Groupwise Bayesian Dimension Reduction
2017 INTERNATIONAL JOINT CONFERENCE ON NEURAL NETWORKS (IJCNN)(2017)
摘要
Nearly all existing estimations of the central subspace in regression take the frequentist approach. However, when the predictors fall naturally into a number of groups, these frequentist methods treat all predictors indiscriminately and can result in loss of the group-specific relation between the response and the predictors. In this article, we propose a Bayesian solution for dimension reduction which incorporates such group knowledge. We place a prior whose variance is constrained to the form of a direct sum on the central subspace and directly model the response density in terms of the sufficient predictors using a finite mixture model. This approach is computationally efficient and offers a unified framework to handle categorical predictors, missing predictors, and Bayesian variable selection. We illustrate the method using both a simulation study and an analysis of a temperature data set.
更多查看译文
关键词
groupwise Bayesian dimension reduction,frequentist approach,group-specific relation,group knowledge,variance,central subspace,finite mixture model,categorical predictors,missing predictors,Bayesian variable selection,temperature data set analysis
AI 理解论文
溯源树
样例
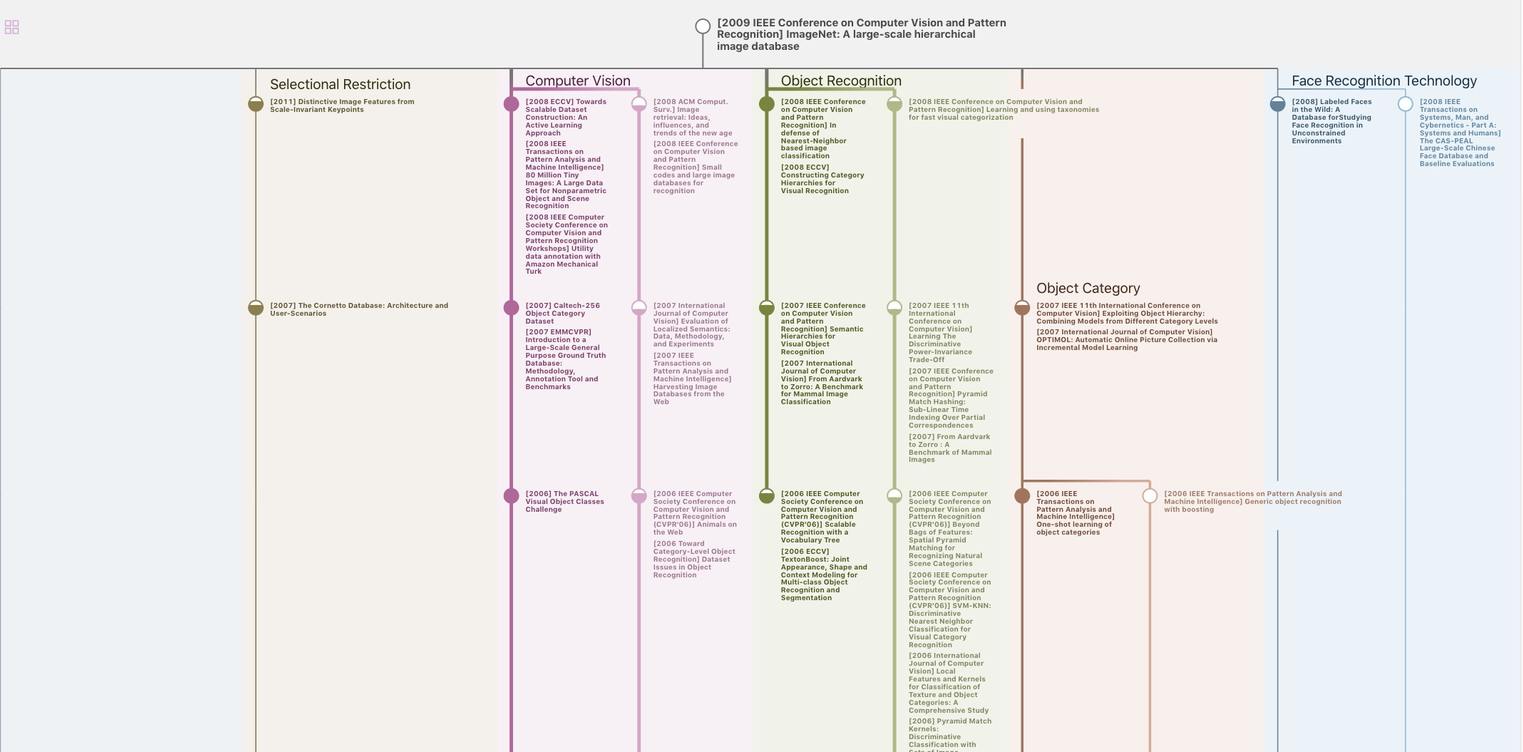
生成溯源树,研究论文发展脉络
Chat Paper
正在生成论文摘要