Transferring From Face Recognition To Face Attribute Prediction Through Adaptive Selection Of Off-The-Shelf Cnn Representations
2016 23RD INTERNATIONAL CONFERENCE ON PATTERN RECOGNITION (ICPR)(2016)
摘要
This paper addresses the problem of transferring CNNs pre-trained for face recognition to a face attribute prediction task. To transfer an off-the-shelf CNN to a novel task, a typical solution is to fine-tune the network towards the novel task. As demonstrated in the state-of-the-art face attribute prediction approach, fine-tuning the high-level CNN hidden layer by using labeled attribute data leads to significant performance improvements. In this paper, however, we tackle the same problem but through a different approach. Rather than using an end-to-end network, we select face descriptors from off-the-shelf hierarchical CNN representations for recognizing different attributes. Through such an adaptive representation selection, even without any fine-tuning, our results still outperform the state-of-the-art face attribute prediction approach on the latest large-scale dataset for an error rate reduction of more than 20%. Moreover, by using intensive empirical probes, we have identified several key factors that are significant for achieving promising face attribute prediction performance. These results attempt to gain and update our understandings of the nature of CNN features and how they can be better applied to the transferred novel tasks.
更多查看译文
关键词
adaptive representation selection,face recognition,convolutional neural network,face descriptors,off-the-shelf CNN representations,face attribute prediction
AI 理解论文
溯源树
样例
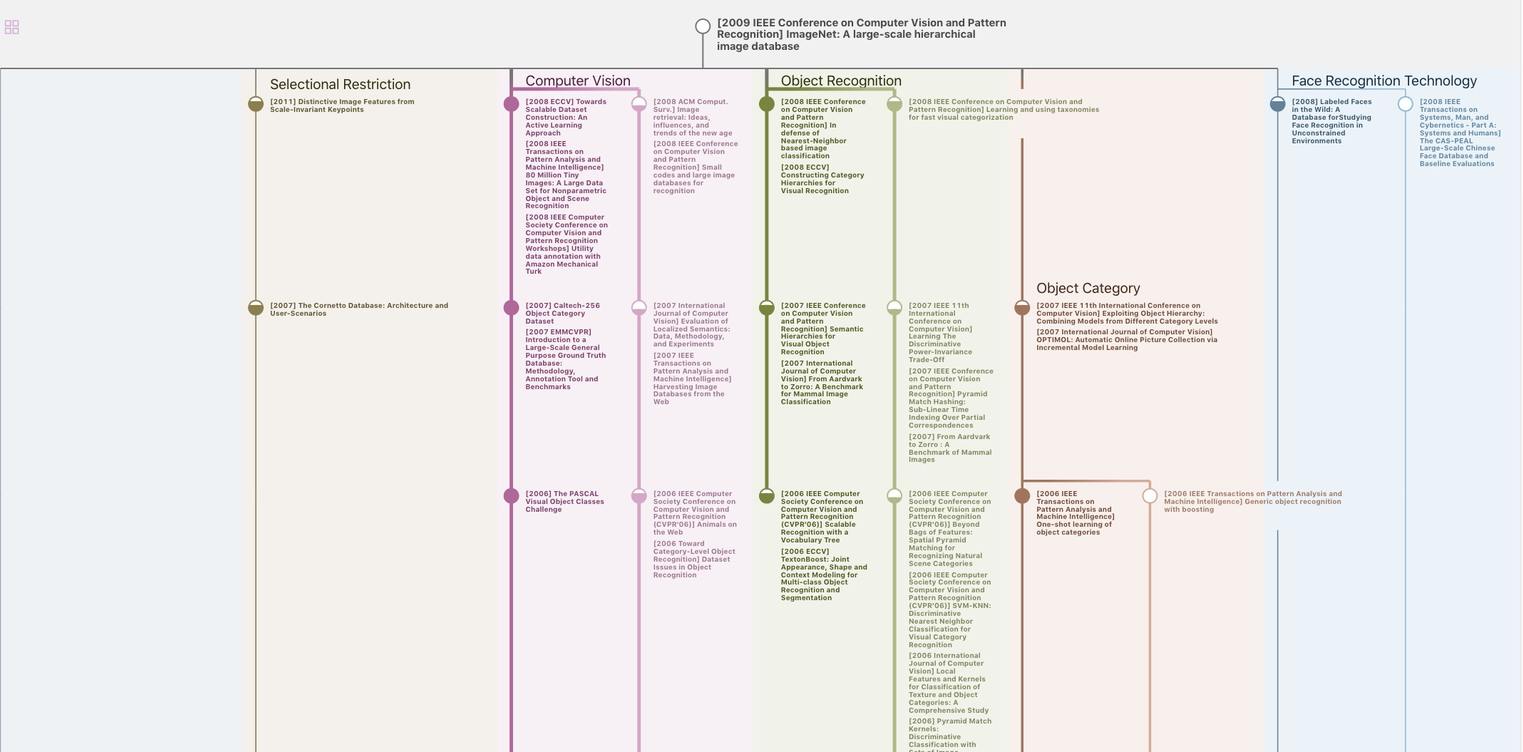
生成溯源树,研究论文发展脉络
Chat Paper
正在生成论文摘要