Fast anatomy segmentation by combining low resolution multi-atlas label fusion with high resolution corrective learning: An experimental study
2017 IEEE 14th International Symposium on Biomedical Imaging (ISBI 2017)(2017)
摘要
Deformable registration based multi-atlas segmentation has been successfully applied in a broad range of anatomy segmentation applications. However, the excellent performance comes with a high computational burden due to the requirement for deformable image registration and voxel-wise label fusion. To address this problem, we conduct an experimental study to investigate trade-off between computational cost and performance by first applying multi-atlas segmentation in coarse spatial resolution and then refining the results by learning-based error correction in the native image space. In a cardiac CT segmentation application, our experiments show that the new combination scheme can significantly reduce computational cost without losing accuracy.
更多查看译文
关键词
Multi-Atlas Segmentation,Corrective Learning
AI 理解论文
溯源树
样例
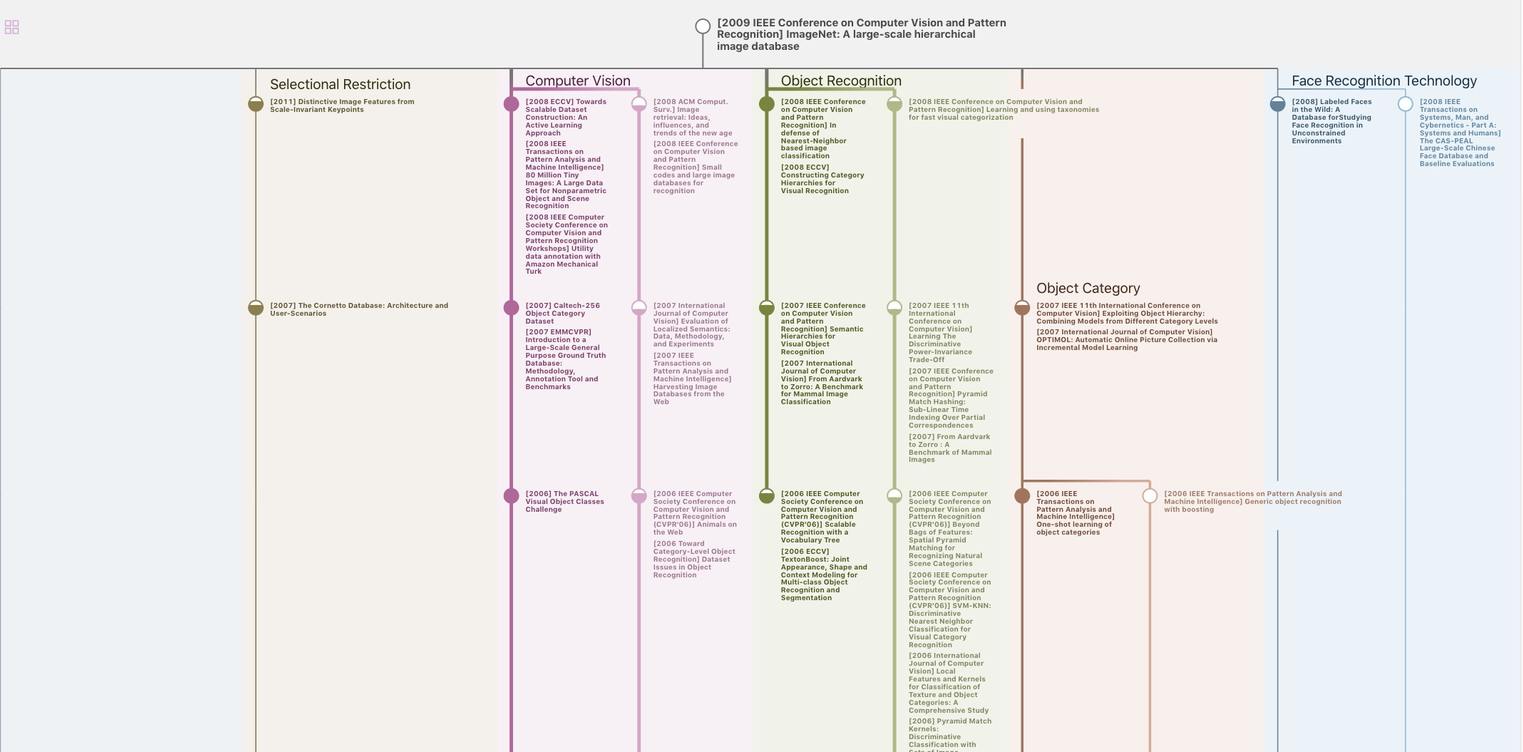
生成溯源树,研究论文发展脉络
Chat Paper
正在生成论文摘要