Temporal Hierarchies In Multilayer Gated Recurrent Neural Networks For Language Models
2017 INTERNATIONAL JOINT CONFERENCE ON NEURAL NETWORKS (IJCNN)(2017)
摘要
Representing multiple compositions of human language has been a difficult task due to the complex hierarchical and compositional nature of language. Hierarchical structures are one of the architectures which can be used to capture such compositionalities. In this paper, we introduce temporal hierarchies to the Neural Language Model (NLM) with the help of a Deep Gated Recurrent Neural Network with adaptive timescales to help represent multiple compositions of language. We demonstrate that by representing multiple compositions of language in a deep recurrent neural network architecture, we can improve the performance of Language Models without complex hierarchical architectures. We report the performance of the proposed model using the popular Penn Treebank (PTB) dataset. The results show that by using the multiple timescale concept in an NLM, we can achieve better perplexities compared to the existing baselines.
更多查看译文
关键词
temporal hierarchies,multilayer gated recurrent neural networks,human language,hierarchical structures,neural language model,NLM,deep gated recurrent neural network,adaptive timescales,deep recurrent neural network architecture,performance improvement,Penn Treebank dataset,PTB dataset
AI 理解论文
溯源树
样例
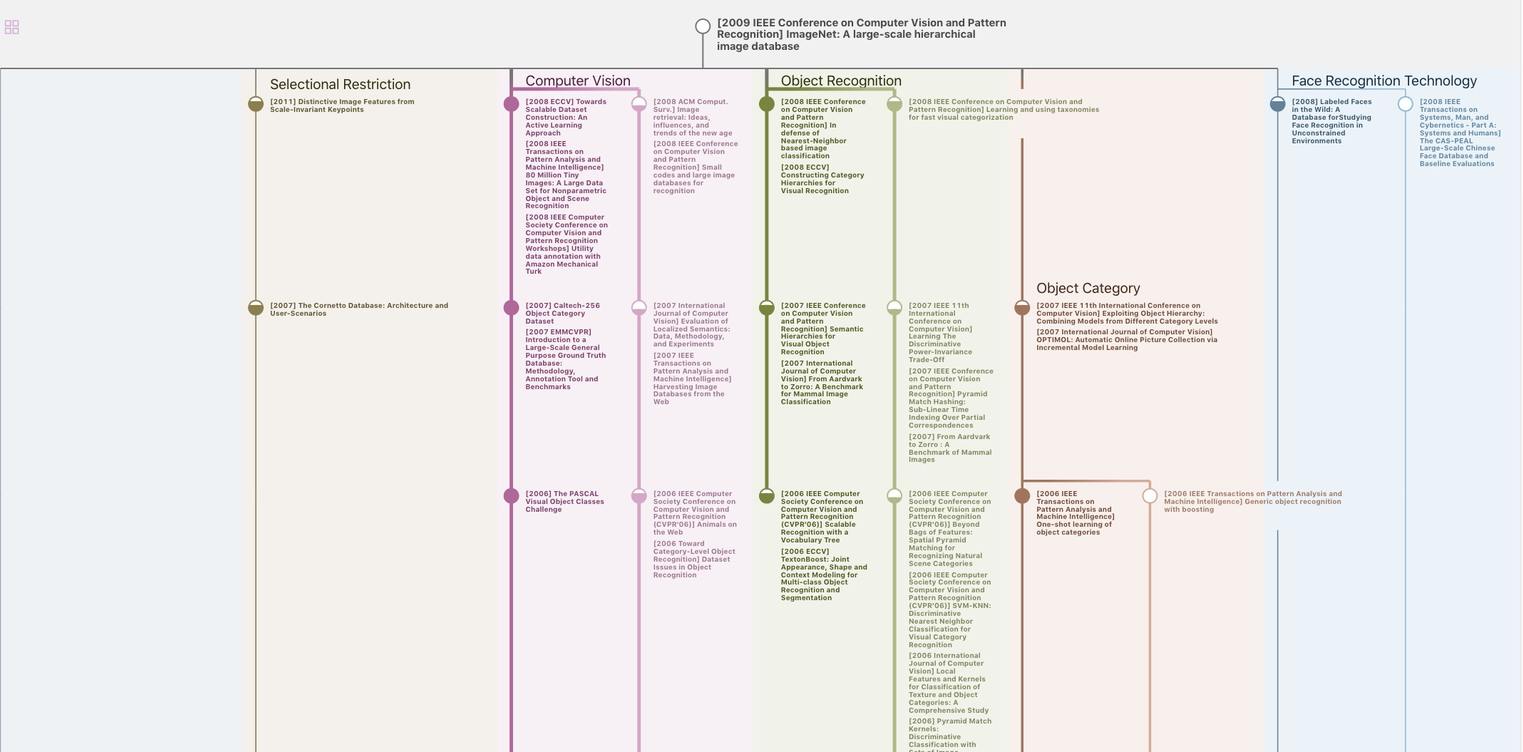
生成溯源树,研究论文发展脉络
Chat Paper
正在生成论文摘要