Hyperparameter Tuning For Big Data Using Bayesian Optimisation
2016 23RD INTERNATIONAL CONFERENCE ON PATTERN RECOGNITION (ICPR)(2016)
摘要
Hyperparameters play a crucial role in the model selection of machine learning algorithms. Tuning these hyperparameters can be exhaustive when the data is large. Bayesian optimisation has emerged as an efficient tool for hyperparameter tuning of machine learning algorithms. In this paper, we propose a novel framework for tuning the hyperparameters for big data using Bayesian optimisation. We divide the big data into chunks and generate hyperparameter configurations for the chunks using the standard Bayesian optimisation. We utilise this information from the chunks for hyperparameter tuning on big data using a transfer learning setting. We evaluate the performance of the proposed method on the task of tuning hyperparameters of two machine learning algorithms. We show that our method achieves the best available hyperparameter configuration within less computational time compared to the state-of-art hyperparameter tuning methods.
更多查看译文
关键词
hyperparameter tuning,Big Data,Bayesian optimisation,model selection,machine learning algorithms,transfer learning,hyperparameter configuration
AI 理解论文
溯源树
样例
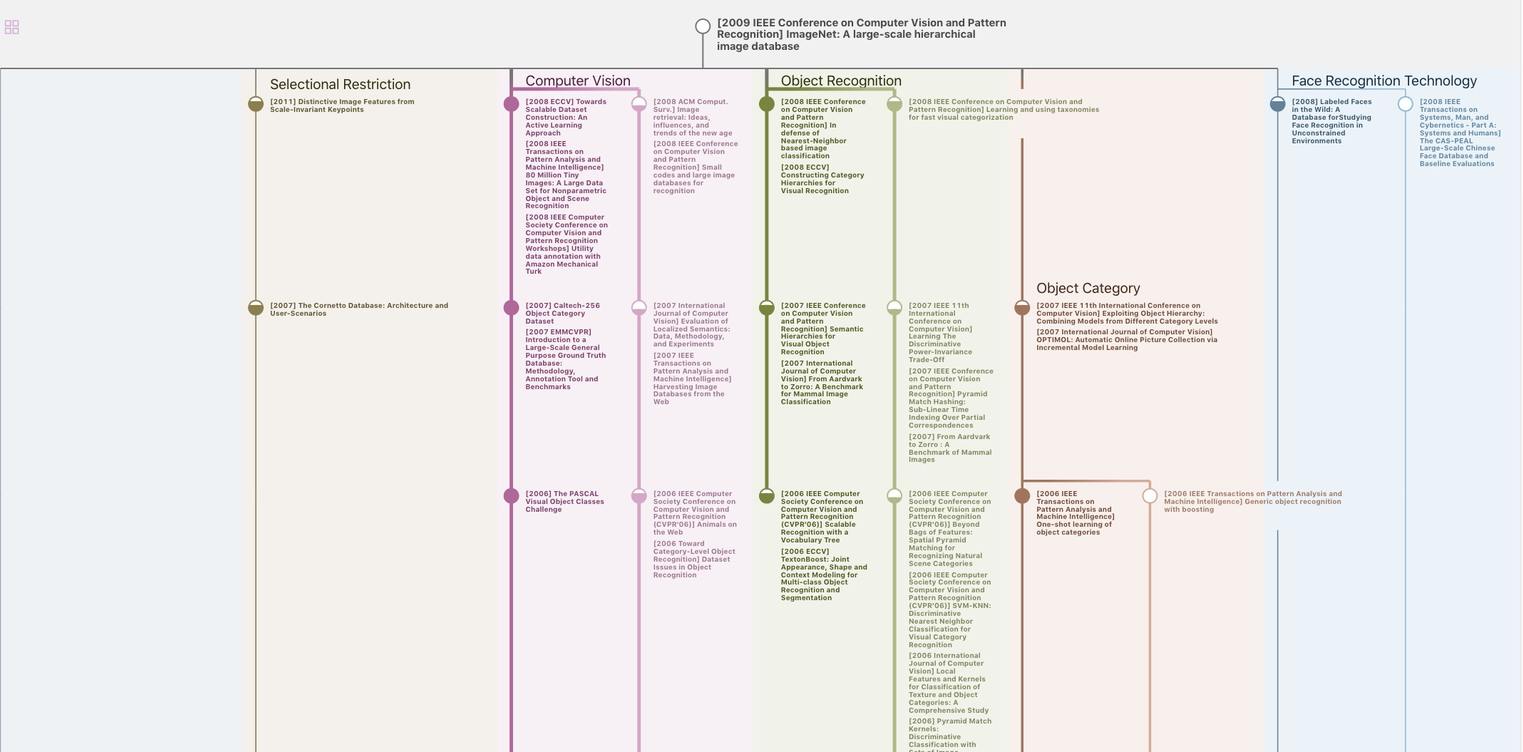
生成溯源树,研究论文发展脉络
Chat Paper
正在生成论文摘要