Distance Metric Learning With Eigenvalue Fine Tuning
2017 INTERNATIONAL JOINT CONFERENCE ON NEURAL NETWORKS (IJCNN)(2017)
摘要
Distance metric learning focuses on learning one global or multiple local distance functions to draw similar instances close to each other and push away dissimilar ones. Most existing work has to do matrix projection to learn distance functions. In this paper, we present a novel distance function learning model which is based on eigenvalue fine tuning. Our model not only is able to learn the global distance function but also can be easily adopted into local metric learning tasks. From the perspective of dimension reduction, the proposed model can measure how much information has been preserved after feature transformation. Moreover, we connect our model with principal components analysis to improve its performance by introducing the label information. Experimental results have demonstrated the effectiveness of the proposed method.
更多查看译文
关键词
distance metric learning,eigenvalue fine tuning,matrix projection,distance function learning model,dimension reduction perspective,principal component analysis,label information
AI 理解论文
溯源树
样例
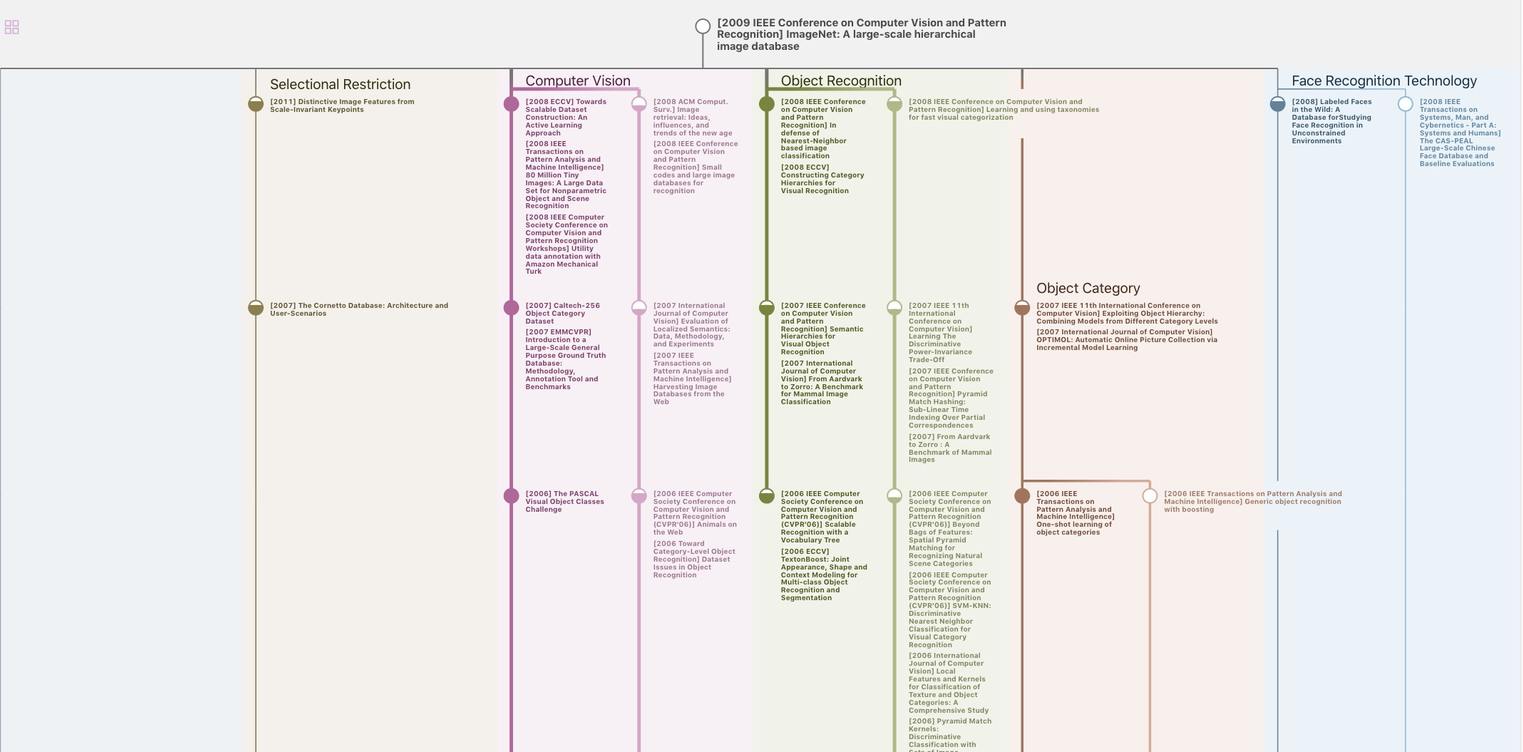
生成溯源树,研究论文发展脉络
Chat Paper
正在生成论文摘要