An investigation into language model data augmentation for low-resourced stt and kws
2017 IEEE INTERNATIONAL CONFERENCE ON ACOUSTICS, SPEECH AND SIGNAL PROCESSING (ICASSP)(2017)
摘要
This paper reports on investigations using two techniques for language model text data augmentation for low-resourced automatic speech recognition and keyword search. Lowresourced languages are characterized by limited training materials, which typically results in high out-of-vocabulary (OOV) rates and poor language model estimates. One technique makes use of recurrent neural networks (RNNs) using word or subword units. Word-based RNNs keep the same system vocabulary, so they cannot reduce the OOV, whereas subword units can reduce the OOV but generate many false combinations. A complementary technique is based on automatic machine translation, which requires parallel texts and is able to add words to the vocabulary. These methods were assessed on 10 languages in the context of the Babel program and NIST OpenKWS evaluation. Although improvements vary across languages with both methods, small gains were generally observed in terms of word error rate reduction and improved keyword search performance.
更多查看译文
关键词
multilingual,low resourced languages,speech recognition,keyword search
AI 理解论文
溯源树
样例
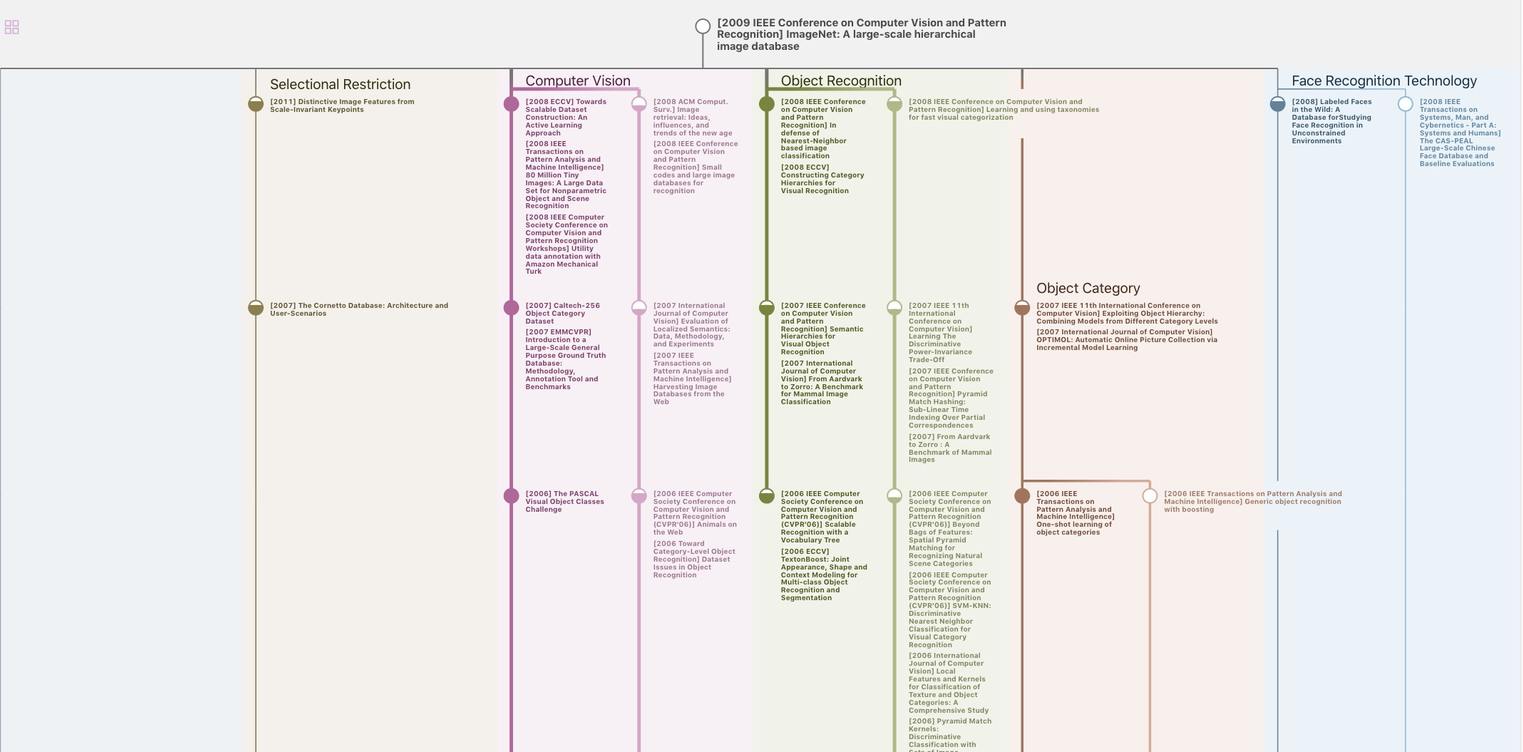
生成溯源树,研究论文发展脉络
Chat Paper
正在生成论文摘要