Deep Fusion Of Heterogeneous Sensor Data
2017 IEEE INTERNATIONAL CONFERENCE ON ACOUSTICS, SPEECH AND SIGNAL PROCESSING (ICASSP)(2017)
摘要
Heterogeneous sensor data fusion is a challenging field that has gathered significant interest in recent years. In this paper, we propose a neural network-based multimodal data fusion framework named deep multimodal encoder (DME). Through our new objective function, both the intra-and inter-modal correlations of multimodal sensor data can be better exploited for recovering the missing values, and the shared representation learned can be used directly for prediction tasks. In experiments with real-world sensor data, DME shows remarkable ability for missing data imputation and new modality prediction. Compared with traditional algorithms such as kNN and Sparse-PCA, DME is more expressive, robust, and scalable to large datasets.
更多查看译文
关键词
Multimodal data fusion, heterogeneous sensor data, missing data imputation, deep learning
AI 理解论文
溯源树
样例
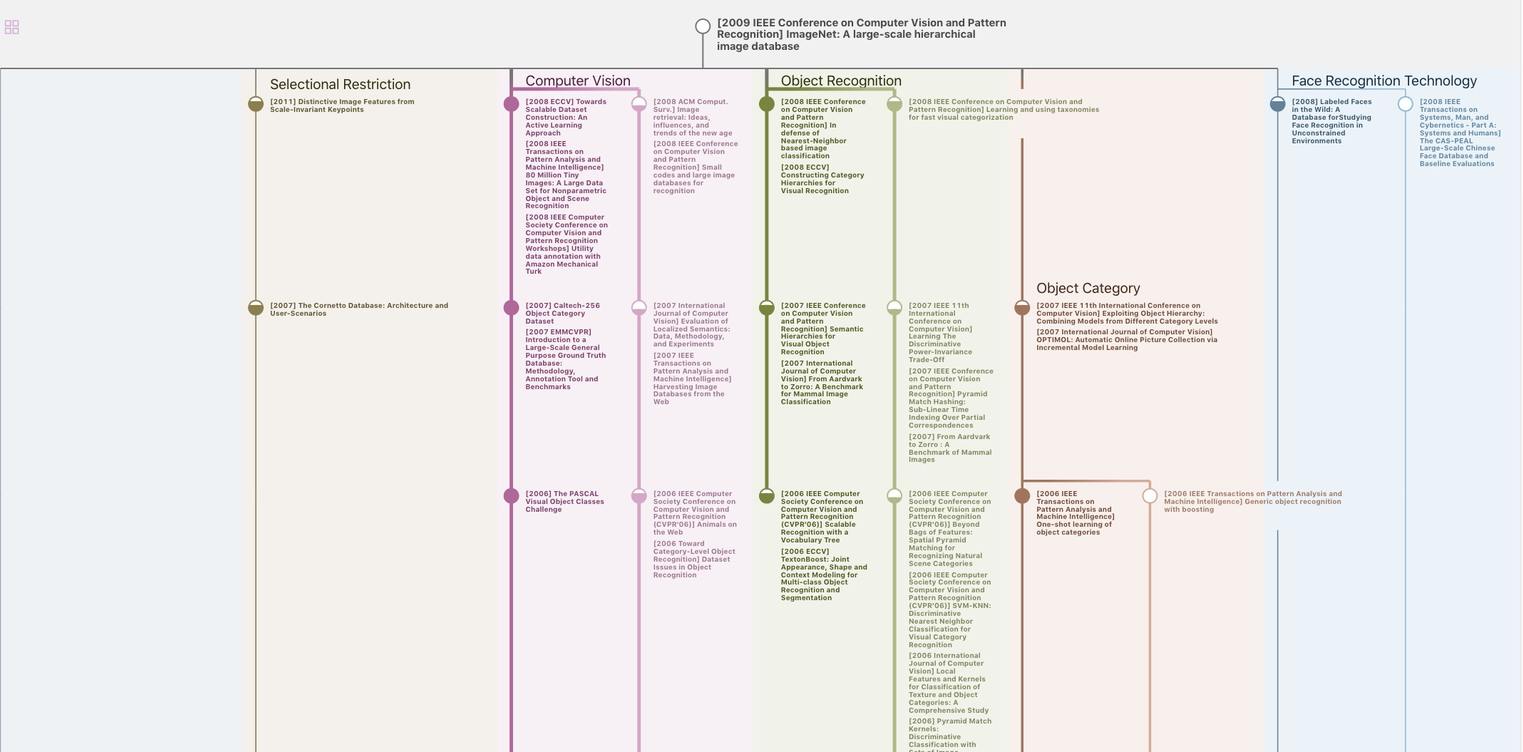
生成溯源树,研究论文发展脉络
Chat Paper
正在生成论文摘要