Extracting Urban Microclimates from Electricity Bills.
THIRTY-FIRST AAAI CONFERENCE ON ARTIFICIAL INTELLIGENCE(2017)
摘要
Sustainable energy policies are of growing importance in all urban centers. Climate - and climate change - will play increasingly important roles in these policies. Climate zones defined by the California Energy Commission have long been influential in energy management. For example, recently a two-zone division of Los Angeles (defined by historical temperature averages) was introduced for electricity rate restructuring. The importance of climate zones has been enormous, and climate change could make them still more important. AI can provide improvements on the ways climate zones are derived and managed. This paper reports on analysis of aggregate household electricity consumption (EC) data from local utilities in Los Angeles, seeking possible improvements in energy management. In this analysis we noticed that EC data permits identification of interesting geographical zones - regions having EC patterns that are characteristically different from surrounding regions. We believe these zones could be useful in a variety of urban models. Microclimates are characterized by common patterns of environmental variables, such as temperature, wind, humidity, and vegetation. Our key finding is that regions in which block groups have similar Electricity Consumption patterns over time are topographically-defined basins having common climate patterns. We call these EC-microclimate zones. Because they reflect local topography and climate, but are based only on EC data, they permit development of new solutions with learning methods. Although microclimates are known to play important roles in energy management, and are important aspects of ecosystems for computational sustainability, the complexity of environmental variables makes them hard to quantify. We analyze how microclimates and household electricity consumption are linked to socioeconomic variables like income. We show how learning-based models can be useful in prediction of energy consumption. Related geostatistical methods also can be used to extend models for each microclimate zone to larger-scale models of electricity consumption.
更多查看译文
AI 理解论文
溯源树
样例
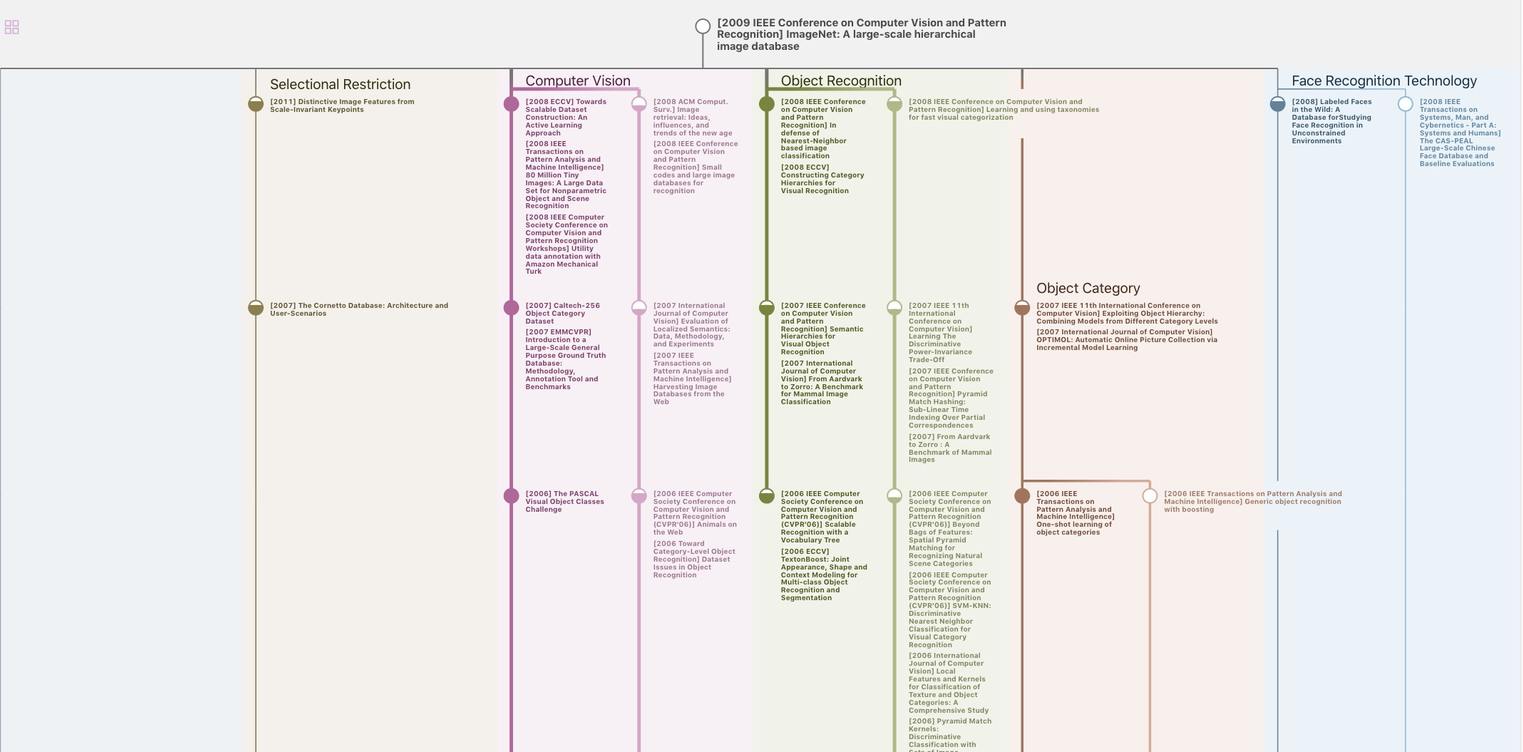
生成溯源树,研究论文发展脉络
Chat Paper
正在生成论文摘要