Exploring Time-Sensitive Variational Bayesian Inference LDA for Social Media Data.
ADVANCES IN INFORMATION RETRIEVAL, ECIR 2017(2017)
摘要
There is considerable interest among both researchers and the mass public in understanding the topics of discussion on social media as they occur over time. Scholars have thoroughly analysed sampling-based topic modelling approaches for various text corpora including social media; however, another LDA topic modelling implementation-Variational Bayesian (VB)-has not been well studied, despite its known efficiency and its adaptability to the volume and dynamics of social media data. In this paper, we examine the performance of the VB-based topic modelling approach for producing coherent topics, and further, we extend the VB approach by proposing a novel time-sensitive Variational Bayesian implementation, denoted as TVB. Our newly proposed TVB approach incorporates time so as to increase the quality of the generated topics. Using a Twitter dataset covering 8 events, our empirical results show that the coherence of the topics in our TVB model is improved by the integration of time. In particular, through a user study, we find that our TVB approach generates less mixed topics than state-of-the-art topic modelling approaches. Moreover, our proposed TVB approach can more accurately estimate topical trends, making it particularly suitable to assist end-users in tracking emerging topics on social media.
更多查看译文
关键词
Beta Distribution, Topic Modelling, Latent Dirichlet Allocation, Balance Parameter, Social Media Data
AI 理解论文
溯源树
样例
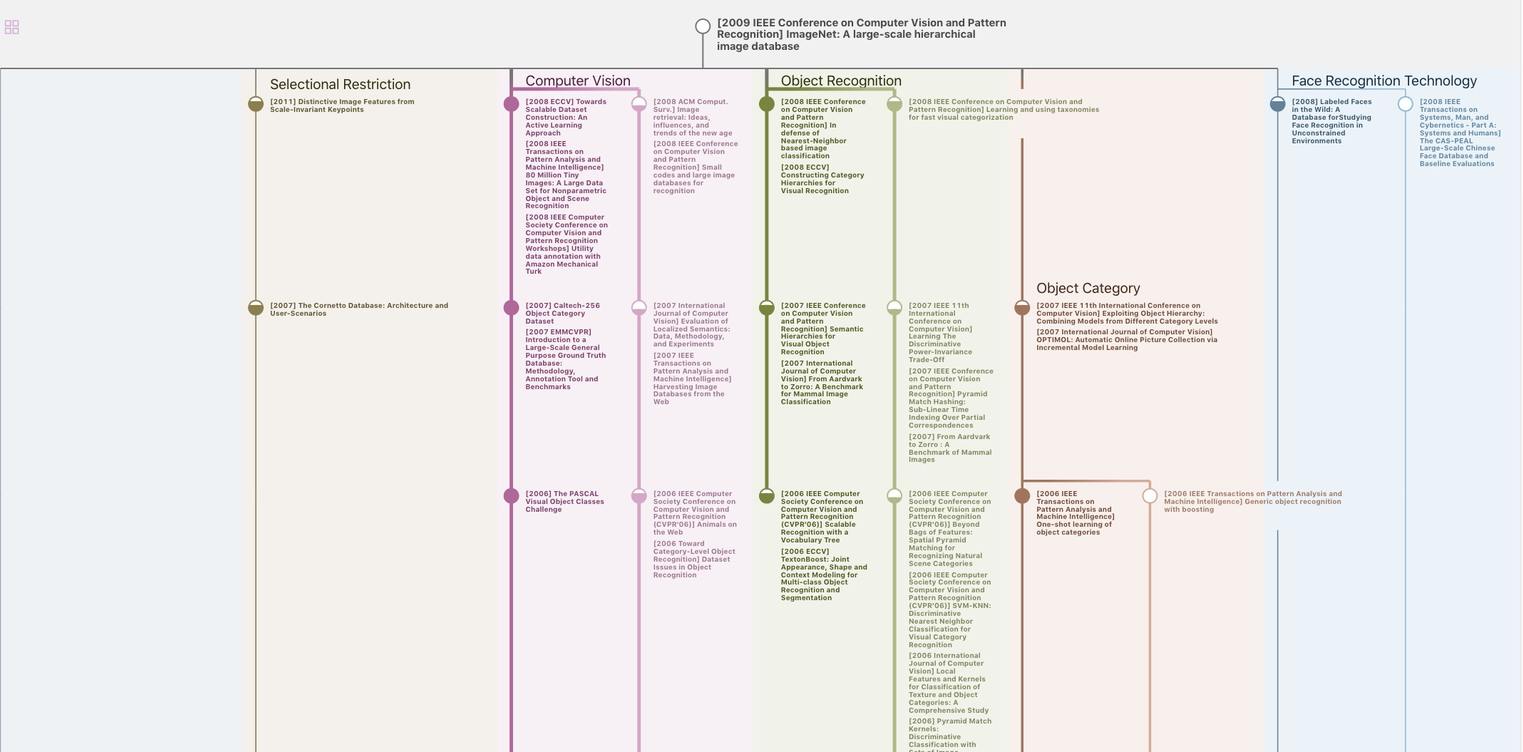
生成溯源树,研究论文发展脉络
Chat Paper
正在生成论文摘要