Image Segmentation With Stochastic Magnetic Tunnel Junctions And Spiking Neurons
2017 INTERNATIONAL JOINT CONFERENCE ON NEURAL NETWORKS (IJCNN)(2017)
摘要
Image segmentation is a crucial pre-processing stage used in many object identification problems. The purpose of image segmentation is to simplify the representation of an image such that it can be more conveniently analyzed in the later stages of a problem. This is generally achieved through partitioning a complicated image into specific groups based on color, intensity or texture of the pixels of that image. Locally Excitatory Globally Inhibitory Oscillator Network or LEGION is one such segmentation algorithm, where synchronization and desynchronization between coupled oscillators are used to segment an image. To extract maximum benefits from the fast parallel processing nature of LEGION, one must resort to a hardware implementation of this architecture. Unfortunately, the present structure of LEGION with relaxation oscillators as nodes, is not ideal for scalable and energy efficient hardware realization of the network. In this work we propose two different networks for image segmentation, one with leaky integrate and fire neurons and the other with stochastic Magnetic Tunneling Junctions (MTJs), both inspired by the operating principles of LEGION. The structure of the proposed networks allows them to be translated into energy efficient and scalable hardware platforms. We demonstrate that the proposed networks can effectively and efficiently segment binary and gray-scale images with multiple objects.
更多查看译文
关键词
image segmentation,stochastic magnetic tunnel junctions,spiking neurons,object identification problems,image representation,image partitioning,pixel color,pixel intensity,pixel texture,locally excitatory globally inhibitory oscillator network,LEGION,segmentation algorithm,MTJ,leaky integrate and fire neurons,binary image,gray-scale image
AI 理解论文
溯源树
样例
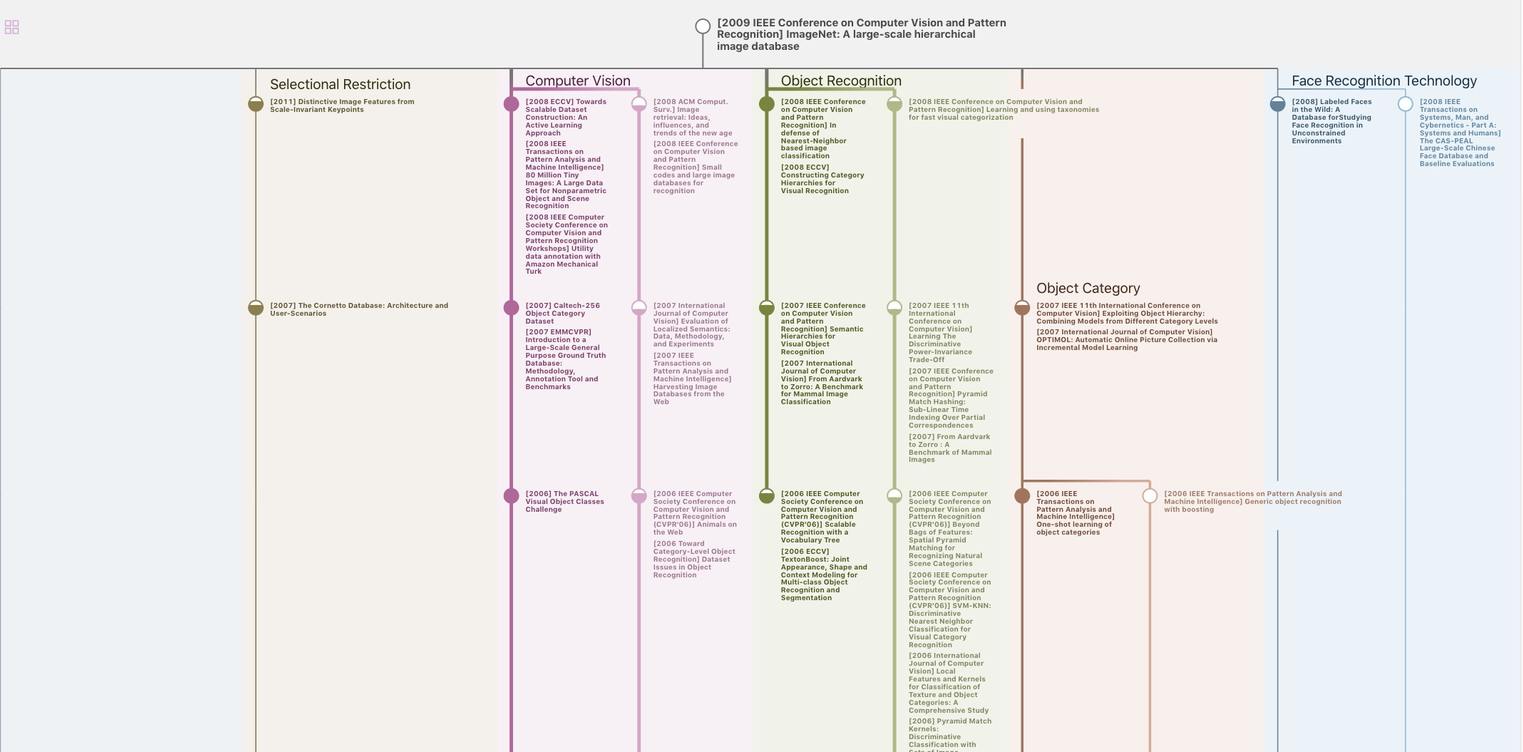
生成溯源树,研究论文发展脉络
Chat Paper
正在生成论文摘要