Saliency Detection Optimization via Modified Secondary Manifold Ranking and Blurring Depression.
ADVANCES IN NEURAL NETWORKS, PT II(2017)
摘要
We propose an unsupervised saliency optimization method mainly via modified secondary manifold ranking and blurring depression (SMBD). Generally, saliency object is detected insufficiently by most methods. To solve this problem, a modified manifold ranking is circulated twice to detect saliency object completely. A blurry degree detection approach is introduced to locate blurring regions, which is more likely to be background. As a result, blurring regions are depressed by SMBD to avoid mistaking background as foreground. Our method is performed based on hierarchical luminance for better performance. Extensive experimental results demonstrate that SMBD is able to promote the performances of state-of-the-art saliency detection algorithms significantly.
更多查看译文
关键词
Sliency detection,Manifold ranking,Blurring depression
AI 理解论文
溯源树
样例
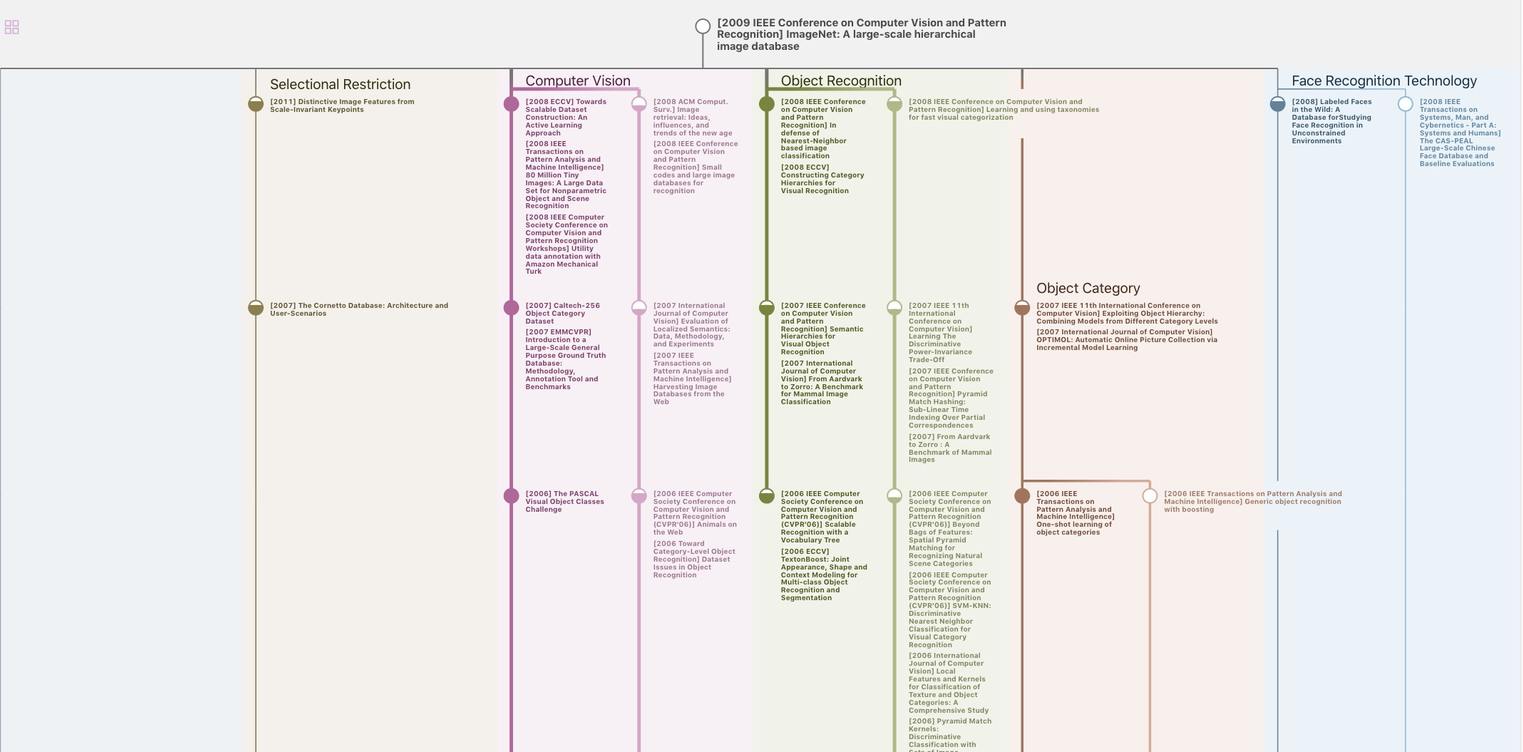
生成溯源树,研究论文发展脉络
Chat Paper
正在生成论文摘要