Anchored Kernel Metric Learning
2016 IEEE INTERNATIONAL CONFERENCE ON IMAGE PROCESSING (ICIP)(2016)
摘要
This paper proposes the Anchored Kernel Metric Learning (AKML) method, which learns metrics by sparsely combining locally discriminative rank-1 basis matrices in the anchored kernel induced space. First, we apply k-means clustering to generate anchored samples, thus forming an anchored sample matrix. Based on the assumption that the basis matrices can be formulated as linear combinations of anchored samples, we then learn the combining weights by regularized LDA in anchored samples' neighborhoods. Finally, a logistic loss with nonnegative constraints is utilized to select and weigh these basis matrices for a PSD metric matrix. Under this framework, we incorporate multiple kernels into AKML for further boosting the performance. Experiments on two benchmark person re-identification datasets demonstrate that our approach clearly outperforms the state-of-the-art metric learning methods.
更多查看译文
关键词
Metric learning,anchored kernel,regularized LDA,person re-identification
AI 理解论文
溯源树
样例
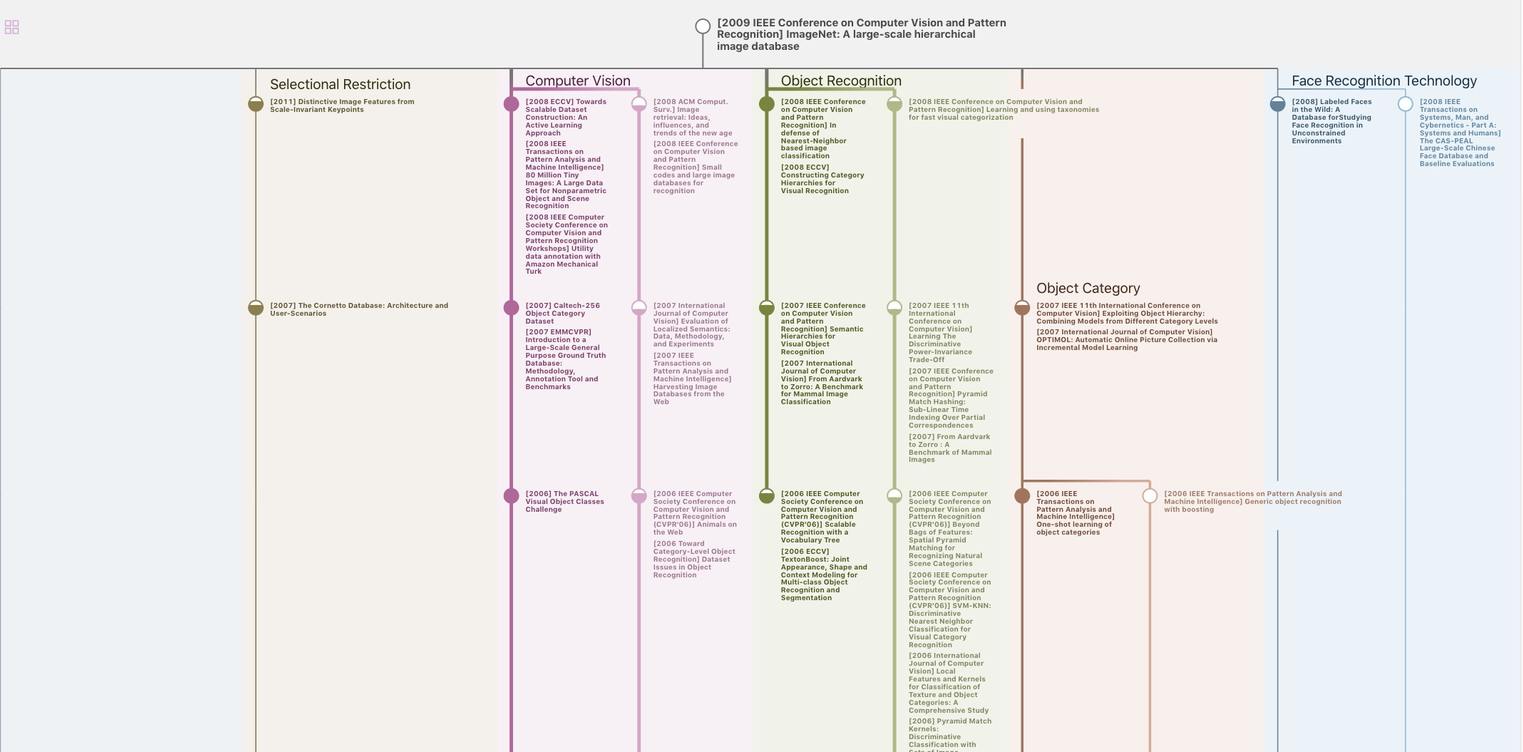
生成溯源树,研究论文发展脉络
Chat Paper
正在生成论文摘要