Topic Model-Based Road Network Inference from Massive Trajectories
2017 18th IEEE International Conference on Mobile Data Management (MDM)(2017)
摘要
Recent years witnessed popular use of various mobile devices, e.g., smart phones, vehicle networks and wearable watches. Such mobile devices generate massive trajectory data, and literature have proposed various algorithms to leverage the trajectory data for map inference. Unfortunately, such algorithms are hard to achieve both high map quality and computation efficiency. In this paper, we propose a solution framework to infer road network maps with high quality and efficiency. The key of our map inference is to divide map extent into smaller cells and maintain a binary cell-trajectory matrix. The binary matrix determines whether or not a trajectory passes a cell. We infer the importance of each cell from the matrix using a popular topic model (e.g., LDA [13] and pLSA [8]). Based on such computed importance, we next infer representative points and road segments to derive a road network map. Our extensive experiments on real data sets verify that the proposed inference algorithm can achieve higher map quality and meanwhile 1.5 ×, 6.8 × and 280 × shorter running time, when compared with three state of the arts including three representative work [4], [7], [14].
更多查看译文
关键词
topic model-based road network inference,massive trajectories,mobile devices,smart phones,vehicle networks,wearable watches,trajectory data,map inference,high map quality,computation efficiency,binary cell-trajectory matrix,road segments,road network map
AI 理解论文
溯源树
样例
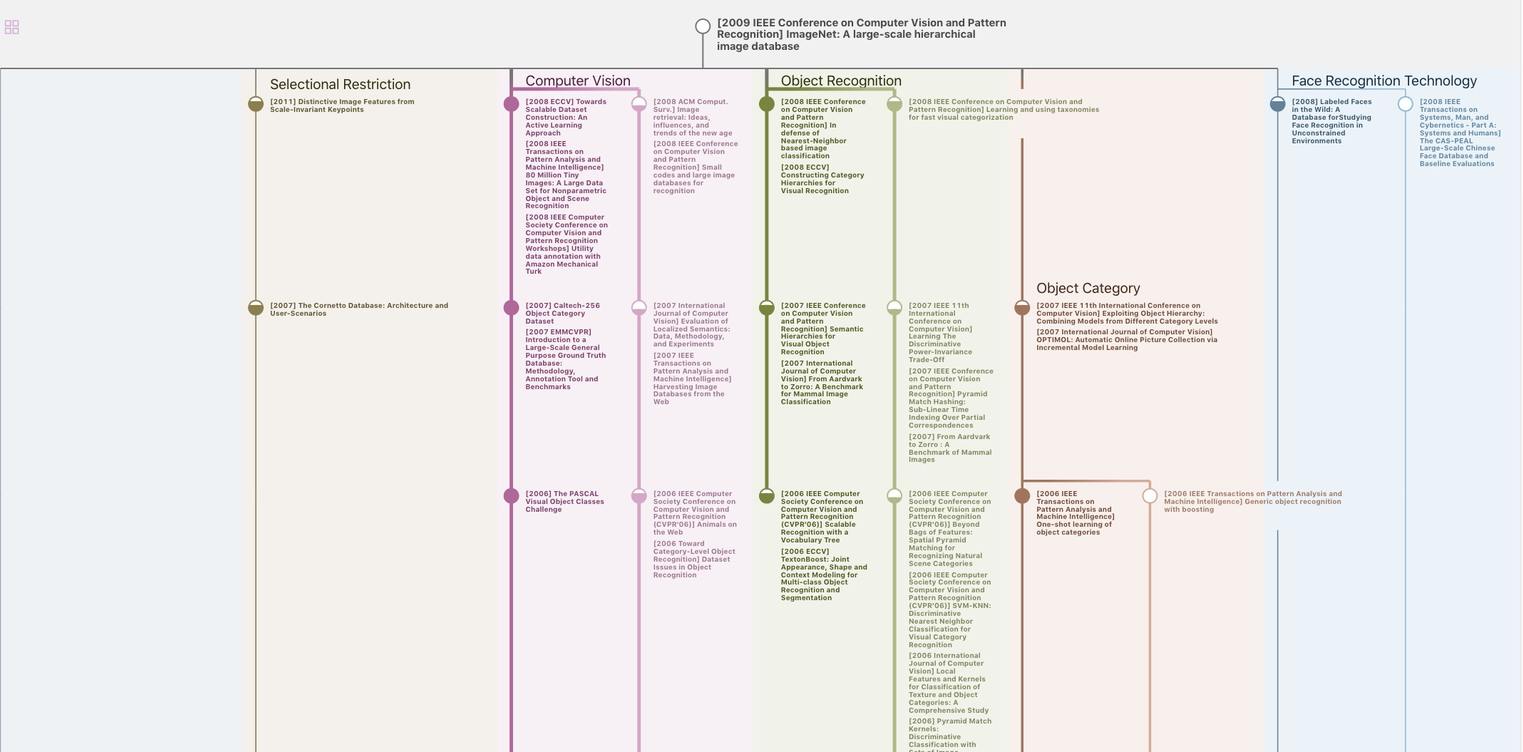
生成溯源树,研究论文发展脉络
Chat Paper
正在生成论文摘要