A Hierarchical Tree Distance Measure For Classification
ICPRAM: PROCEEDINGS OF THE 6TH INTERNATIONAL CONFERENCE ON PATTERN RECOGNITION APPLICATIONS AND METHODS(2017)
摘要
In this paper, we explore the problem of classification where class labels exhibit a hierarchical tree structure. Many multiclass classification algorithms assume a flat label space, where hierarchical structures are ignored. We take advantage of hierarchical structures and the interdependencies between labels. In our setting, labels are structured in a product and service hierarchy, with a focus on spend analysis. We define a novel distance measure between classes in a hierarchical label tree. This measure penalizes paths though high levels in the hierarchy. We use a known classification algorithm that aims to minimize distance between labels, given any symmetric distance measure. The approach is global in that it constructs a single classifier for an entire hierarchy by embedding hierarchical distances into a lower-dimensional space. Results show that combining our novel distance measure with the classifier induces a trade-off between accuracy and lower hierarchical distances on misclassifications. This is useful in a setting where erroneous predictions vastly change the context of a label.
更多查看译文
关键词
Machine Learning, Multi-class Classification, Hierarchical Classification, Tree Distance Measures, Multi-output Regression, Multidimensional Scaling, Process Automation, UNSPSC
AI 理解论文
溯源树
样例
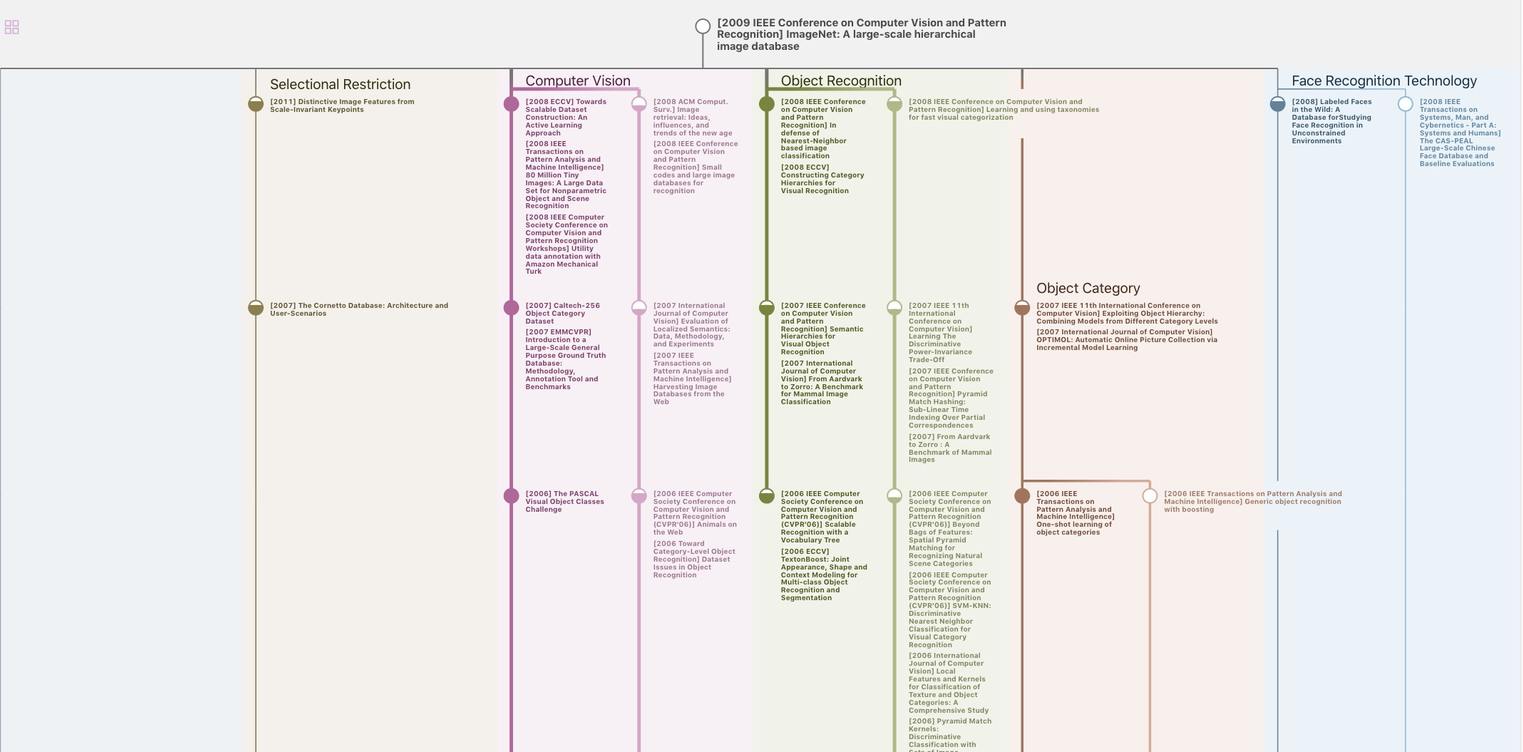
生成溯源树,研究论文发展脉络
Chat Paper
正在生成论文摘要