Bayesian Learning And Inference In Recurrent Switching Linear Dynamical Systems
ARTIFICIAL INTELLIGENCE AND STATISTICS, VOL 54(2017)
摘要
Many natural systems, such as neurons firing in the brain or basketball teams traversing a court, give rise to time series data with complex, nonlinear dynamics. We can gain insight into these systems by decomposing the data into segments that are each explained by simpler dynamic units. Building on switching linear dynamical systems (SLDS), we develop a model class and Bayesian inference algorithms that not only discover these dynamical units but also, by learning how transition probabilities depend on observations or continuous latent states, explain their switching behavior. Our key innovation is to design these recurrent SLDS models to enable recent Polya-gamma auxiliary variable techniques and thus make approximate Bayesian learning and inference in these models easy, fast, and scalable.
更多查看译文
AI 理解论文
溯源树
样例
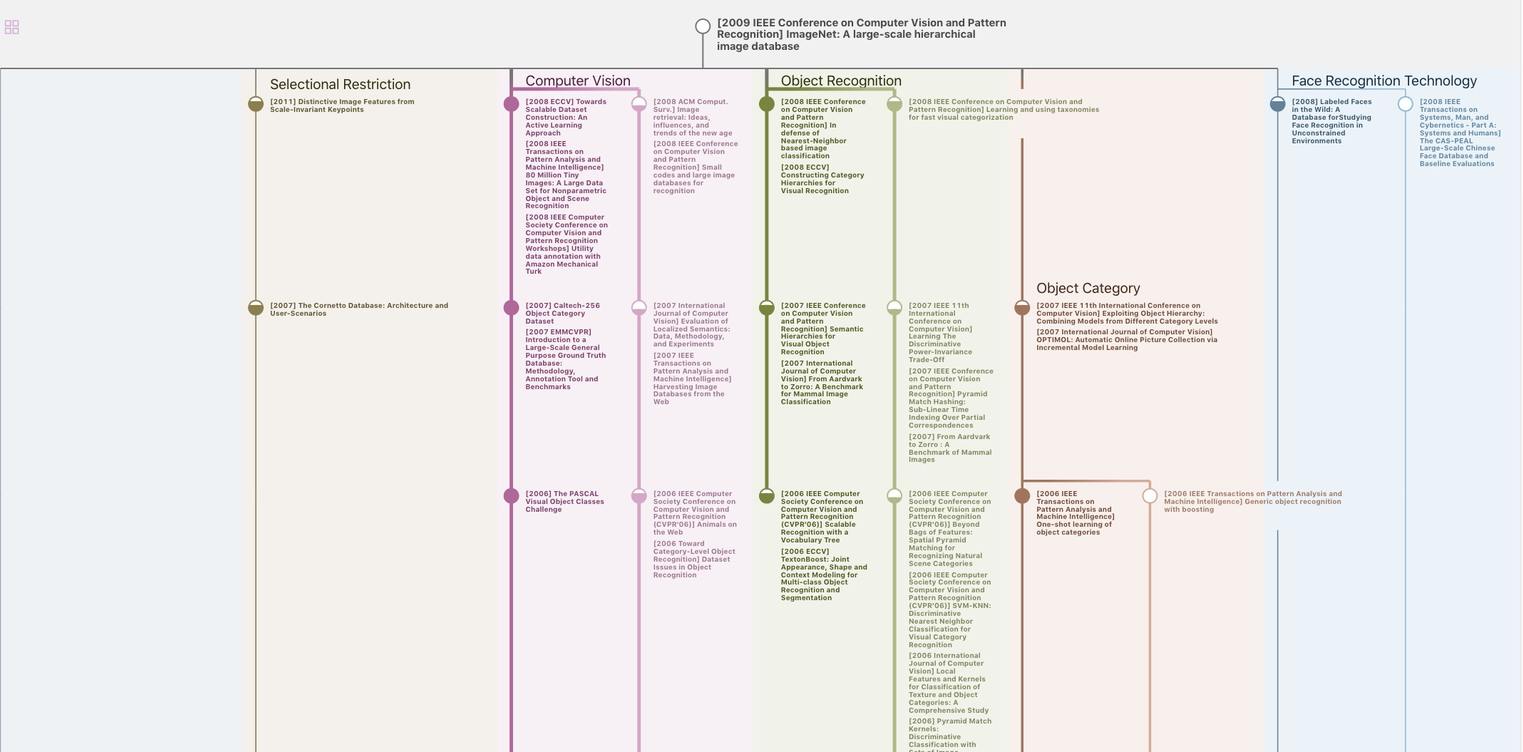
生成溯源树,研究论文发展脉络
Chat Paper
正在生成论文摘要