Balancing Learning And Engagement In Game-Based Learning Environments With Multi-Objective Reinforcement Learning
ARTIFICIAL INTELLIGENCE IN EDUCATION, AIED 2017(2017)
摘要
Game-based learning environments create rich learning experiences that are both effective and engaging. Recent years have seen growing interest in data-driven techniques for tutorial planning, which dynamically personalize learning experiences by providing hints, feedback, and problem scenarios at run-time. In game-based learning environments, tutorial planners are designed to adapt gameplay events in order to achieve multiple objectives, such as enhancing student learning or student engagement, which may be complementary or competing aims. In this paper, we introduce a multi-objective reinforcement learning framework for inducing game-based tutorial planners that balance between improving learning and engagement in game-based learning environments. We investigate a model-based, linear-scalarized multi-policy algorithm, Convex Hull Value Iteration, to induce a tutorial planner from a corpus of student interactions with a game-based learning environment for middle school science education. Results indicate that multi-objective reinforcement learning creates policies that are more effective at balancing multiple reward sources than single-objective techniques. A qualitative analysis of select policies and multi-objective preference vectors shows how a multi-objective reinforcement learning framework shapes the selection of tutorial actions during students' game-based learning experiences to effectively achieve targeted learning and engagement outcomes.
更多查看译文
关键词
Tutorial planning,Multi-objective reinforcement learning,Game-based learning environments,Narrative centered learning
AI 理解论文
溯源树
样例
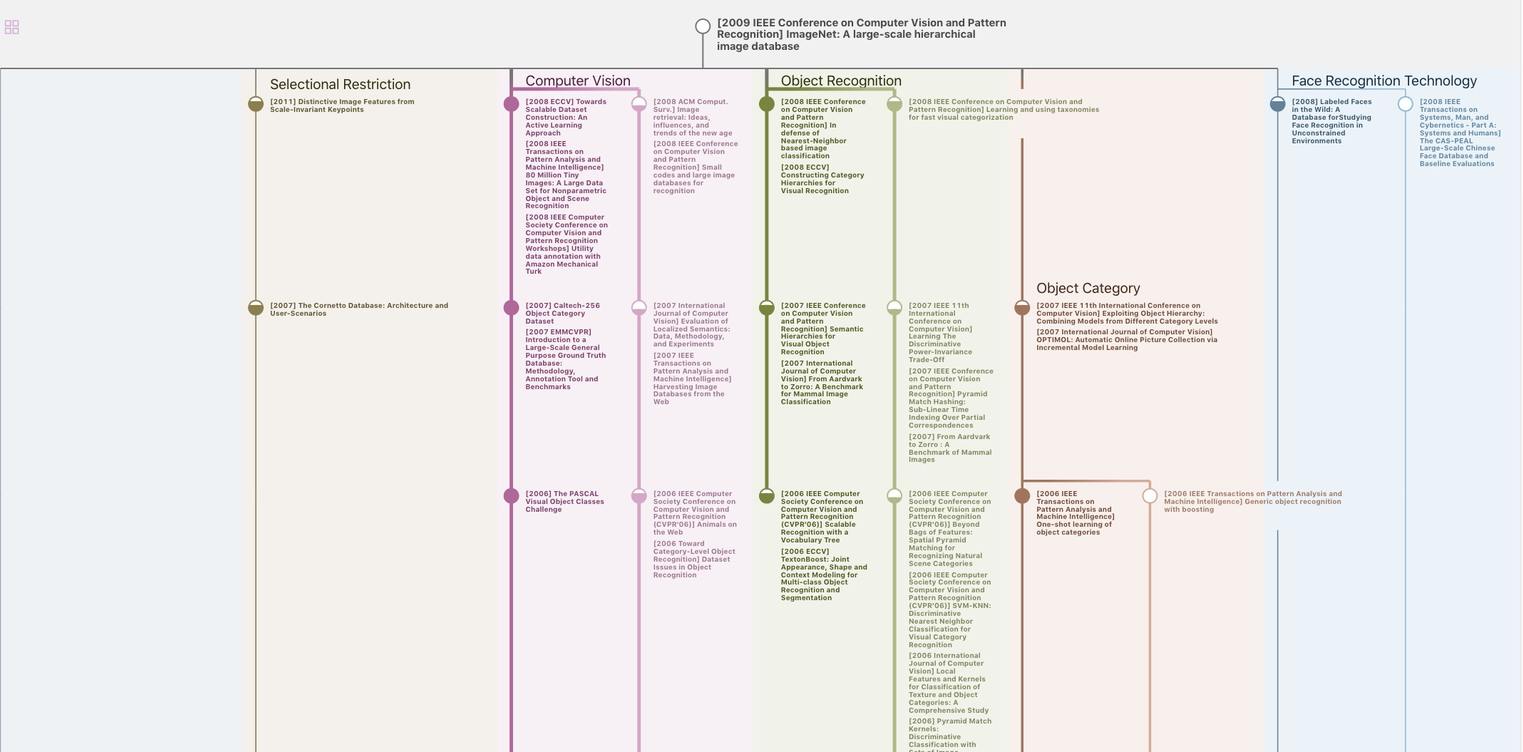
生成溯源树,研究论文发展脉络
Chat Paper
正在生成论文摘要