Learning to Recommend Accurate and Diverse Items.
WWW(2017)
摘要
In this study, we investigate diversified recommendation problem by supervised learning, seeking significant improvement in diversity while maintaining accuracy. In particular, we regard each user as a training instance, and heuristically choose a subset of accurate and diverse items as ground-truth for each user. We then represent each user or item as a vector resulted from the factorization of the user-item rating matrix. In our paper, we try to discover a factorization for matching the following supervised learning task. In doing this, we define two coupled optimization problems, parameterized matrix factorization and structural learning, to formulate our task. And we propose a diversified collaborative filtering algorithm (DCF) to solve the coupled problems. We also introduce a new pairwise accuracy metric and a normalized topic coverage diversity metric to measure the performance of accuracy and diversity respectively. Extensive experiments on benchmark datasets show the performance gains of DCF in comparison with the state-of-the-art algorithms.
更多查看译文
关键词
Diversity, Collaborative filtering, Recommender systems, Structural SVM
AI 理解论文
溯源树
样例
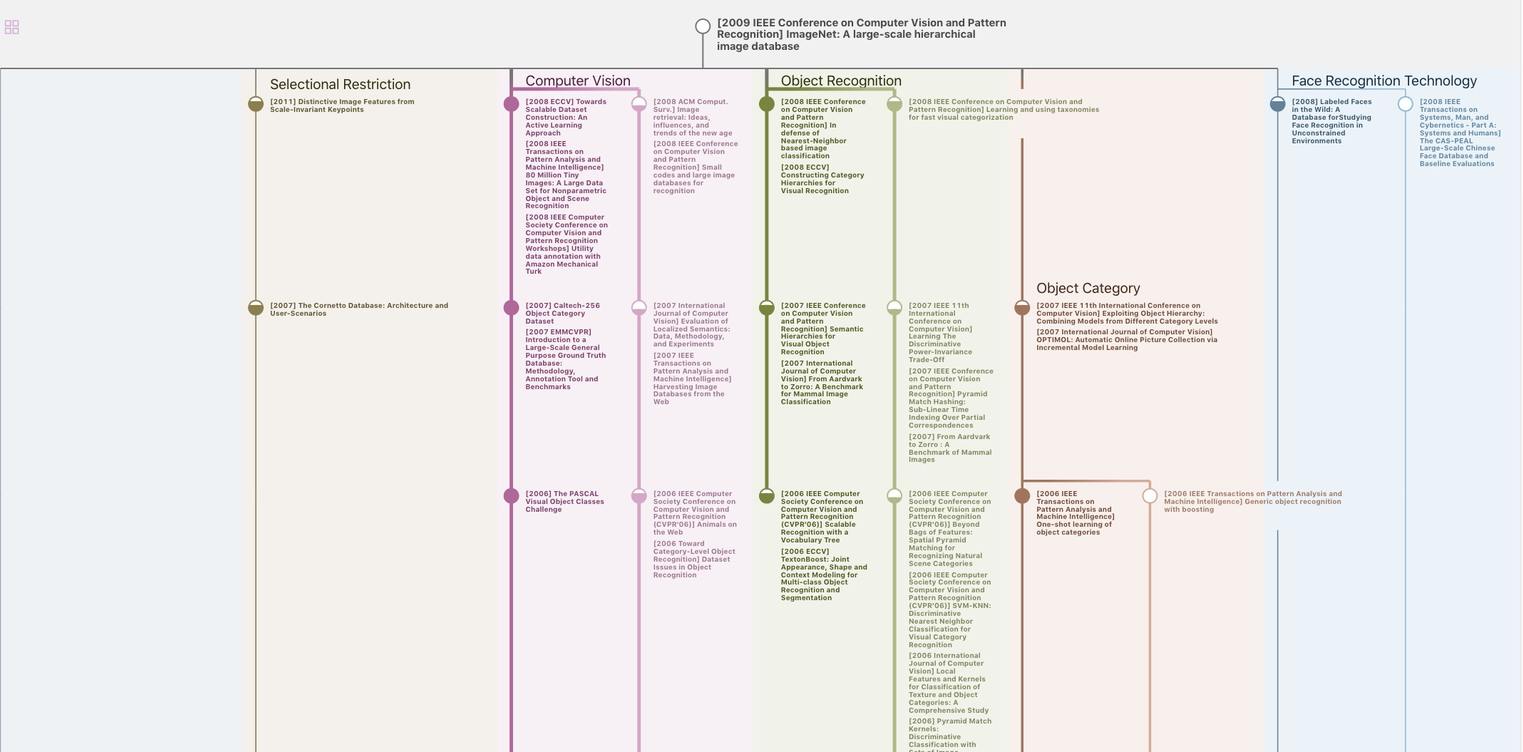
生成溯源树,研究论文发展脉络
Chat Paper
正在生成论文摘要