3d Object Recognition From Large-Scale Point Clouds With Global Descriptor And Sliding Window
2016 23RD INTERNATIONAL CONFERENCE ON PATTERN RECOGNITION (ICPR)(2016)
摘要
We propose a novel method for the recognition of objects that match a given 3D model in large-scale scene point clouds captured in indoor environments with a laser range finder. Since large-scale indoor point clouds are greatly damaged by noise such as clutter, occlusion, hole, and measurement errors, it is difficult to exactly identify local correspondences between points in a target model point cloud and points in a scene point cloud, based on similarities between local descriptors computed at keypoints on both point clouds. To avoid such a problem, we suggest to utilize sliding window in order to match the input model and pieces of scene point clouds, both of which are represented with Bag-of-Features(BoF). A BoF representation of a window is efficiently calculated by using the integral image, which stores accumulated BoF vectors. Though BoF is robust to partial noises, it does not preserve any spatial information. Then, we propose a method to make a global descriptor of a window which is almost invariant to horizontal rotations of an object inside the divided window and roughly preserves spatial information by dividing sliding window into several parts. Experiments on real world data show that our approach offers better performance than a baseline method in terms of precision and recall.
更多查看译文
关键词
3D object recognition,global descriptor,sliding window,large-scale scene point clouds,indoor environments,laser range finder,target model point cloud,bag-of-features,BoF representation,integral image,partial noises,horizontal object rotations
AI 理解论文
溯源树
样例
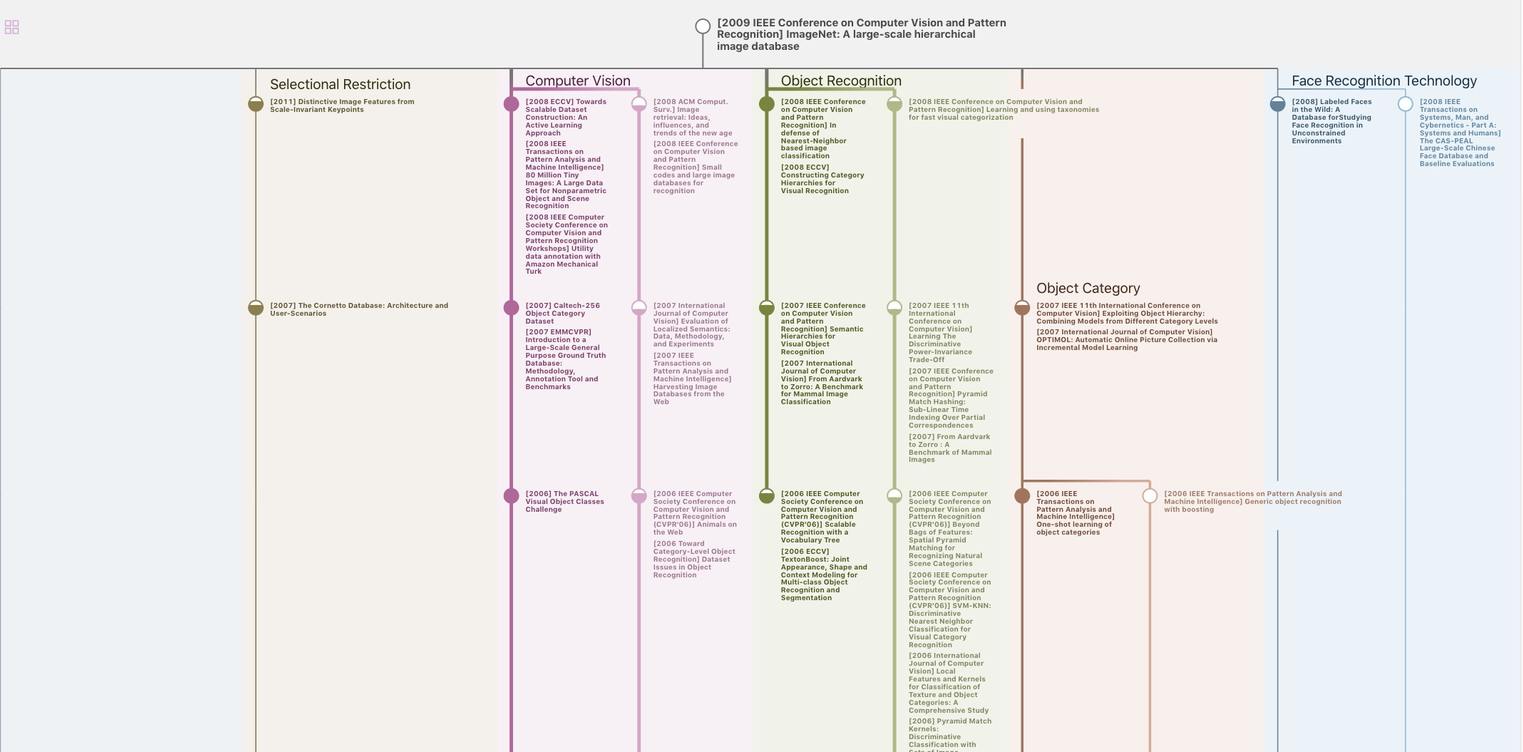
生成溯源树,研究论文发展脉络
Chat Paper
正在生成论文摘要