Resource Constrained Structured Prediction.
THIRTY-FIRST AAAI CONFERENCE ON ARTIFICIAL INTELLIGENCE(2017)
摘要
We study the problem of structured prediction under test-time budget constraints. We propose a novel approach based on selectively acquiring computationally costly features during test-time in order to reduce the computational cost of prediction with minimal performance degradation. We formulate a novel empirical risk minimization (ERM) for policy learning. We show that policy learning can be reduced to a series of structured learning problems, resulting in efficient training using existing structured learning algorithms. This framework provides theoretical justification for several existing heuristic approaches found in literature. We evaluate our proposed adaptive system on two structured prediction tasks, optical character recognition and dependency parsing and show significant reduction in the feature costs without degrading accuracy.
更多查看译文
AI 理解论文
溯源树
样例
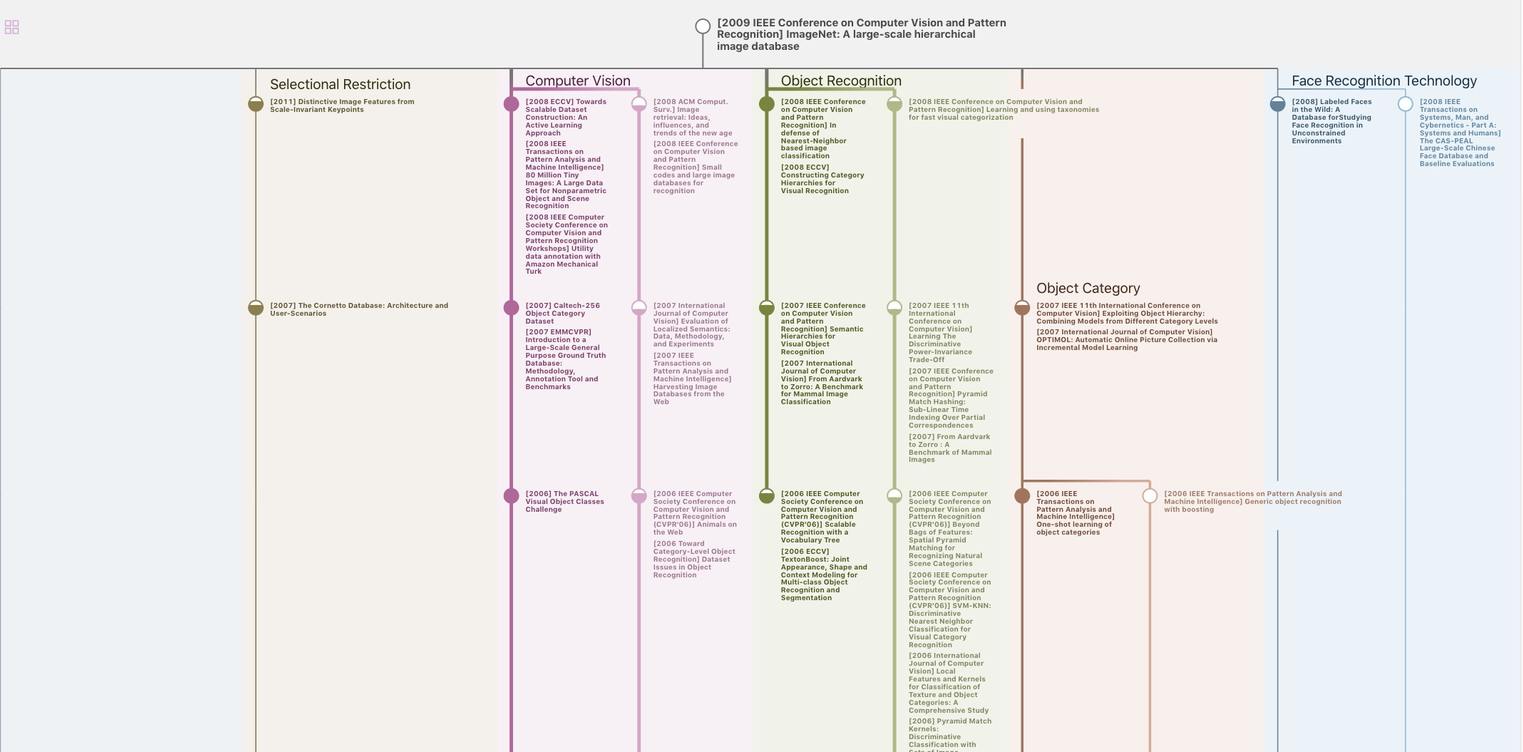
生成溯源树,研究论文发展脉络
Chat Paper
正在生成论文摘要