Density-based multiscale analysis for clustering in strong noise settings
Lecture Notes in Computer Science (including subseries Lecture Notes in Artificial Intelligence and Lecture Notes in Bioinformatics)(2017)
摘要
Finding clustering patterns in data is challenging when clusters can be of arbitrary shapes and the data contains high percentage (e.g., 80%) of noise. This paper presents a novel technique named density-based multiscale analysis for clustering (DBMAC) that can conduct noise-robust clustering without any strict assumption on the shapes of clusters. Firstly, DBMAC calculates the r-neighborhood statistics with different r (radius) values. Next, instead of trying to find a single optimal r value, a set of radius values appropriate for separating “clustered” objects and “noisy” objects is identified, using a formal statistical method for multimodality test. Finally, the classical DBSCAN is employed to perform clustering on the subset of data with significantly less amount of noise. Experiment results confirm that DBMAC is superior to classical DBSCAN in strong noise settings and also outperforms the latest technique SkinnyDip when the data contains arbitrarily shaped clusters. © Springer International Publishing AG 2017.
更多查看译文
关键词
Density-based clustering,Multiscale analysis,Statistical test
AI 理解论文
溯源树
样例
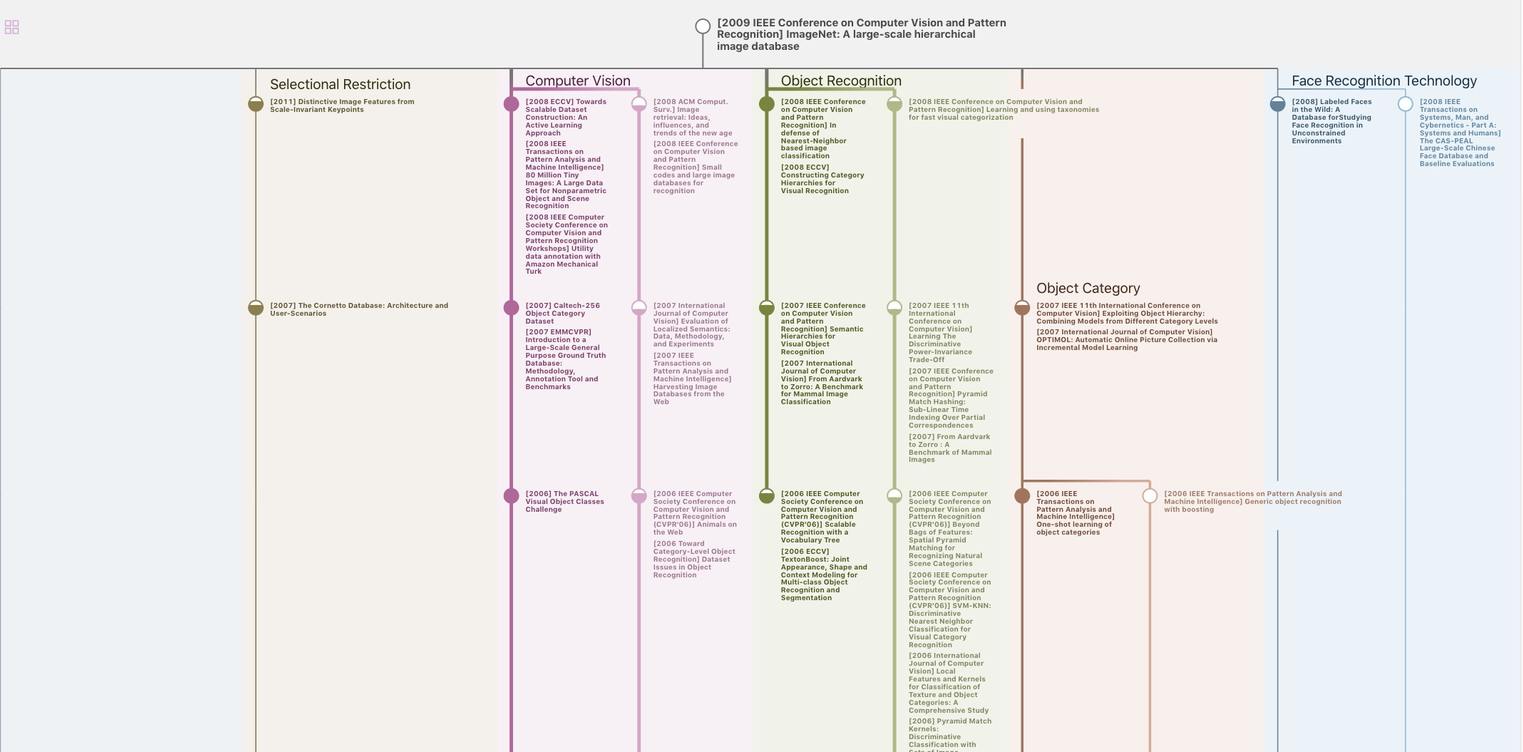
生成溯源树,研究论文发展脉络
Chat Paper
正在生成论文摘要