Solar Power Prediction With Data Source Weighted Nearest Neighbors
2017 INTERNATIONAL JOINT CONFERENCE ON NEURAL NETWORKS (IJCNN)(2017)
摘要
We consider the task of predicting the half-hourly solar PhotoVoltaic (PV) power output for the next day from three sources: previous solar power, previous weather data and weather forecasts. We propose DWkNN, a data source weighed nearest neighbor method that considers the importance of the different data sources and learns the best weights for them. We evaluate its performance using Australian PV and weather data for one year. Our results show that DWkNN is more accurate than the standard nearest neighbor and other machine learning, statistical and persistence methods used for comparison. We assess the importance of the three data sources to predict the PV power output and show that the most useful information source is the weather forecast, followed by the previous weather and previous PV power data. The addition of the daily average solar irradiance forecast for the next day, when this information is available, considerably improves the accuracy.
更多查看译文
关键词
solar power forecasting, time series prediction, multiple data sources, data source weighted nearest neighbor algorithm
AI 理解论文
溯源树
样例
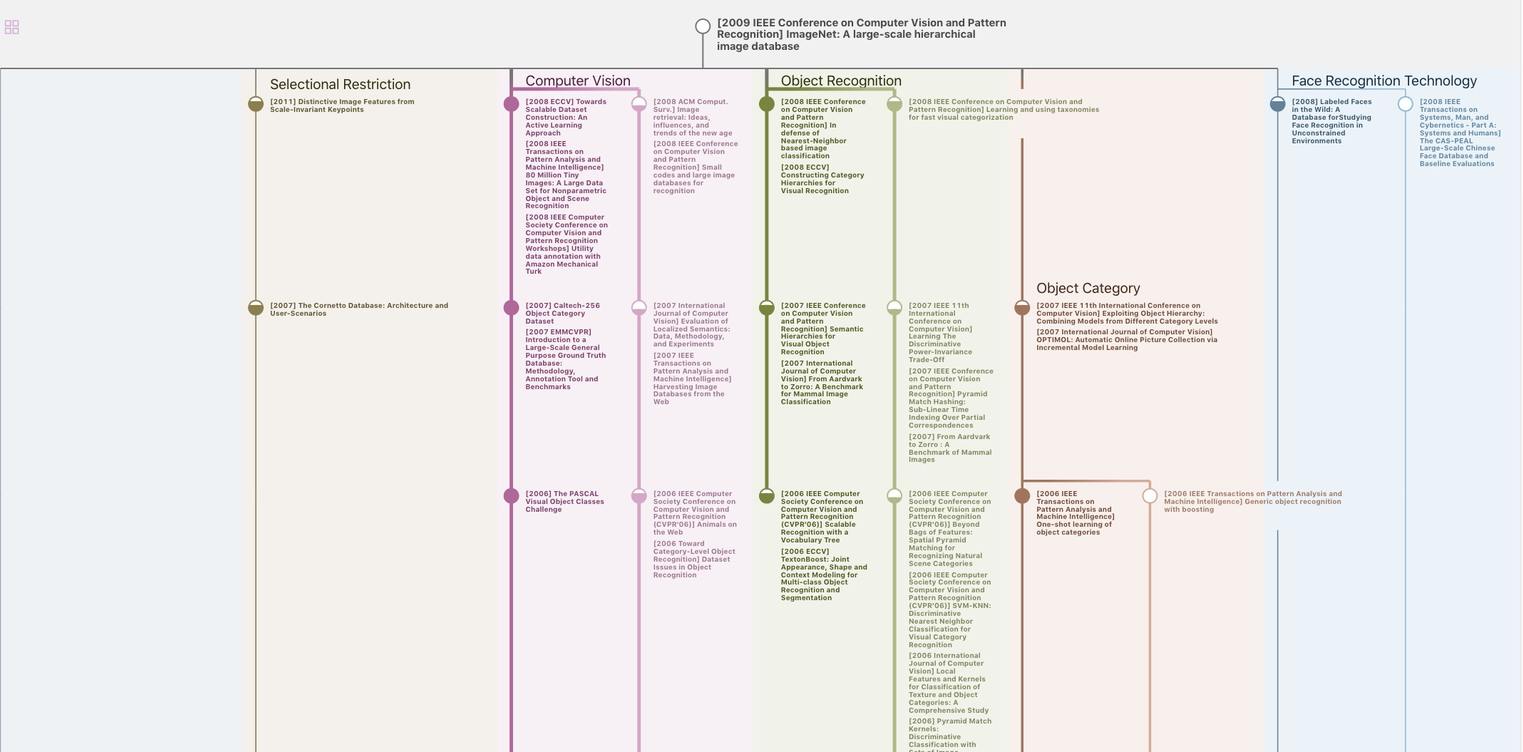
生成溯源树,研究论文发展脉络
Chat Paper
正在生成论文摘要