Similarity Search And Dimensionality Reduction: Not All Dimensions Are Equally Useful
DATABASE SYSTEMS FOR ADVANCED APPLICATIONS(2004)
摘要
Indexing high-dimensional data is a well known,problem. Techniques for dimensionality reduction which map D-dimensional objects onto a d-dimensional space (d much less than D) are often used to speedup similarity queries. In this paper we show that one can further improve query performance by initially overestimating the reduction, i.e., reducing the dimensionality of the space to D' dimensions, where d < D' < D, and, at query time, automatically choosing only d', where d' < d, dimensions to be used - that is, using only a few good dimensions after the initial reduction of the dimensionality. By incorporating this idea within a recently proposed technique, we can process range queries up to three times faster at the expense of limited storage overhead.
更多查看译文
关键词
high dimensional data,similarity search,indexation
AI 理解论文
溯源树
样例
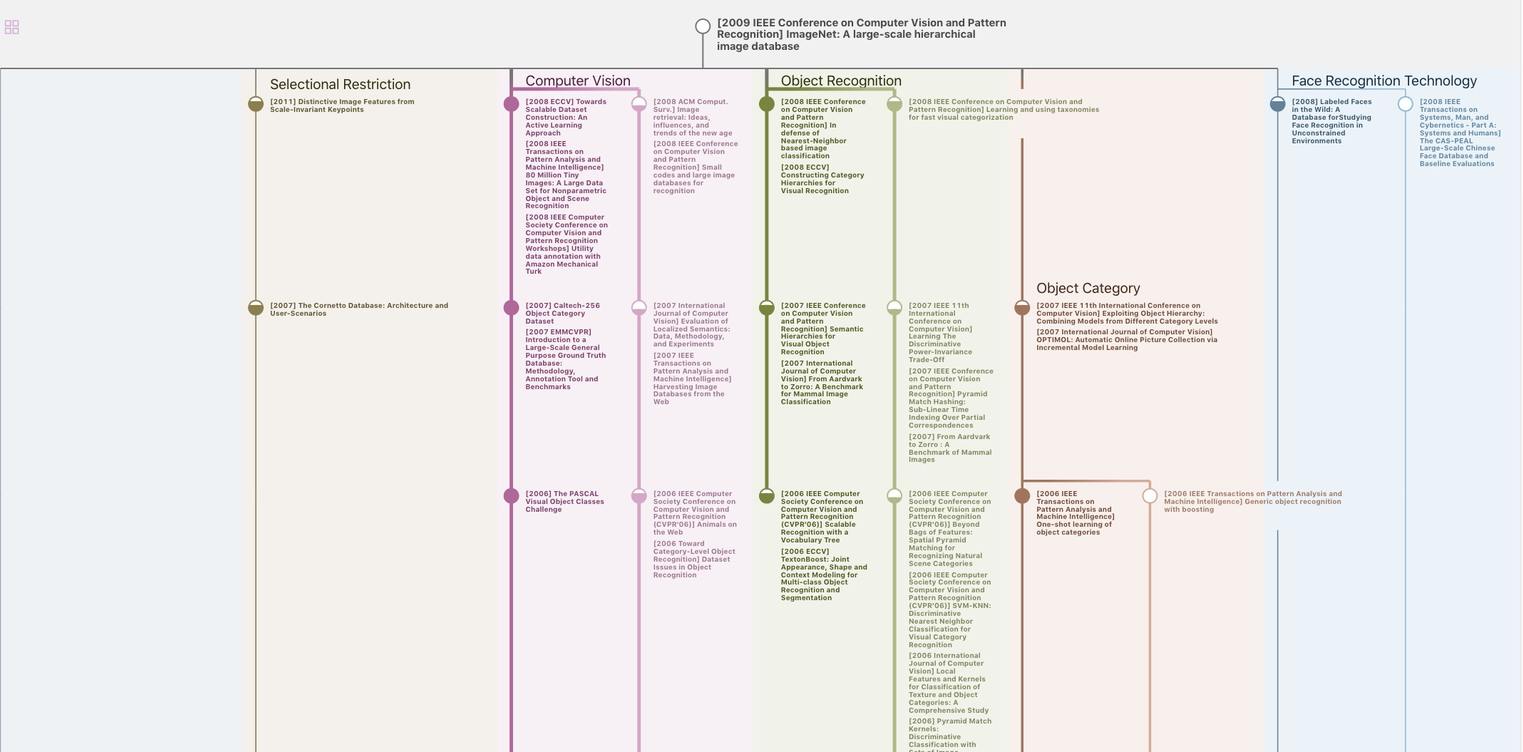
生成溯源树,研究论文发展脉络
Chat Paper
正在生成论文摘要