Reporting Statistical Validity and Model Complexity in Machine Learning based Computational Studies
EASE(2017)
摘要
Background:: Statistical validity and model complexity are both important concepts to enhanced understanding and correctness assessment of computational models. However, information about these are often missing from publications applying machine learning. Aim: The aim of this study is to show the importance of providing details that can indicate statistical validity and complexity of models in publications. This is explored in the context of citation screening automation using machine learning techniques. Method: We built 15 Support Vector Machine (SVM) models, each developed using word2vec (average word) features --- and data for 15 review topics from the Drug Evaluation Review Program (DERP) of the Agency for Healthcare Research and Quality (AHRQ). Results: The word2vec features were found to be sufficiently linearly separable by the SVM and consequently we used the linear kernels. In 11 of the 15 models, the negative (majority) class used over 80% of its training data as support vectors (SVs) and approximately 45% of the positive training data. Conclusions: In this context, exploring the SVs revealed that the models are overly complex against ideal expectations of not more than 2%-5% (and preferably much less) of the training vectors.
更多查看译文
AI 理解论文
溯源树
样例
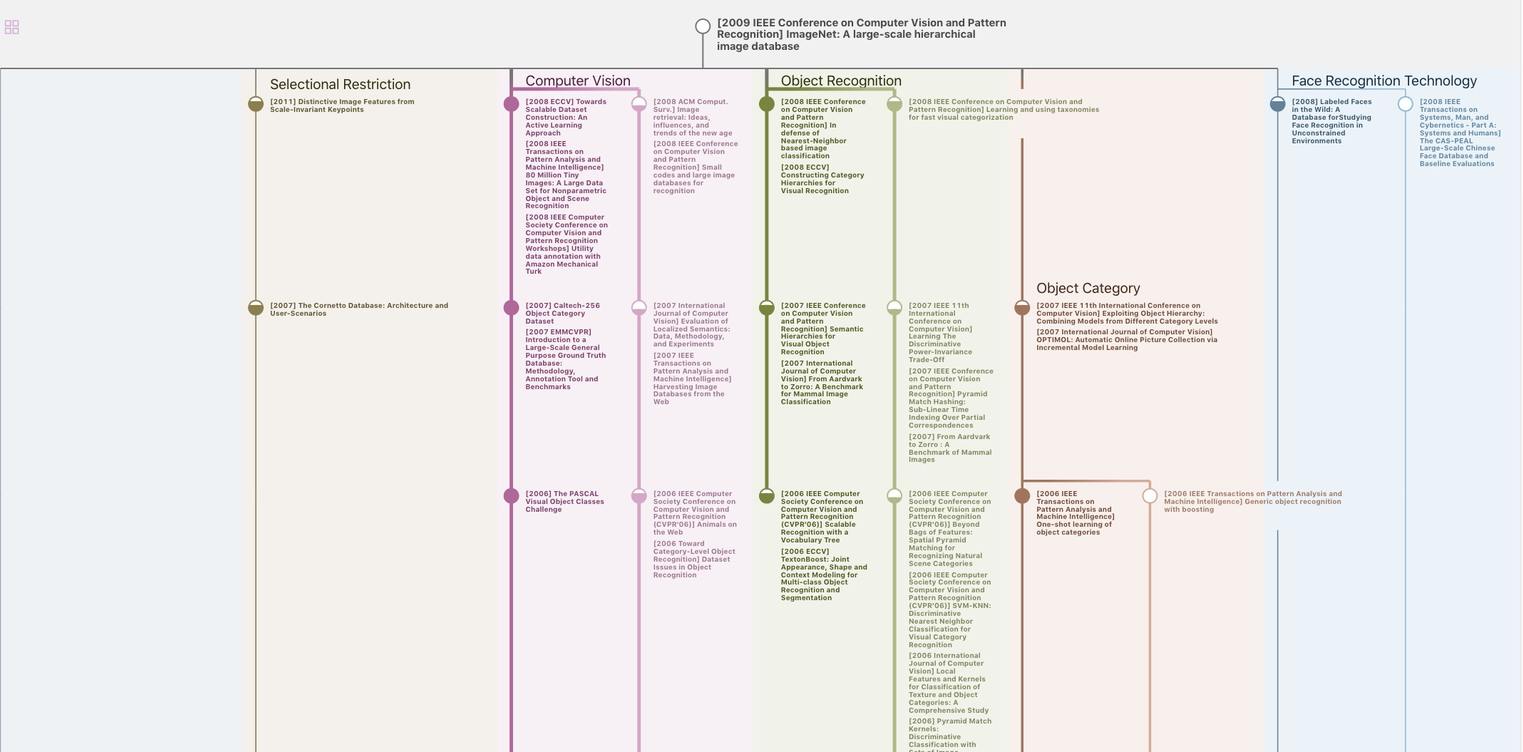
生成溯源树,研究论文发展脉络
Chat Paper
正在生成论文摘要