A Generative Model With Hypergraph Regularizers For Protein Function Prediction
2017 INTERNATIONAL JOINT CONFERENCE ON NEURAL NETWORKS (IJCNN)(2017)
摘要
Heterogeneous data sources and multi-label are two important characteristics of protein function prediction. They describe protein data from two different aspects. However, it is of considerable challenge to integrate multiple data sources and multi-label simultaneously for predicting protein functions, especially when there are only a limited number of labeled proteins. In this paper, we propose a generative model with hypergraph regularizers algorithm, called GMHR, for predicting proteins with multiple functions. The GMHR algorithm integrates all data sources that are available, including protein attribute features, interaction networks, label correlations, and unlabeled data. Experimental results on the real-world datasets predicting the functions of proteins demonstrate the superiority of our proposed method compared with the state-of-the-art baselines.
更多查看译文
关键词
generative model,protein function prediction,heterogeneous data sources,multilabel data,protein data,multiple data sources,labeled proteins,hypergraph regularizers algorithm,GMHR algorithm,protein attribute features,interaction networks,label correlations,unlabeled data
AI 理解论文
溯源树
样例
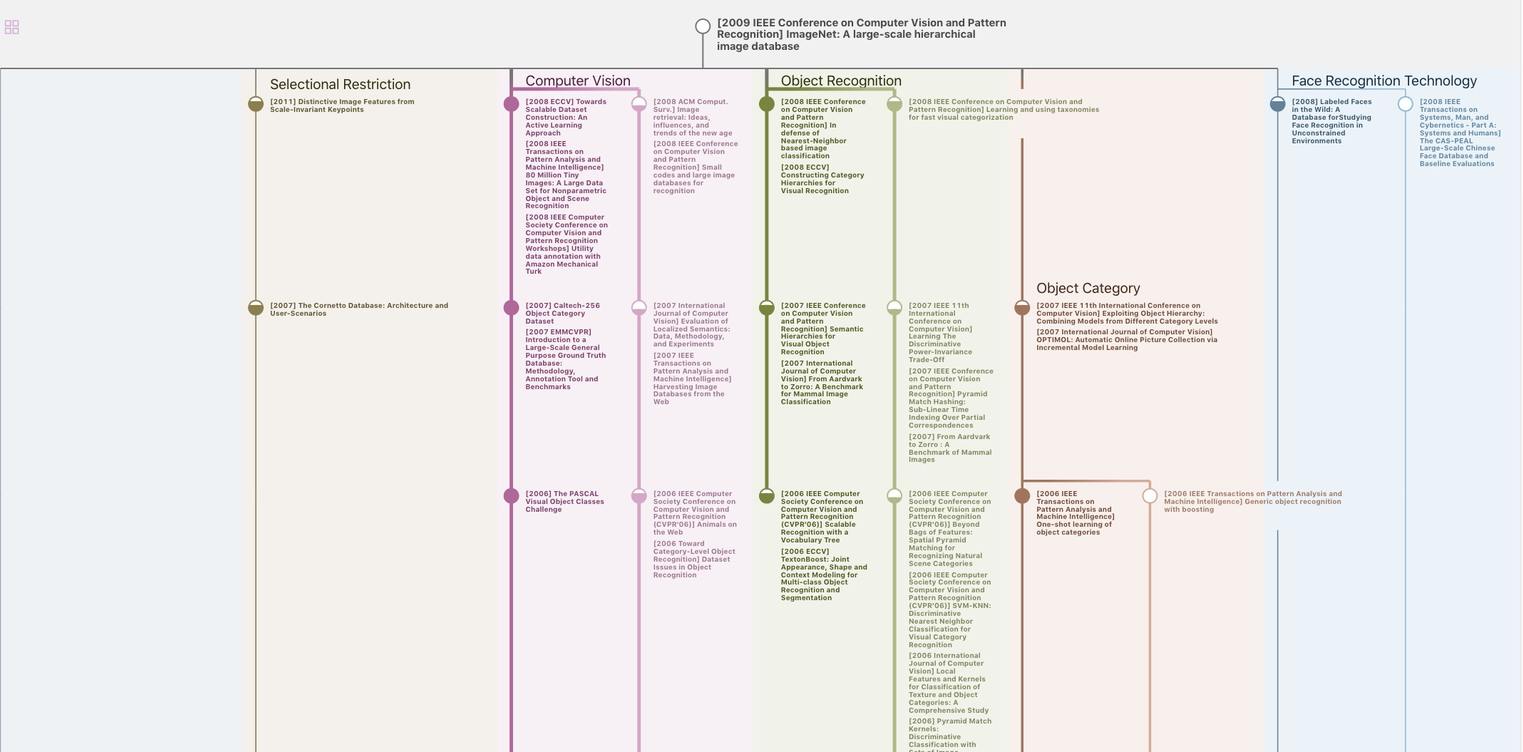
生成溯源树,研究论文发展脉络
Chat Paper
正在生成论文摘要