User Perception of Next-Track Music Recommendations
UMAP(2017)
摘要
Many of today's music streaming websites and apps provide personalized next-track listening recommendations based on the user's current and past listening behavior. In the research literature, various algorithmic approaches to determine suitable next tracks can be found. However, almost all of them were evaluated in offline experiments using, for example, manually created playlists as a gold standard. In this work, we aim to check the external validity of insights that are obtained through such offline experiments on historical datasets. We conducted an online user study involving 277 subjects in which the participants evaluated the suitability of four different alternatives of continuing a given set of playlists. Our results indicate that manually created playlists can in fact represent a reasonable gold standard, an insight for which no evidence existed in the literature before. Furthermore, our work was able to confirm that considering playlist homogeneity aspects does not only lead to performance improvements in offline experiments -- as indicated by past research -- but also to a better quality perception by users. However, the observations also revealed that user studies of this type can be easily distorted by item familiarity biases, because the participants tend to evaluate continuation alternatives better when they know the track or the artist.
更多查看译文
AI 理解论文
溯源树
样例
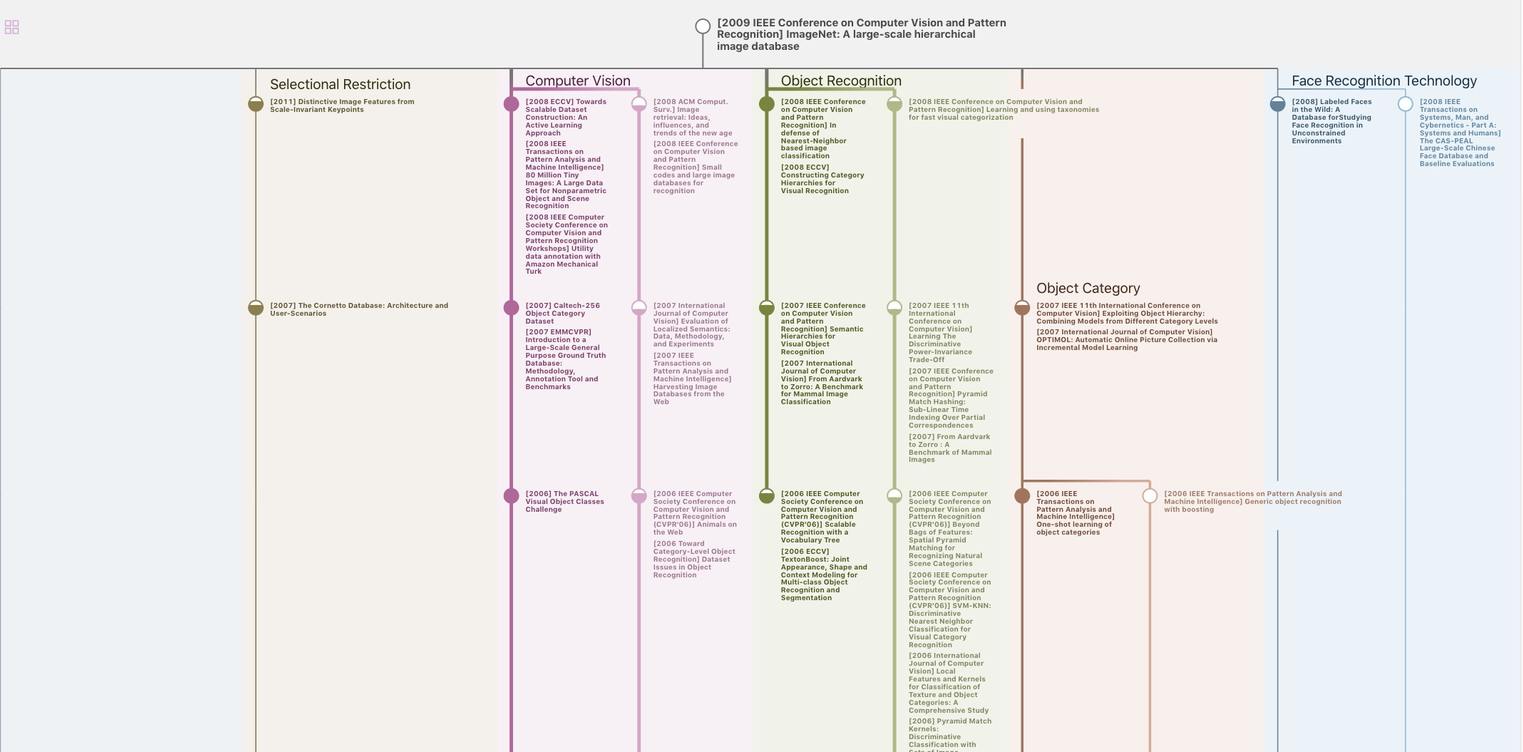
生成溯源树,研究论文发展脉络
Chat Paper
正在生成论文摘要