Unsupervised Learning of Semantics of Object Detections for Scene Categorization
dblp(2015)
摘要
Classifying scenes (e.g. into “street”, “home” or “leisure”) is an important but complicated task nowadays, because images come with variability, ambiguity, and a wide range of illumination or scale conditions. Standard approaches build an intermediate representation of the global image and learn classifiers on it. Recently, it has been proposed to depict an image as an aggregation of its contained objects: the representation on which classifiers are trained is composed of many heterogeneous feature vectors derived from various object detectors. In this paper, we propose to study different approaches to efficiently learn contextual semantics out of these object detections. We use the features provided by Object-Bank [24] (177 different object detectors producing 252 attributes each), and show on several benchmarks for scene categorization that careful combinations, taking into account the structure of the data, allows to greatly improve over original results (from \(+5\) to \(+11\,\%\)) while drastically reducing the dimensionality of the representation by 97 % (from 44,604 to 1,000). We also show that the uncertainty relative to object detectors hampers the use of external semantic knowledge to improve detectors combination, unlike our unsupervised learning approach.
更多查看译文
关键词
Unsupervised learning, Transfer learning, Deep learning, Scene categorization, Object detection
AI 理解论文
溯源树
样例
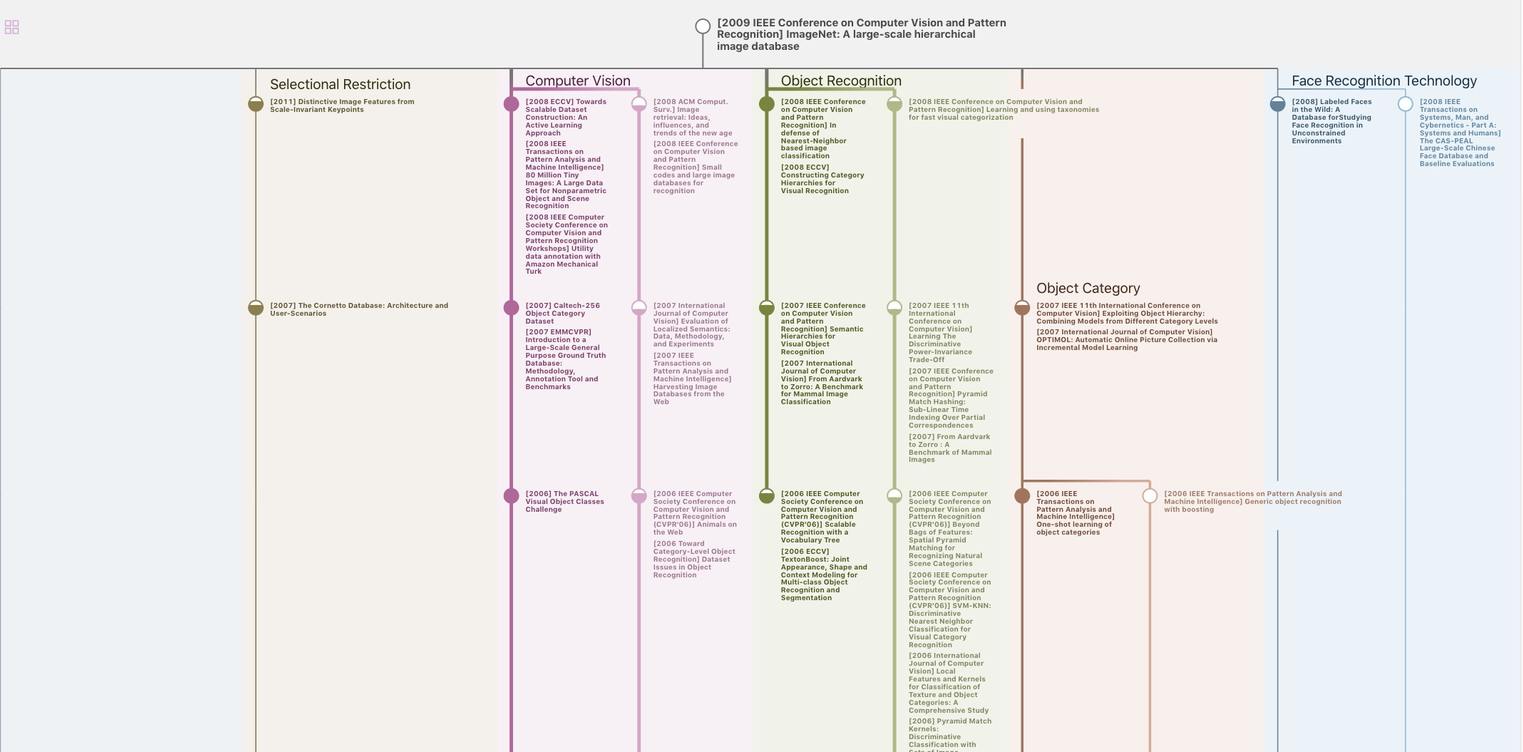
生成溯源树,研究论文发展脉络
Chat Paper
正在生成论文摘要