Predicting the Popularity of Online Content with Group-specific Models.
WWW (Companion Volume)(2017)
摘要
Predicting the popularity of online content is highly valuable in many applications and has been studied for several years. However, existing models either work in population level---all messages are assumed to follow similar popularity dynamics, lacking flexibility to capture the intrinsic complexity of popularity dynamics, or work in individual level---the popularity dynamics of messages are independent of each other, failing to leverage other messages to improve prediction accuracy. In this paper, we propose a divide and conquer framework for popularity prediction. We first divide messages into groups, anticipating each group of messages follow similar popularity dynamics, and then, we train a group-specific model for the messages of each group. Experiments demonstrate that group-specific models improve the population-level models by about 30% and outperform state-of-the-art individual-level model.
更多查看译文
AI 理解论文
溯源树
样例
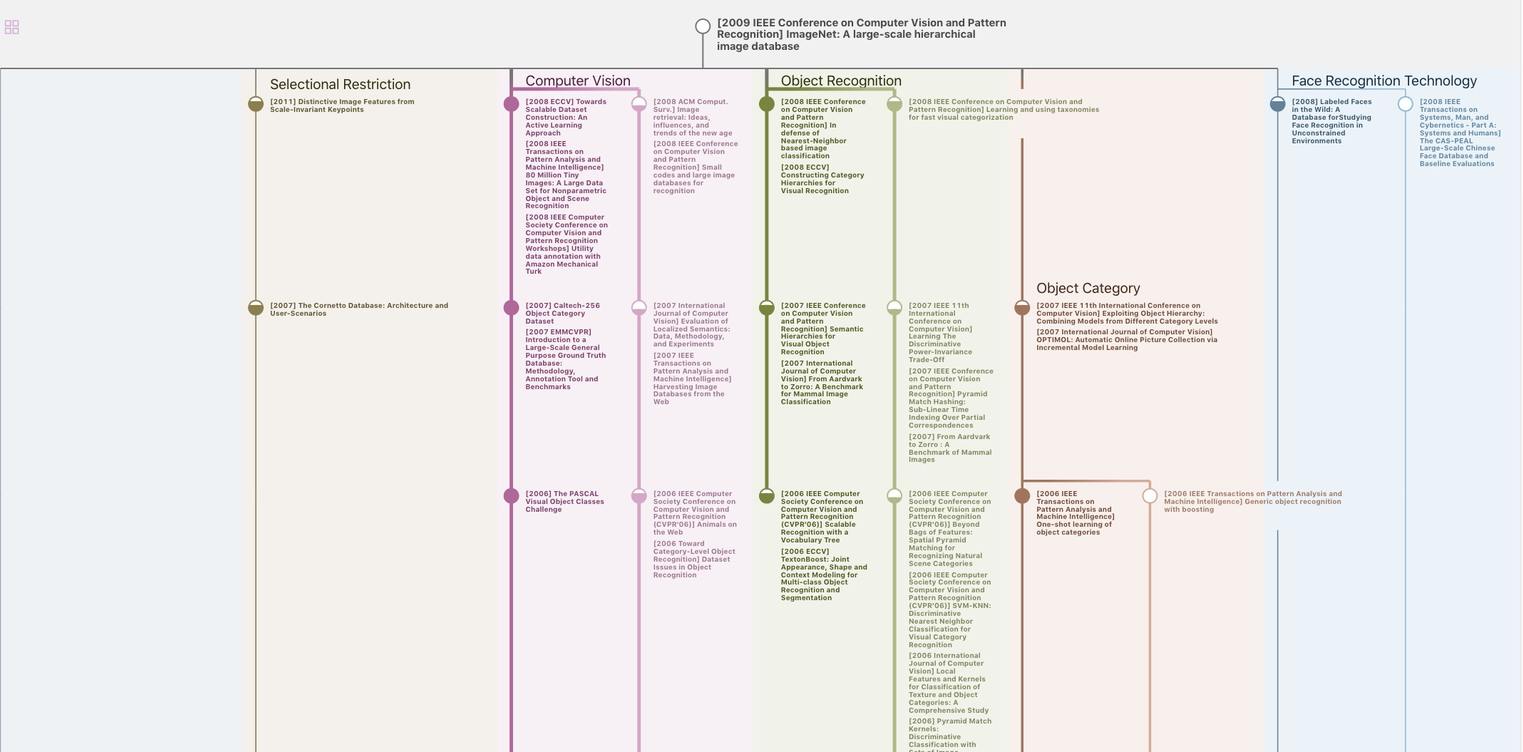
生成溯源树,研究论文发展脉络
Chat Paper
正在生成论文摘要