Cluster-Than-Label: Semi-Supervised Approach For Domain Adaptation
2017 IEEE 31ST INTERNATIONAL CONFERENCE ON ADVANCED INFORMATION NETWORKING AND APPLICATIONS (AINA)(2017)
摘要
The performance of a conventional machine learning model trained on a source domain degrades poorly when they are tested on a different data distribution (target domain). These traditional models deal with this problem by training a new paradigm for the particular different data distribution (target domain). Therefore, training of a new paradigm for the individual data distribution is computationally expensive. This paper demonstrates that how to adapt to a new data distribution (target domain), utilising the model trained on source domain and avoiding the cost of re-training and the need for access to the source labelled data. In particular, we introduce an Efficient Semi-supervised Cluster-than-Label Cross-domain Adaptation Algorithm (SCTLCDA) to address the cross-domain adaptation classification problem in which we utilised both labelled and unlabelled data samples in the target domain, as well as completely unlabelled data samples in the source domain. Subsequently, we also describe that our proposed method can manage large datasets and easily lead to cross-domain adaptation problem. The effectiveness and performance of our method are confirmed by experiments on two real-world applications: Cross-domain sentiments and Web-Spam classification problem.
更多查看译文
关键词
Cross domian adaptation,clutersing,web spam classification
AI 理解论文
溯源树
样例
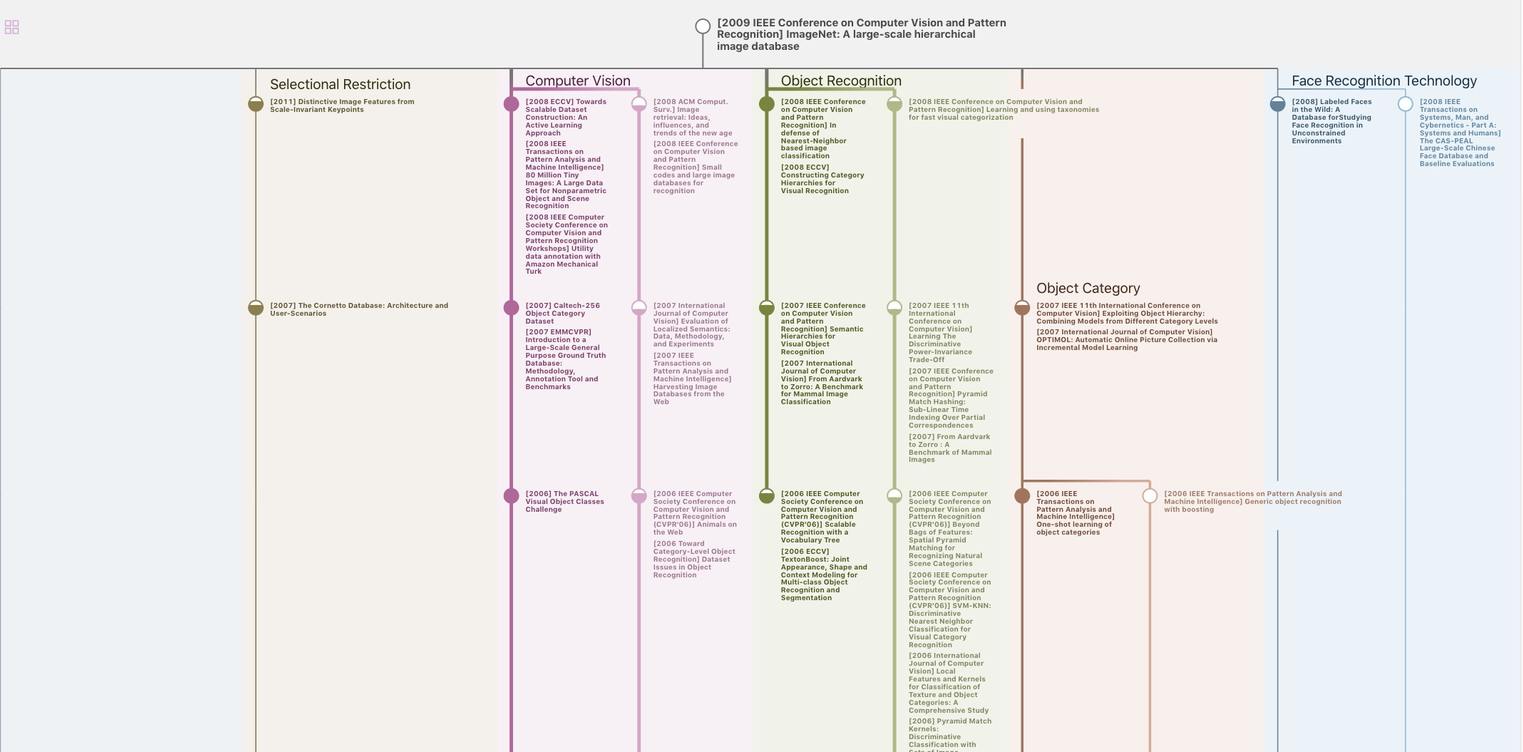
生成溯源树,研究论文发展脉络
Chat Paper
正在生成论文摘要