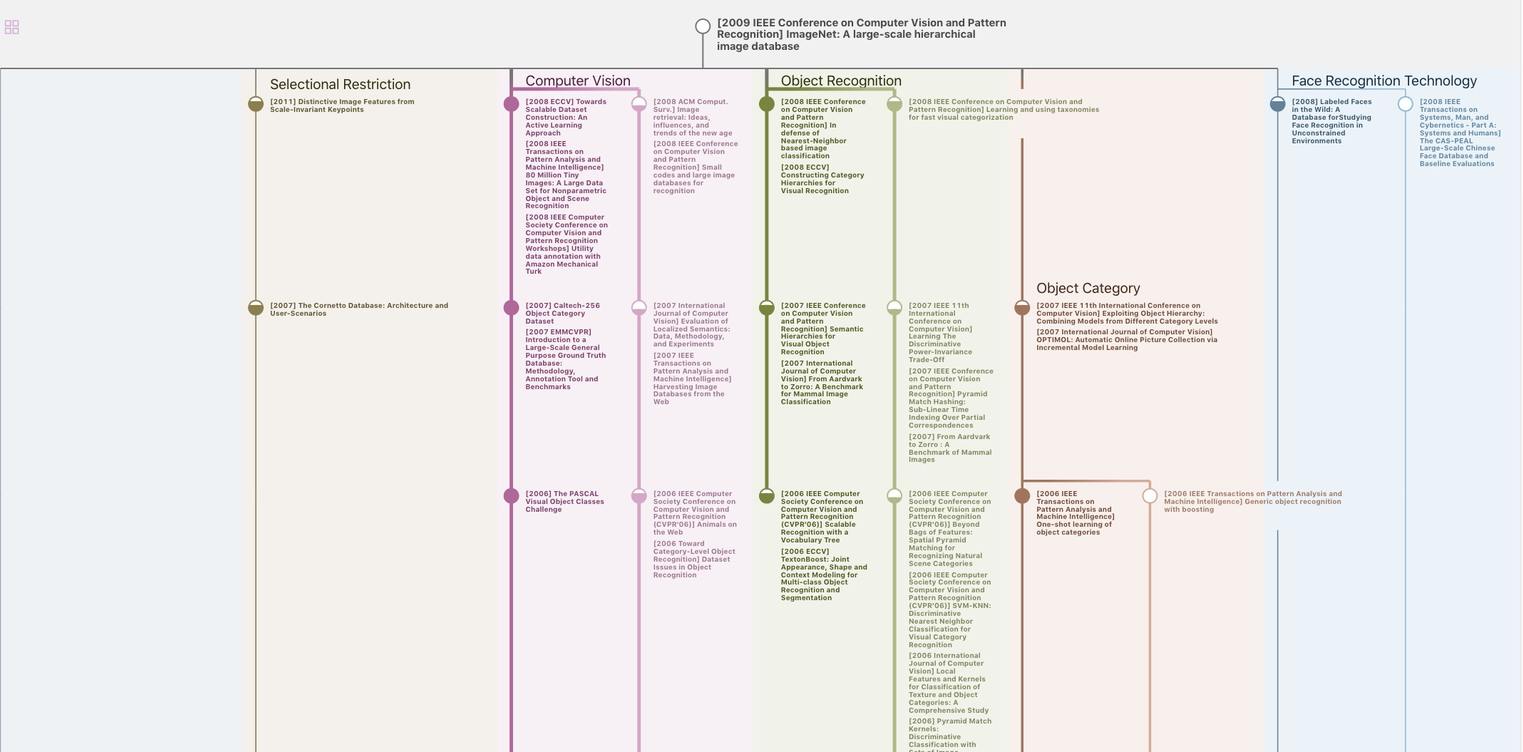
生成溯源树,研究论文发展脉络
Chat Paper
正在生成论文摘要