On Asymptotic Cost of Triangle Listing in Random Graphs.
PODS(2017)
摘要
Triangle listing has been a long-standing problem, with many heuristics, bounds, and experimental results, but not much asymptotically accurate complexity analysis. To address this issue, we introduce a novel stochastic framework, based on Glivenko-Cantelli results for functions of order statistics, that allows modeling cost of in-memory triangle enumeration in families of random graphs. Unlike prior work that usually studies the O(.) notation, we derive the exact limits of CPU complexity of all vertex/edge iterators under arbitrary acyclic orientations as graph size n → ∞. These results are obtained in simple closed form as functions of the degree distribution. This allows us to establish optimal orientations for all studied algorithms, compare them to each other, and discover the best technique within each class.
更多查看译文
AI 理解论文
溯源树
样例
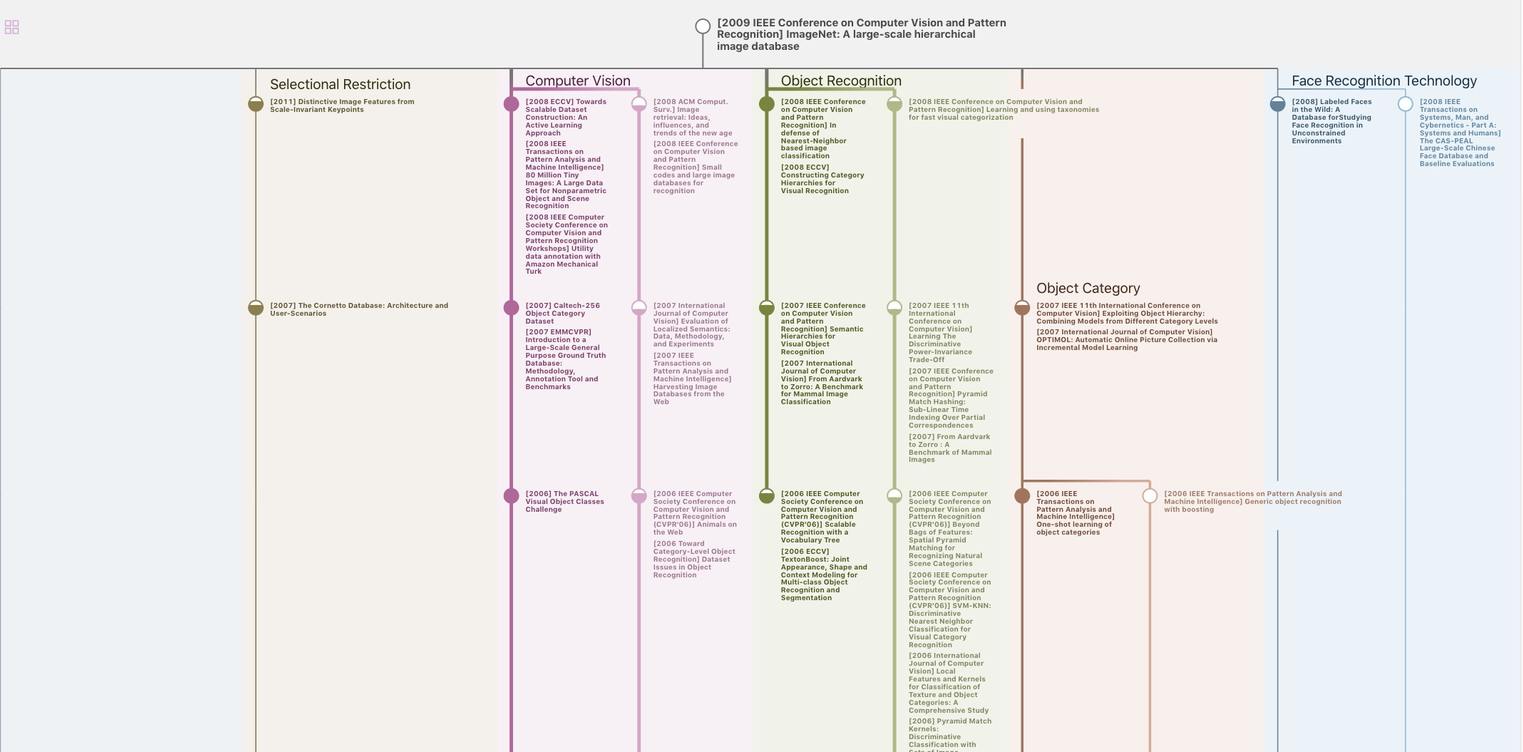
生成溯源树,研究论文发展脉络
Chat Paper
正在生成论文摘要