Enhanced Indoor Localization Through Crowd Sensing
2017 IEEE INTERNATIONAL CONFERENCE ON ACOUSTICS, SPEECH AND SIGNAL PROCESSING (ICASSP)(2017)
摘要
In localization tasks, one typically assumes a statistical model of the observations, where the model quantifies the observations by exploiting interrelationships based on geometry. These models might incorporate unknown parameters that, in general, are functions of space. In this article, we propose a crowd sensing method for estimating a spatial field of a quantity (e.g., ranging biases due to line-of-sight/non-line-of-sight or path-loss parameter) allowing for improved indoor localization. Our method takes advantage of the information provided by various users that navigate the area of interest. The proposed learning approach is based on Gaussian processes and its computational cost does not increase with the number of measurements. We present numerical results that show how the proposed method estimates a spatial field of biases and how these estimates lead to much improved performance in estimation of user positions.
更多查看译文
关键词
indoor localization, crowd sourcing, Gaussian processes, spatial field, biases
AI 理解论文
溯源树
样例
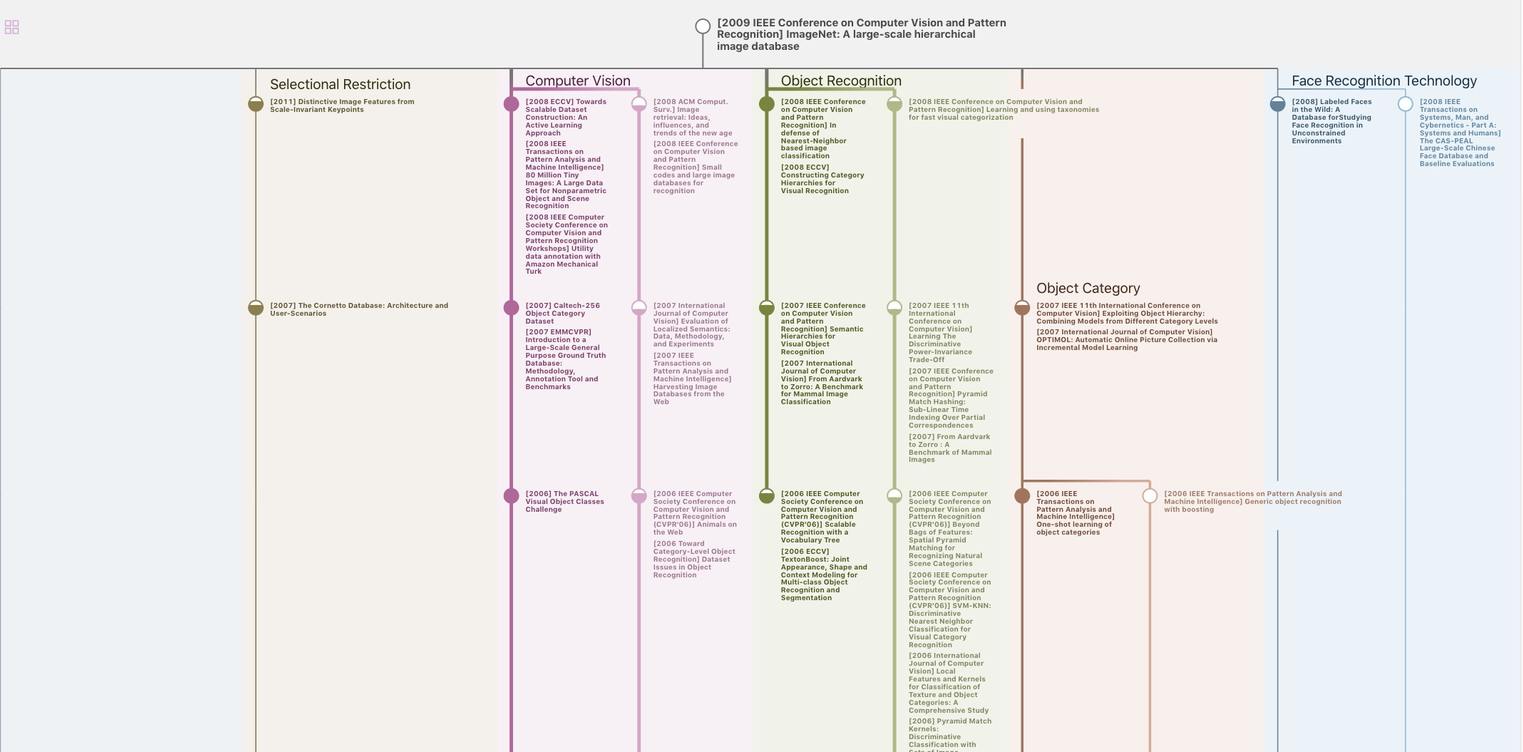
生成溯源树,研究论文发展脉络
Chat Paper
正在生成论文摘要