Learning Structured Inference Neural Networks with Label Relations
2016 IEEE CONFERENCE ON COMPUTER VISION AND PATTERN RECOGNITION (CVPR)(2016)
摘要
Images of scenes have various objects as well as abundant attributes, and diverse levels of visual categorization are possible. A natural image could be assigned with fine-grained labels that describe major components, coarse-grained labels that depict high level abstraction or a set of labels that reveal attributes. Such categorization at different concept layers can be modeled with label graphs encoding label information. In this paper, we exploit this rich information with a state-of-art deep learning framework, and propose a generic structured model that leverages diverse label relations to improve image classification performance. Our approach employs a novel stacked label prediction neural network, capturing both inter-level and intra-level label semantics. We evaluate our method on benchmark image datasets, and empirical results illustrate the efficacy of our model.
更多查看译文
关键词
structured inference neural networks,scene image,visual categorization,natural image,fine-grained labels,coarse-grained labels,high level abstraction,concept layers,label graphs,deep learning,label relations,image classification performance,stacked label prediction neural network,inter-level label semantics,intra-level label semantics
AI 理解论文
溯源树
样例
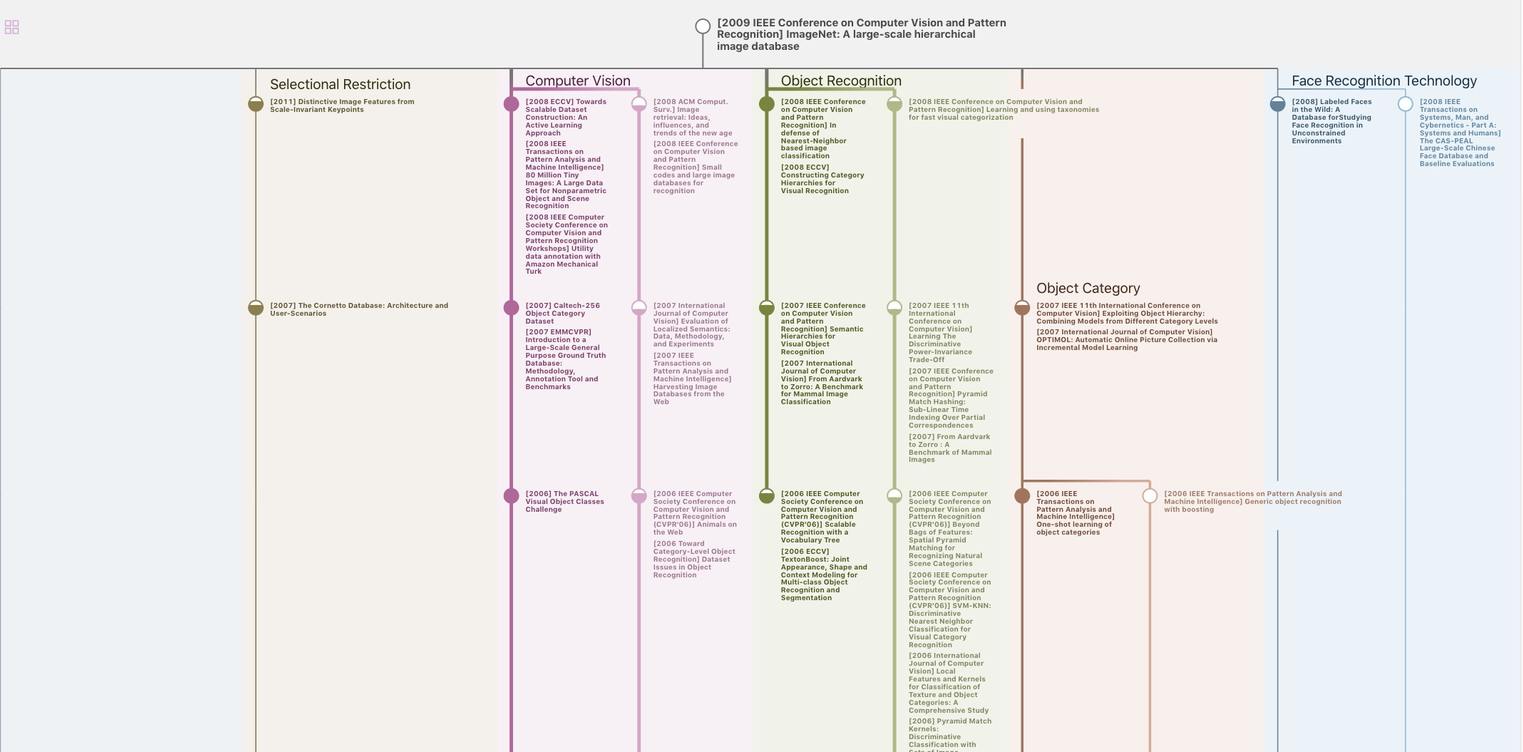
生成溯源树,研究论文发展脉络
Chat Paper
正在生成论文摘要