Comparison of Automatic Vessel Segmentation Techniques for Whole Body Magnetic Resonance Angiography with Limited Ground Truth Data.
MIUA(2017)
摘要
This work is part of a project aimed at automatically detecting vascular disease in whole body magnetic resonance angiograms (WBMRA). Here we present a comparison of four techniques for automatic artery segmentation in WBMRA data volumes; active contours, two “vesselness” filter approaches (the Frangi filter and Optimally Oriented Flux (OOF)) and a convolutional neural network (Convnet) trained for voxel-wise classification. Their performance was assessed on three manually segmented WBMRA datasets, comparing the maximum Dice Similarity Coefficient (DSC) achieved by each method. Our results show that, in the presence of limited training data, OOF performs best for our three patients, achieving a mean DSC of 0.71 across all patients. By comparison, the 3D Convnet achieved a mean DSC of 0.63. We discuss the potential reasons for these differences, and the implications it has for the automated segmentation of arteries in large WBMRA datasets, where ground truth data is often limited and there are currently no pre-trained 3D Convnet models available, requiring models to be trained from scratch. To the best of our knowledge this is the first comparison of these automated vessel segmentation techniques for WBMRA data, and the first quantitative results of applying a Convnet to vessel segmentation in WBMRA, for which no public sets of manually annotated vascular networks currently exist.
更多查看译文
关键词
automatic vessel segmentation techniques,angiography
AI 理解论文
溯源树
样例
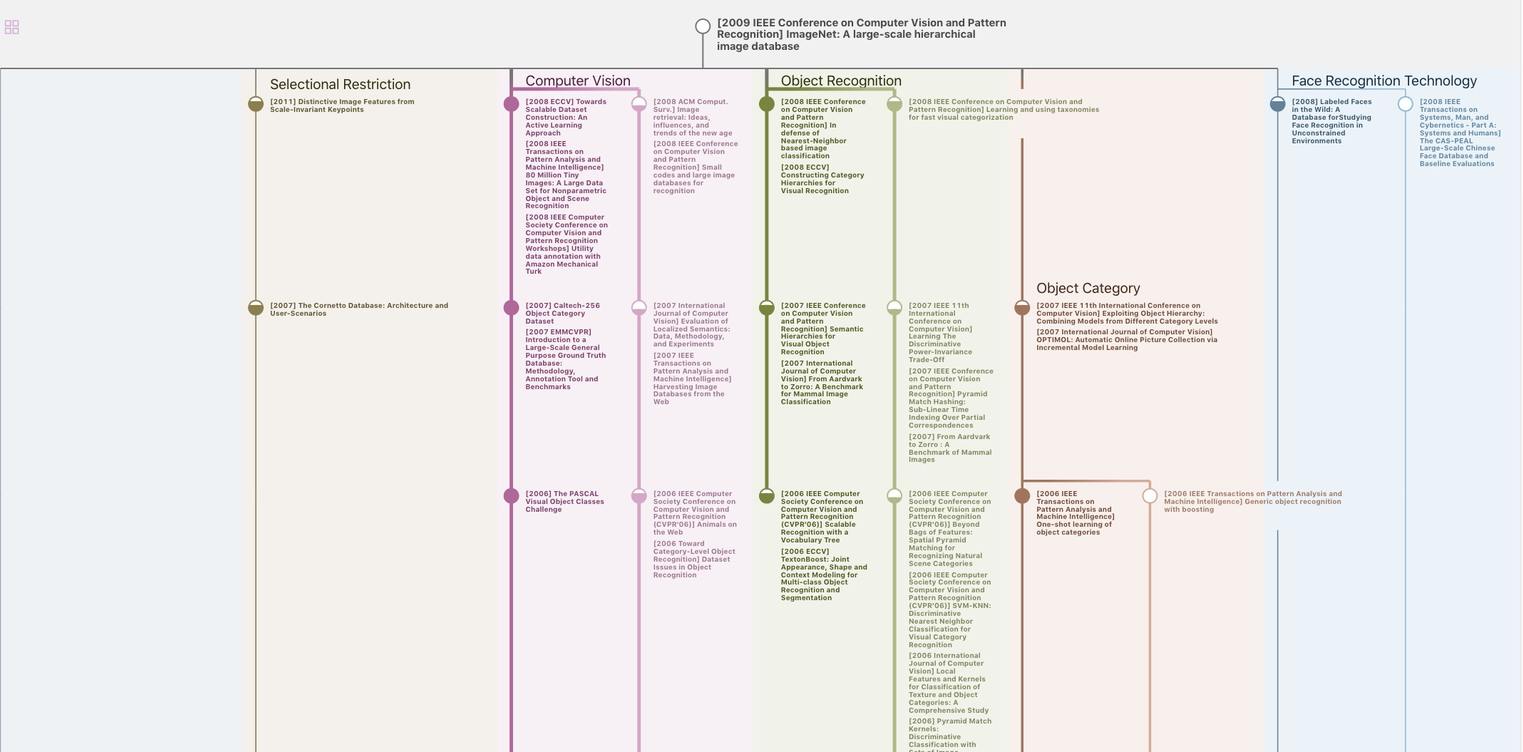
生成溯源树,研究论文发展脉络
Chat Paper
正在生成论文摘要