Speeding up evolutionary multi-objective optimisation through diversity-based parent selection.
GECCO(2017)
摘要
Parent selection in evolutionary algorithms for multi-objective optimization is usually performed by dominance mechanisms or indicator functions that prefer non-dominated points, while the reproduction phase involves the application of diversity mechanisms or other methods to achieve a good spread of the population along the Pareto front. We propose to refine the parent selection on evolutionary multi-objective optimization with diversity-based metrics. The aim is to focus on individuals with a high diversity contribution located in poorly explored areas of the search space, so the chances of creating new non-dominated individuals are better than in highly populated areas. We show by means of rigorous runtime analysis that the use of diversity-based parent selection mechanisms in the Simple Evolutionary Multi-objective Optimiser (SEMO) and Global SEMO for the well known bi-objective functions OneMinMax and Lotz can significantly improve their performance. Our theoretical results are accompanied by additional experiments that show a correspondence between theory and empirical results.
更多查看译文
关键词
Parent selection, evolutionary algorithms, multi-objective optimization, diversity mechanisms, runtime analysis, theory
AI 理解论文
溯源树
样例
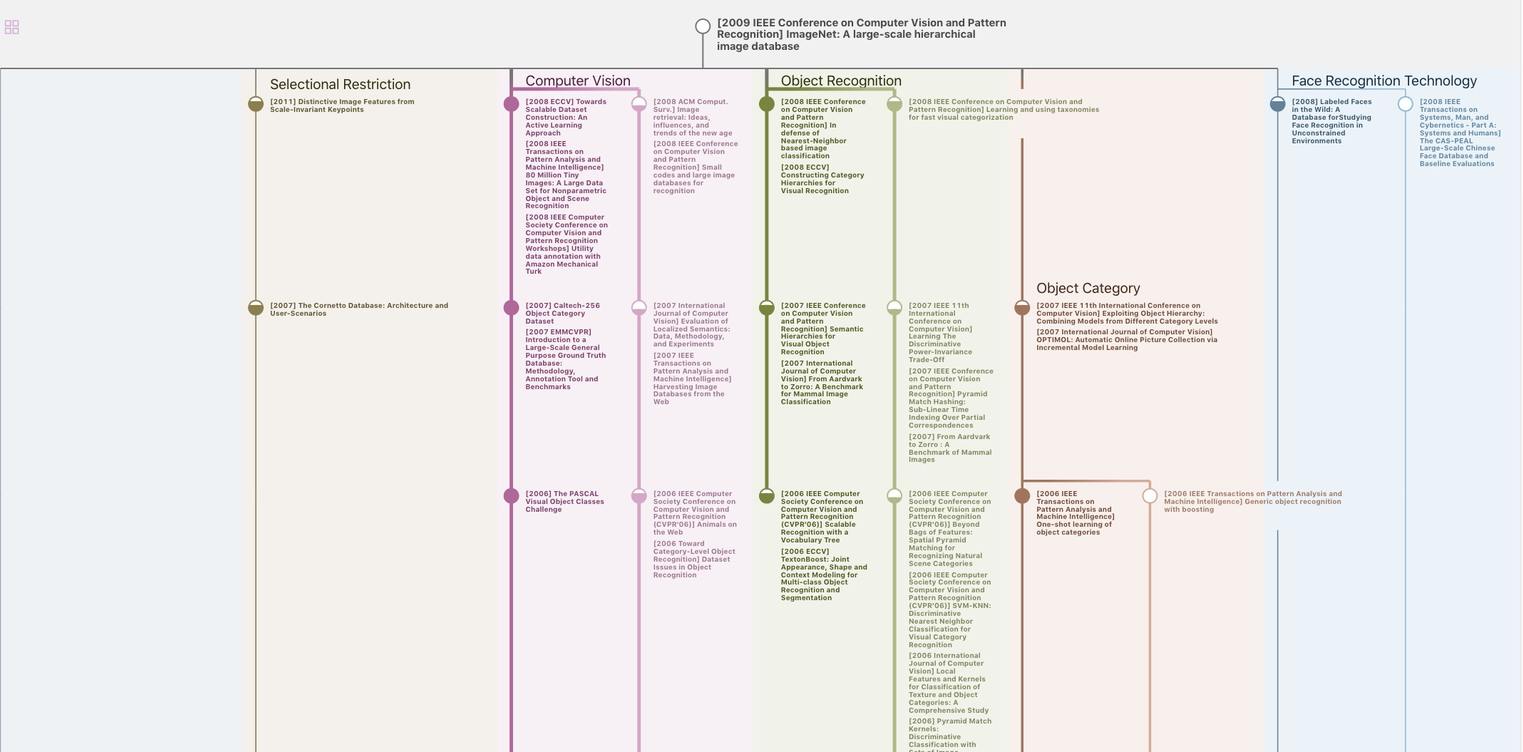
生成溯源树,研究论文发展脉络
Chat Paper
正在生成论文摘要