Item Contents Good, User Tags Better: Empirical Evaluation of a Food Recommender System
UMAP(2017)
摘要
Traditional food recommender systems exploit items' ratings and descriptions in order to generate relevant recommendations for the users. While this data is important, it might not entirely capture the true users' preferences. In this paper, we analyse the performance of a food recommender that allows users to enter their preferences in the form of both ratings and tags, which are then used by a Matrix Factorization (MF) rating prediction model. The performed offline and online experiments have clarified the importance of user tags in comparison to content features. While item content contributes more to the quality of the prediction accuracy, user tags yields better ranking quality. Even more importantly, a live user study has revealed that a system variant, which leverages user tags in the prediction model and in the interface, achieves a significantly better user evaluation in terms of perceived effectiveness, choice satisfaction and choice difficulty.
更多查看译文
AI 理解论文
溯源树
样例
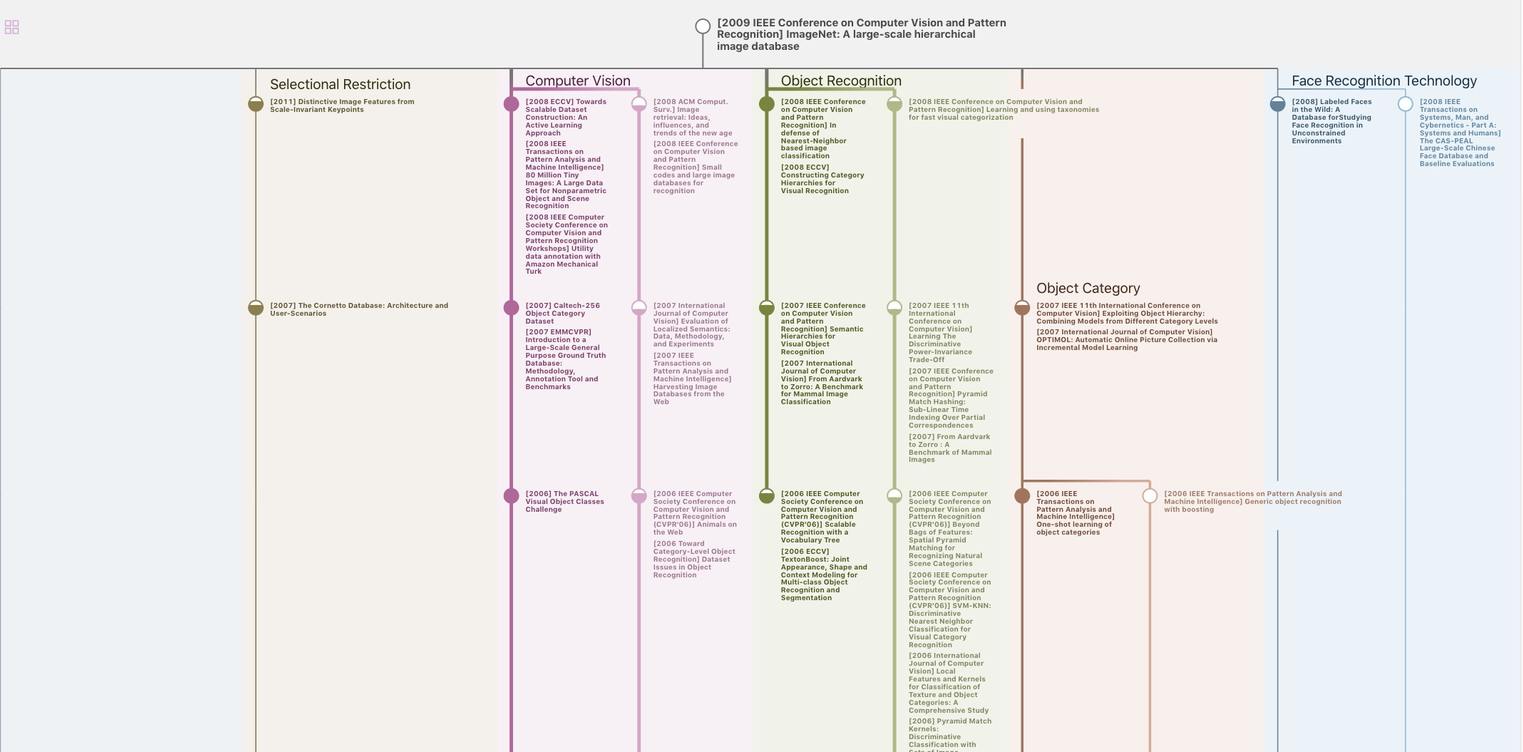
生成溯源树,研究论文发展脉络
Chat Paper
正在生成论文摘要