On How to Design Dataflow FPGA-Based Accelerators for Convolutional Neural Networks.
ISVLSI(2017)
摘要
In the past few years we have experienced an extremely rapid growth of modern applications based on deep learning algorithms such as Convolutional Neural Network (CNN), and consequently, an intensification of academic and industrial research focused on the optimization of their imple- mentation. Among the different alternatives that have been ex- plored, FPGAs seems to be one of the most attractive, as they are able to deliver high performance and energy-efficiency, thanks to their inherent parallelism and direct hardware execution, while retaining extreme flexibility due to their reconfigurability.In this paper we present a design methodology of a dataflow accelerator for the implementation of CNNs on FPGAs, that ensures scalability – and achieve a higher degree of parallelism as the size of the CNN increases – and an efficient exploitation of the available resources. Furthermore, we analyze resource consumption of the layers of the CNN as well as latency in relation to the implementationu0027s hyperparameters. Finally, we show that the proposed design implements a high-level pipeline between the different network layers, and as a result, we can improve the latency to process an image by feeding the CNN with batches of multiple images.
更多查看译文
关键词
Field Programmable Gate Arrays,Convolutional Neural Networks,Dataflow Architectures
AI 理解论文
溯源树
样例
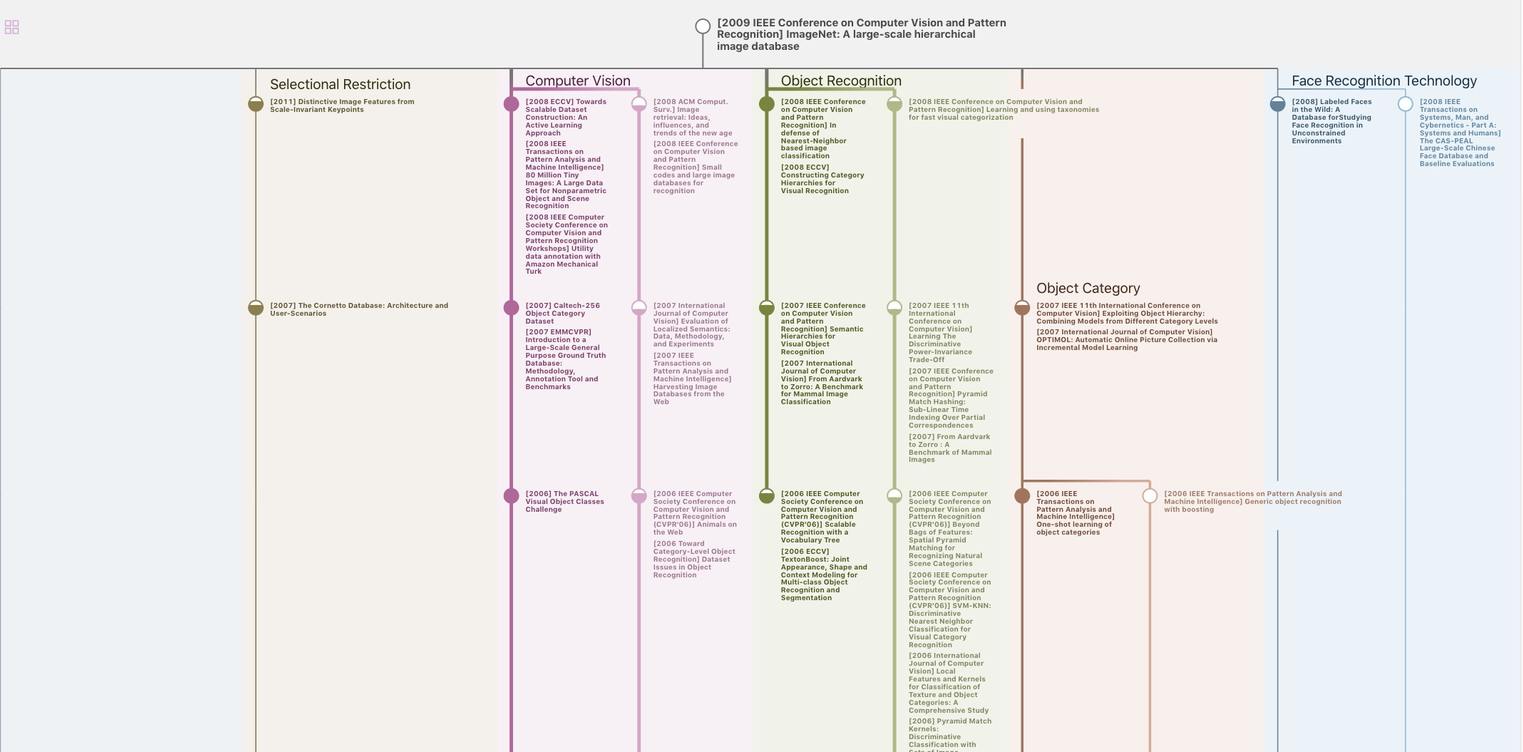
生成溯源树,研究论文发展脉络
Chat Paper
正在生成论文摘要