Training Robust Models Using Random Projection
2016 23RD INTERNATIONAL CONFERENCE ON PATTERN RECOGNITION (ICPR)(2016)
摘要
Regularization plays an important role in machine learning systems. We propose a novel methodology for model regularization using random projection. We demonstrate the technique on neural networks, since such models usually comprise a very large number of parameters, calling for strong regularizers. It has been shown recently that neural networks are sensitive to two kinds of samples: (i) adversarial samples, which are generated by imperceptible perturbations of previously correctly-classified samples-yet the network will misclassify them; and (ii) fooling samples, which are completely unrecognizable, yet the network will classify them with extremely high confidence. In this paper, we show how robust neural networks can be trained using random projection. We show that while random projection acts as a strong regularizer, boosting model accuracy similar to other regularizers, such as weight decay and dropout, it is far more robust to adversarial noise and fooling samples. We further show that random projection also helps to improve the robustness of traditional classifiers, such as Random Forrest and Gradient Boosting Machines.
更多查看译文
关键词
training robust model,random projection,machine learning system,model regularization,neural networks,random forrest machines,gradient boosting machines,image classification
AI 理解论文
溯源树
样例
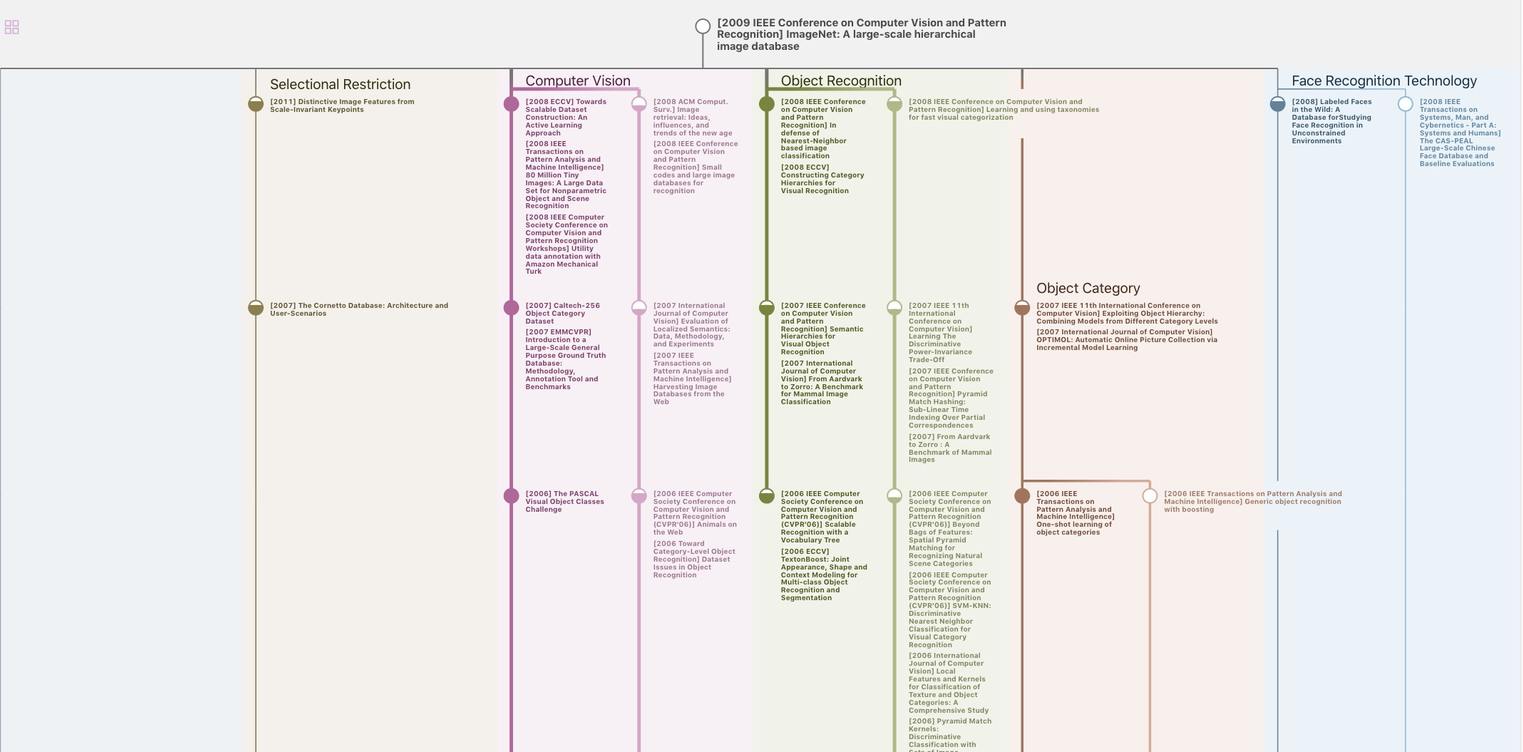
生成溯源树,研究论文发展脉络
Chat Paper
正在生成论文摘要