Leveraging Intra-Class Variations To Improve Large Vocabulary Gesture Recognition
2016 23RD INTERNATIONAL CONFERENCE ON PATTERN RECOGNITION (ICPR)(2016)
摘要
Large vocabulary gesture recognition using a training set of limited size is a challenging problem in computer vision. With few examples per gesture class, researchers often employ exemplar-based methods such as Dynamic Time Warping (DTW). This paper makes two contributions in the area of exemplar-based gesture recognition: 1) it introduces Multiple-Pass DTW (MP-DTW), a method in which scores from multiple DTW passes focusing on different gesture properties are combined, and 2) it introduces a new set of features modeling intra-class variation of several gesture properties that can be used in conjunction with MP-DTW or DTW. We demonstrate that these techniques provide substantial improvement over DTW in both user-dependent and user-independent experiments on American Sign Language (ASL) datasets, even when using noisy data generated by RGB-D skeleton detectors. We further show that using these techniques in a large vocabulary system with a limited training set provides significantly better results compared to Long Short-Term Memory (LSTM) network and Hidden Markov Model (HMM) approaches.
更多查看译文
关键词
large-vocabulary gesture recognition improvement,intra-class variations,computer vision,multiple-pass DTW,MP-DTW,gesture properties,user-independent experiments,user-dependent experiments,American sign language datasets,ASL,RGB-D skeleton detectors,noisy data generation,long short-term memory network,hidden Markov model,HMM,LSTM
AI 理解论文
溯源树
样例
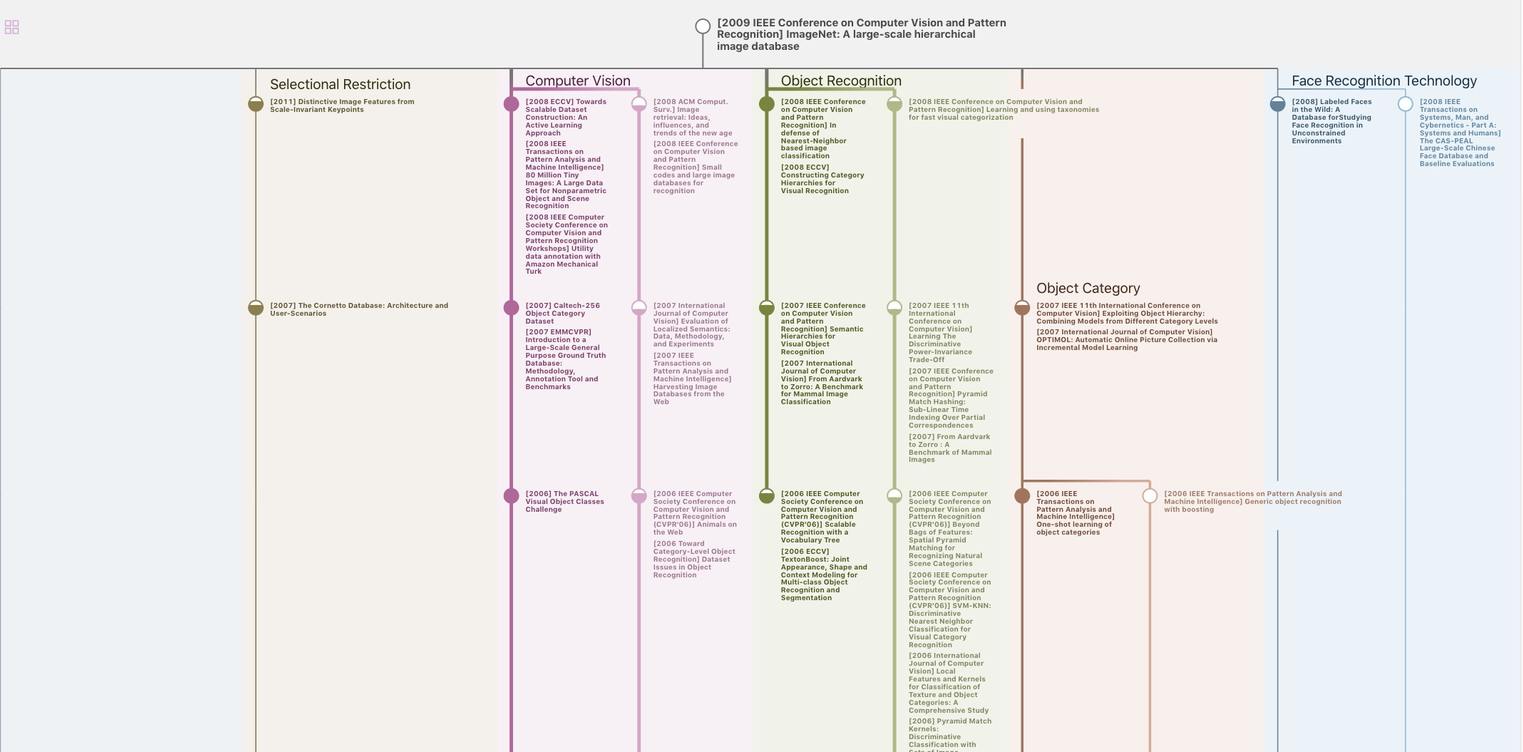
生成溯源树,研究论文发展脉络
Chat Paper
正在生成论文摘要