Learning Deep Representations With Diode Loss For Quantization-Based Similarity Search
2017 INTERNATIONAL JOINT CONFERENCE ON NEURAL NETWORKS (IJCNN)(2017)
摘要
Recent advance of large scale similarity search involves using deeply learned representations to improve the search accuracy and apply vector quantization techniques to accelerate the search speed. However, simultaneous learning of deep representations and vector quantizers still remains ineffective. To this end, we propose to directly optimize the asymmetric distance between a query representation and the quantized database representations. A novel diode loss is proposed, it wraps a commonly used similarity loss function and then it allows effective end-to-end learning of both deep representations and vector quantizers with a siamese network. The proposed learning framework is compatible with various existing vector quantization approaches, and is compatible with commonly used loss functions for learning representations preserving similarities. Experimental results demonstrate that the proposed framework is effective, flexible and outperforms the state-of-the-art large scale similarity search methods.
更多查看译文
关键词
deep representations,diode loss,quantization-based similarity search,large scale similarity search,search accuracy improvement,vector quantization,search speed,simultaneous learning,asymmetric distance optimization,query representation,quantized database representations,similarity loss function,end-to-end learning,siamese network
AI 理解论文
溯源树
样例
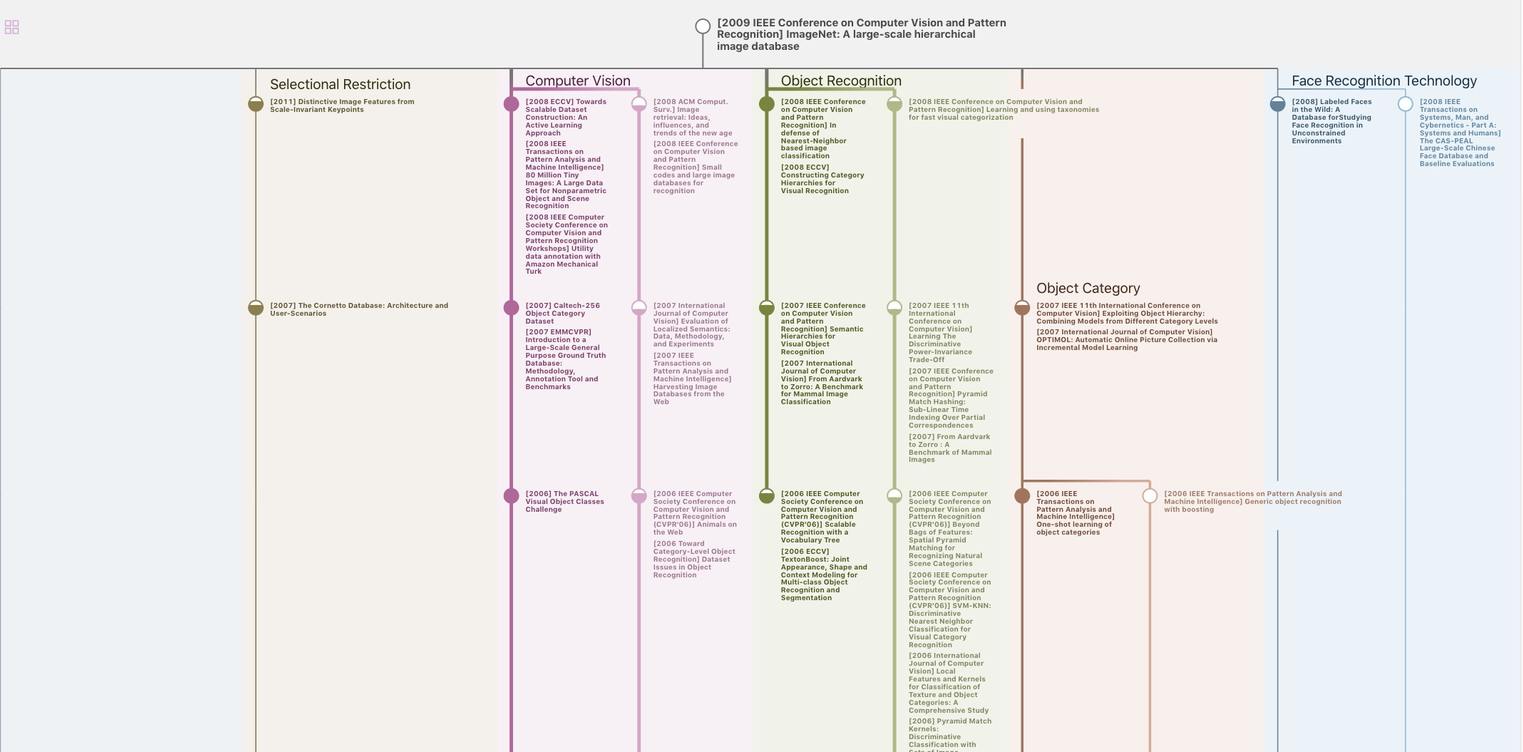
生成溯源树,研究论文发展脉络
Chat Paper
正在生成论文摘要