Learning To Identify Container Contents Through Tactile Vibration Signatures
2016 IEEE INTERNATIONAL CONFERENCE ON SIMULATION, MODELING, AND PROGRAMMING FOR AUTONOMOUS ROBOTS (SIMPAR)(2016)
摘要
We examine using a simple contact sensor coupled with standard machine learning algorithms to classify and count objects shaken in a container. The contact sensor measures the resulting vibrations, and these signatures are used to learn a classifier that maps vibration signatures to known object categories. A linear support vector machine trained on labeled vibration signatures achieves a mean binary classification accuracy of 99% over 66 pairs of objects and a mean multi-class classification accuracy of 94% over 12 classes. It is also shown that useful tasks such as approximate counting of objects over the range 1 to 10 is possible. We see potential applications of these ideas in service robots engaged in cleanup and inventory control in labs, workshops, stores, warehouses and homes.
更多查看译文
关键词
container content identification,tactile vibration signatures,contact sensor,machine learning algorithms,vibration measurement,vibration signature mapping,known object categories,linear support vector machine training,labeled vibration signatures,mean binary classification accuracy,mean multiclass classification accuracy,approximate object counting,cleanup control,inventory control
AI 理解论文
溯源树
样例
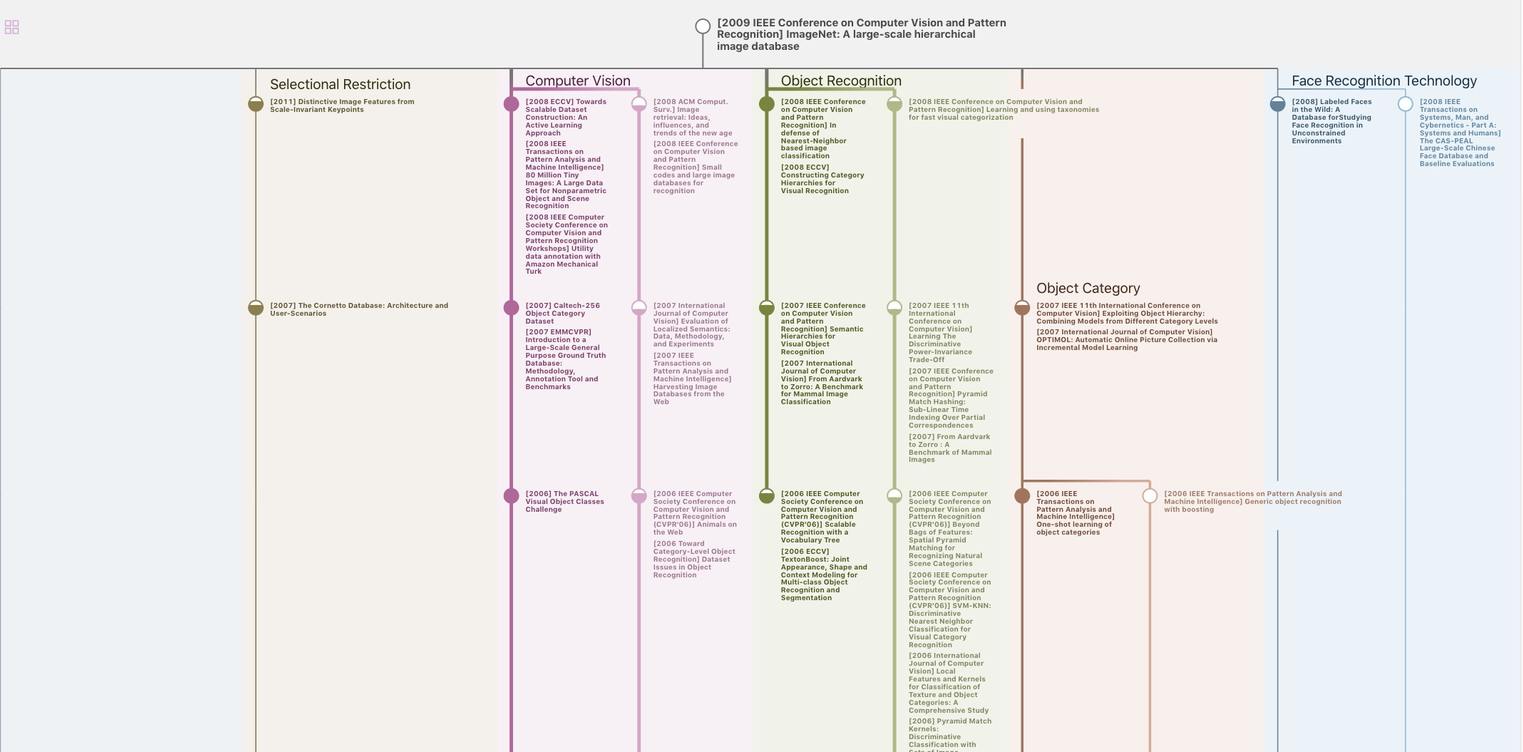
生成溯源树,研究论文发展脉络
Chat Paper
正在生成论文摘要