Quasilinearization-Based Controllability Analysis Of Neuronal Rate Networks
2016 AMERICAN CONTROL CONFERENCE (ACC)(2016)
摘要
Recent interest has developed around the problem of assaying the controllability of networks in the brain. The analysis of such networks is highly nontrivial, owing to their overwhelming complexity. Thus, any controllability analysis must tradeoff against model complexity/explanatory power, and analysis tractability. Here, we consider a class of neuronal network models with nearly linear dynamics, whose primary complication arises due to a sigmoidal nonlinearity in the neuronal coupling. Exploiting the equivalence between the controllability gramian and the steady state covariance matrix of a linear system under white noise, we develop an approximate controllability analysis based on the method of stochastic linearization (quasilinearization). We show that for this relatively simple system, the quasilinear approach generates a significantly better characterization of controllability as compared with a Jacobian linearization. Our results provide a new tool for assessing controllability of networks with sigmoidal interactions, and, moreover, highlight the potential inaccuracy of linear characterizations of networks with even relatively mild nonlinearities.
更多查看译文
关键词
quasilinearization-based controllability analysis,neuronal rate networks,brain,model complexity/explanatory power,analysis tractability,neuronal network models,linear dynamics,sigmoidal nonlinearity,neuronal coupling,controllability gramian,steady state covariance matrix,linear system,white noise,approximate controllability analysis,stochastic linearization method,Jacobian linearization,sigmoidal interactions,network linear characterizations
AI 理解论文
溯源树
样例
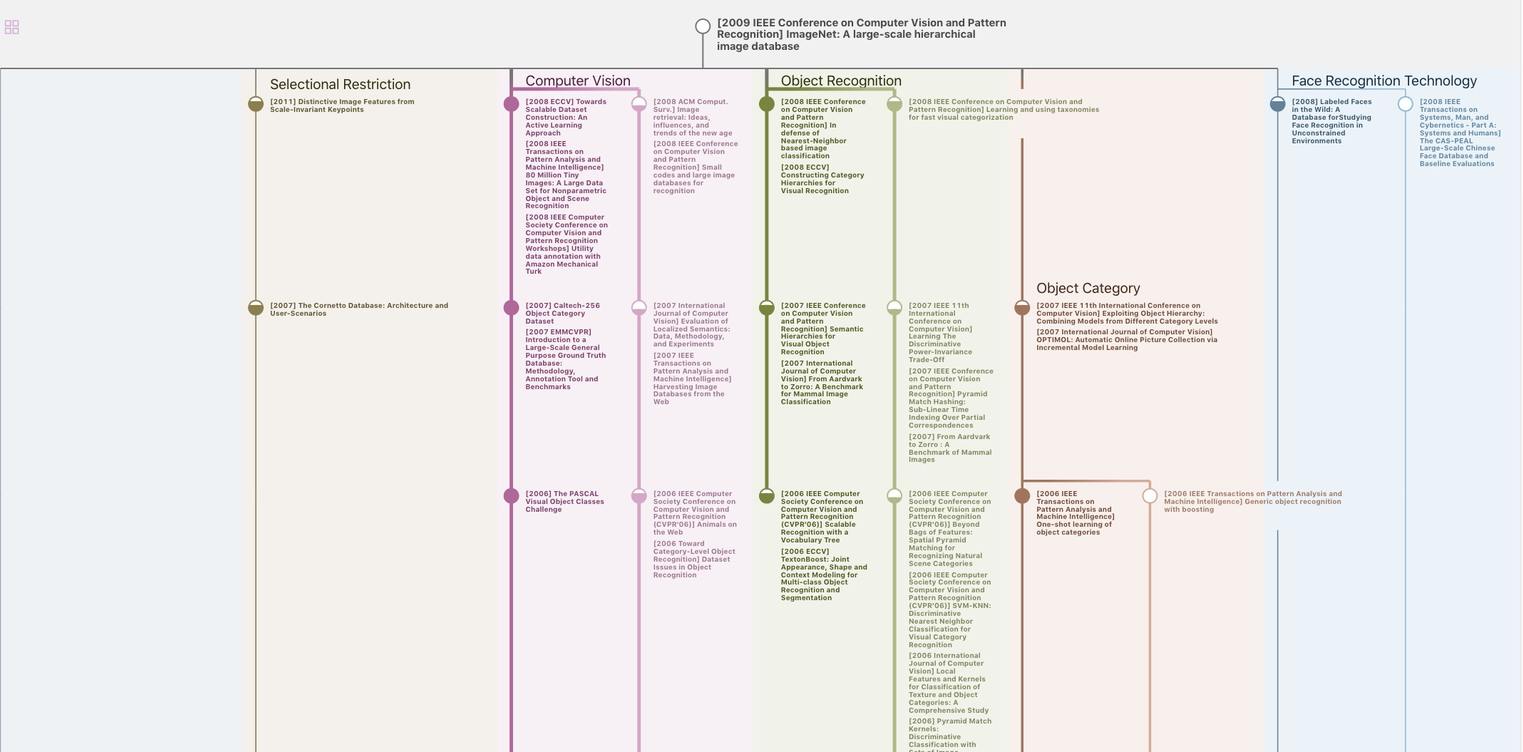
生成溯源树,研究论文发展脉络
Chat Paper
正在生成论文摘要