Navigating Mobile Robots To Target In Near Shortest Time Using Reinforcement Learning With Spiking Neural Networks
2017 INTERNATIONAL JOINT CONFERENCE ON NEURAL NETWORKS (IJCNN)(2017)
摘要
The autonomous navigation of mobile robots in unknown environments is of great interest in mobile robotics. This article discusses a new strategy to navigate to a known target location in an unknown environment using a combination of the "go-to-goal" approach and reinforcement learning with biologically realistic spiking neural networks. While the "go-to-goal" approach itself might lead to a solution for most environments, the added neural reinforcement learning in this work results in a strategy that takes the robot from a starting position to a target location in a near shortest possible time. To achieve the goal, we propose a reinforcement learning approach based on spiking neural networks. The presented biologically motivated delayed reward mechanism using eligibility traces results in a greedy approach that leads the robot to the target in a close to shortest possible time.
更多查看译文
关键词
autonomous mobile robots navigation,go-to-goal approach,biologically realistic spiking neural networks,neural reinforcement learning,near shortest possible time,biologically motivated delayed reward mechanism,eligibility traces,greedy approach
AI 理解论文
溯源树
样例
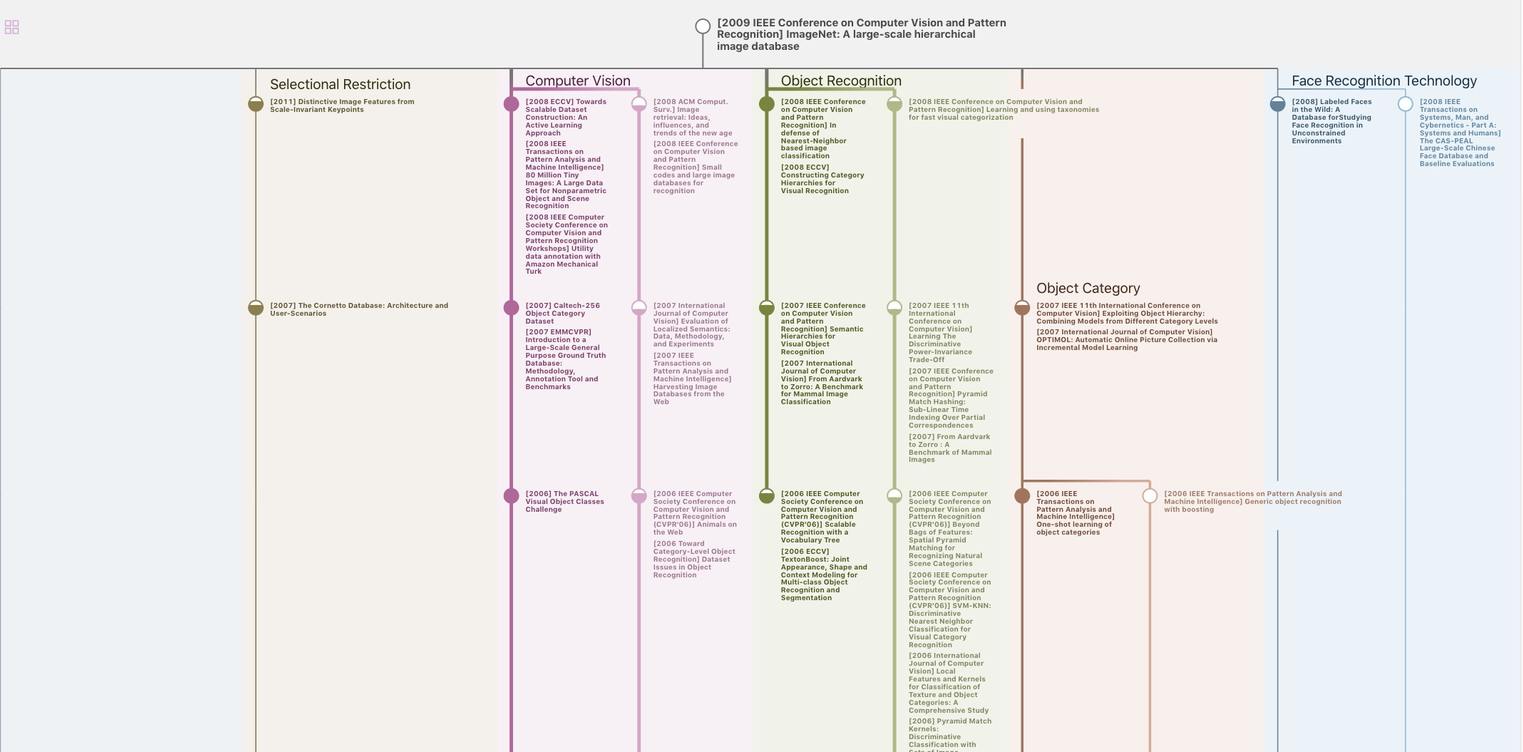
生成溯源树,研究论文发展脉络
Chat Paper
正在生成论文摘要