Adaptive Hierarchical Classification Networks
2016 23RD INTERNATIONAL CONFERENCE ON PATTERN RECOGNITION (ICPR)(2016)
摘要
Hierarchical decomposition enables increased number of classes in a classification problem. Class similarities guide the creation of a family of course to fine classifiers which solve categorical problems more effectively than a single flat classifier. High accuracies require precise configurations for each of the family of classifiers. This paper proposes a method to adaptively select the configuration of the hierarchical family of classifiers. Linkage statistics from overall and sub-classification confusion matrices define categorical groupings for an efficient and accurate classification framework. Depending on the number of classes and the complexity of the problem, an adaptive configuration manager chooses between a multi-layer perceptron or a deep convolutional neural network, then selects the complexity of each. Experiments on CalTech101, CalTech256, CIFAR100 and the ImageNet datasets demonstrate performance of adaptive hierarchical models on an image classification task.
更多查看译文
关键词
Hierarchy,Decomposition,Image Classification,Muli-layer Perceptron,Convolutional Neural Network
AI 理解论文
溯源树
样例
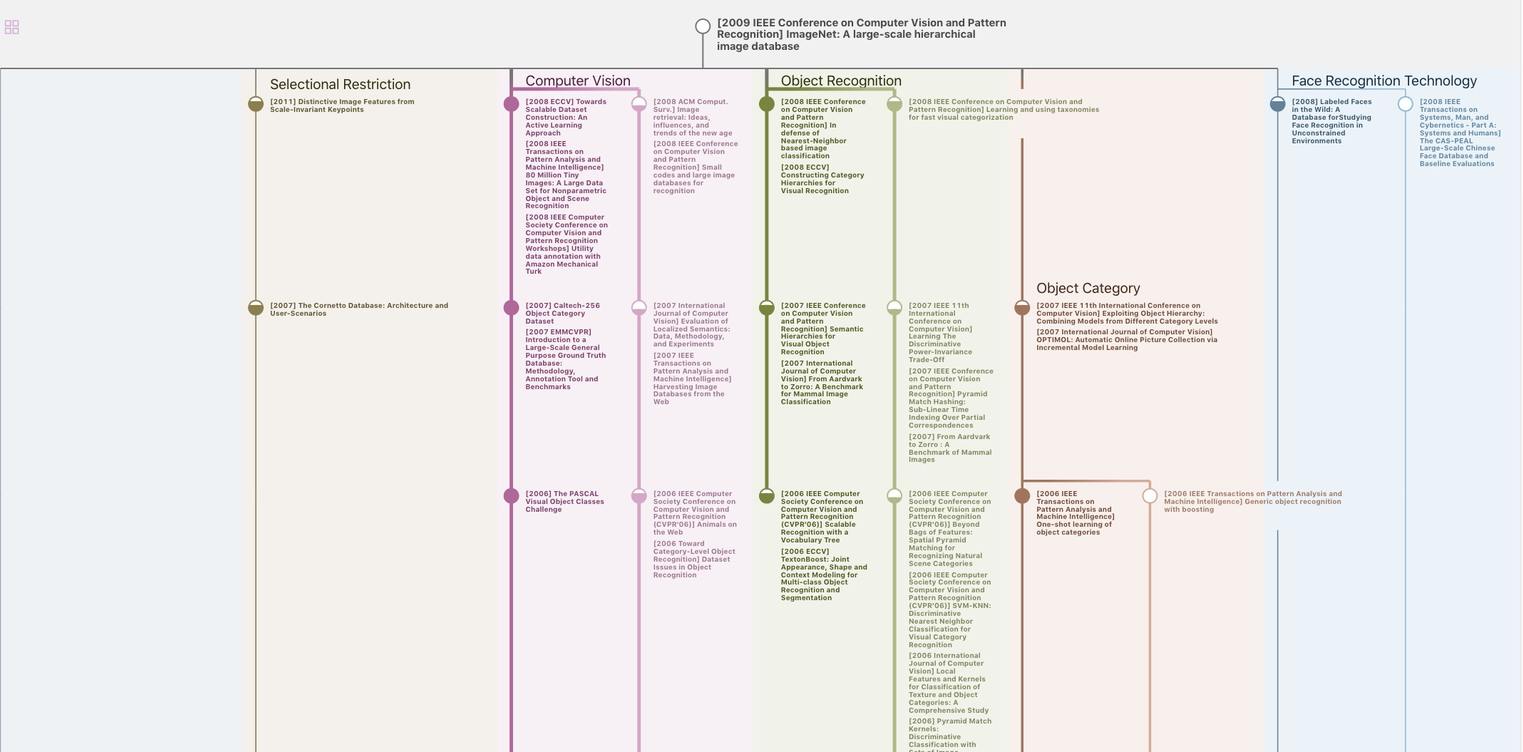
生成溯源树,研究论文发展脉络
Chat Paper
正在生成论文摘要