Effective Learning With 2-Dimensional Active Selection On Feature And Instance
INTERNATIONAL CONFERENCE ON COMPUTATIONAL SCIENCE (ICCS 2017)(2017)
摘要
Active learning is an effective way to minimize the number of queries on ground-truth labels from an oracle via human-computer interaction. Traditional active learning focus on the selection of unlabeled instances that are most informative for parameter optimization under the existing hypothesis. However, the variation of optimal feature subset and the emergence of new class labels are neglected. In this paper, we propose a novel active learning method with 2-dimentional selection on both feature and instance. For feature-dimensional selection, discriminative feature selection is implemented to extract the smallest possible subset of features that can most accurately reveal the underlying classification labels. For instance-dimensional selection, an indeterminate model is adopted to balance between model update and model upgrade. Finally, the active selection in both dimensions are integrated into a unified framework for robust model learning. (C) 2017 The Authors. Published by Elsevier B.V.
更多查看译文
关键词
active learning,feature selection,instance selection,matrix optimization,decision theory
AI 理解论文
溯源树
样例
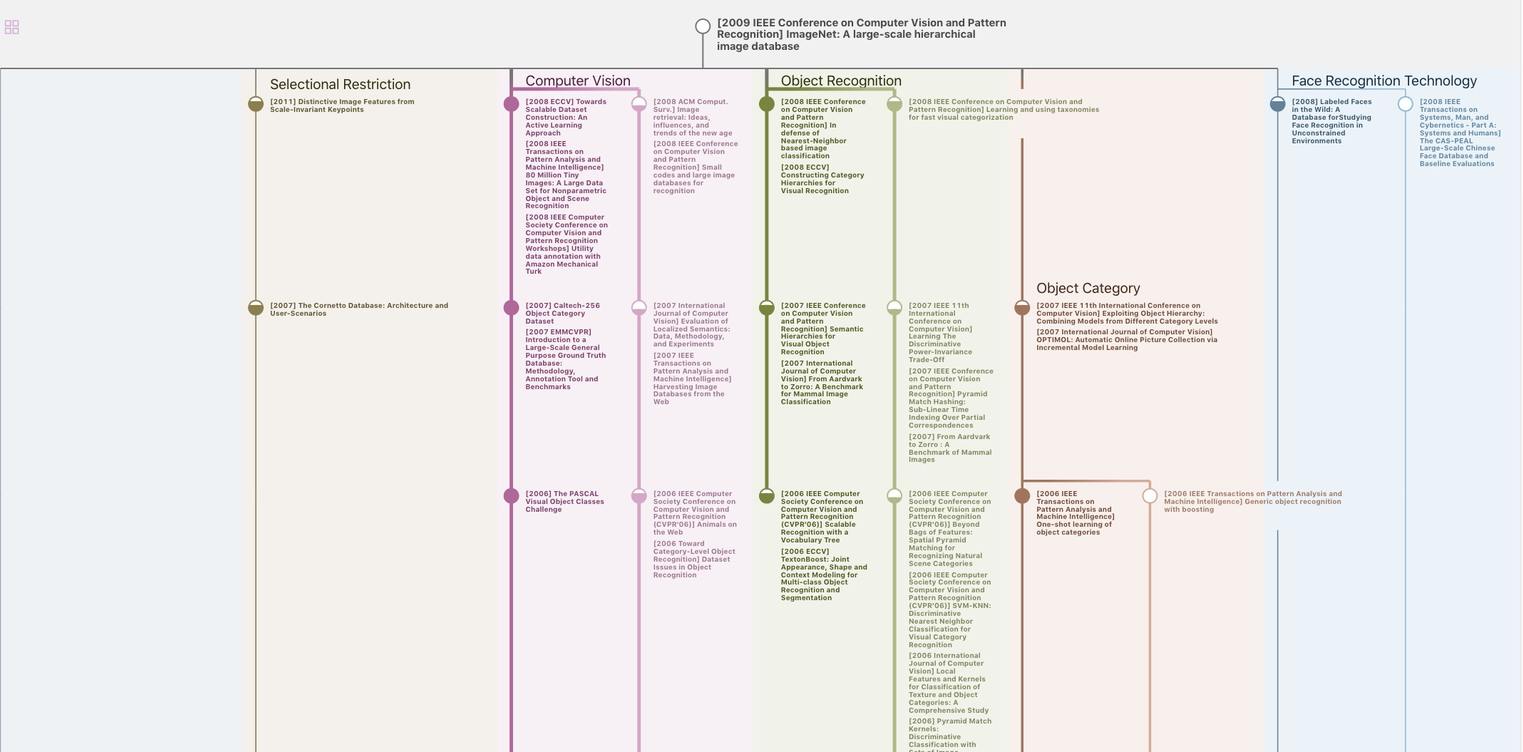
生成溯源树,研究论文发展脉络
Chat Paper
正在生成论文摘要