Query Suggestion to allow Intuitive Interactive Search in Multidimensional Time Series.
SSDBM(2017)
摘要
In recent years, the research community, inspired by its success in dealing with single-dimensional time series, has turned its attention to dealing with multidimensional time series. There are now a plethora of techniques for indexing, classification, and clustering of multidimensional time series. However, we argue that the difficulty of exploratory search in large multidimensional time series remains underappreciated. In essence, the problem reduces to the \"chicken-and-egg\" paradox that it is difficult to produce a meaningful query without knowing the best subset of dimensions to use, but finding the best subset of dimensions is itself query dependent. In this work we propose a solution to this problem. We introduce an algorithm that runs in the background, observing the user's search interactions. When appropriate, our algorithm suggests to the user a dimension that could be added or deleted to improve the user's satisfaction with the query. These query dependent suggestions may be useful to the user, even if she does not act on them (by reissuing the query), as they can hint at unexpected relationships or redundancies between the dimensions of the data. We evaluate our algorithm on several real-world datasets in medical, human activity, and industrial domains, showing that it produces subjectively sensible and objectively superior results.
更多查看译文
关键词
Query Suggestion,Multidimensional Time Series
AI 理解论文
溯源树
样例
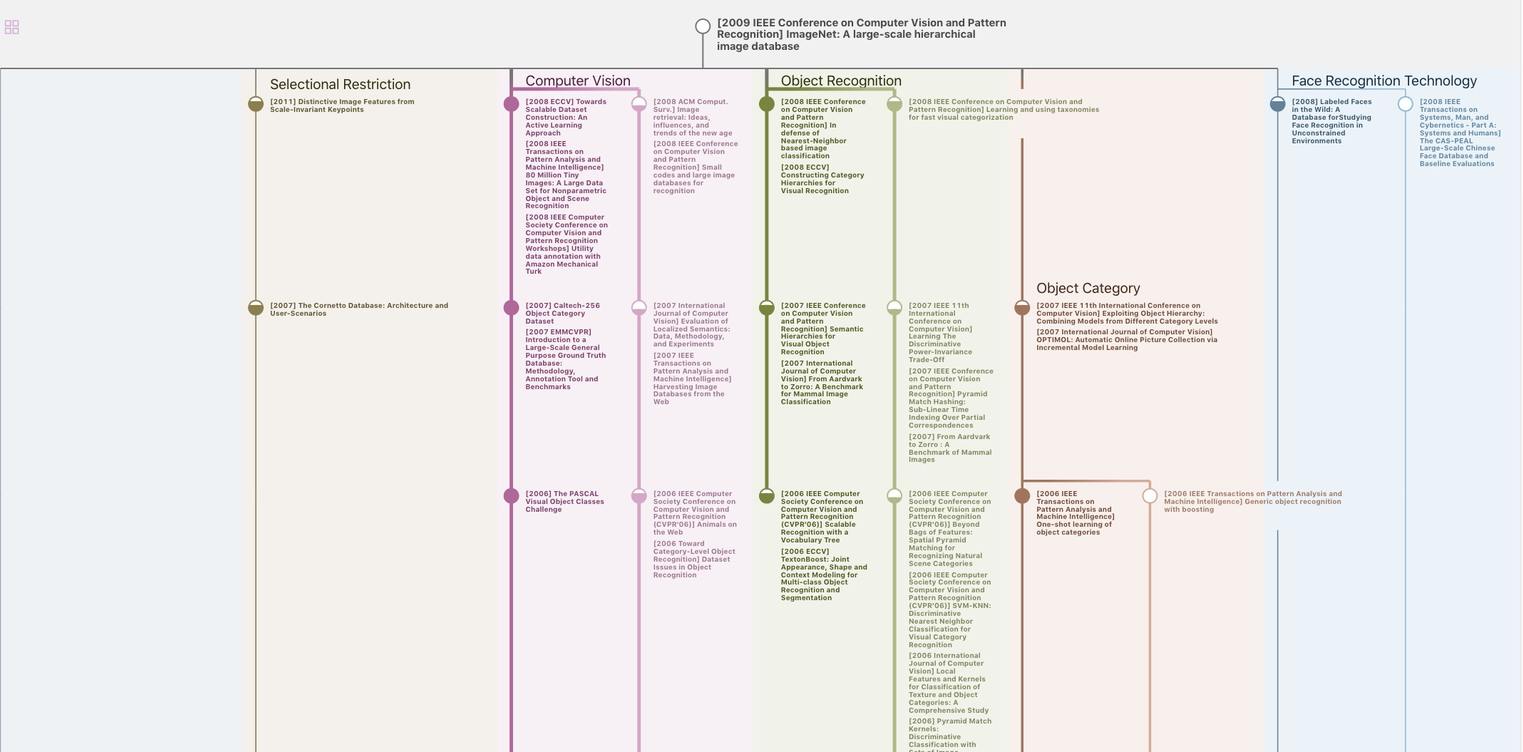
生成溯源树,研究论文发展脉络
Chat Paper
正在生成论文摘要