Temporal persistence modeling for object search.
ICRA(2017)
摘要
We present a novel solution to the object search problem for domains in which object permanence cannot be assumed and other agents may move objects between locations without the robot's knowledge. We formalize object search as a failure analysis problem and contribute temporal persistence modeling (TPM), an algorithm for probabilistic prediction of the time that an object is expected to remain at a given location given sparse prior observations. We show that probabilistic exponential distributions augmented with a Gaussian component can accurately represent probable object locations and search suggestions based entirely on sparsely made visual observations. We evaluate our work in two domains, a large scale GPS location data set for person tracking, and multi-object tracking on a mobile robot operating in a small-scale household environment over a 2-week period. TPM performance exceeds four baseline methods across all study conditions.
更多查看译文
关键词
temporal persistence modeling,object search problem,failure analysis problem,TPM,probabilistic prediction,probabilistic exponential distributions,Gaussian component,probable object locations,visual observations,GPS location data set,person tracking,multiobject tracking,mobile robot,small-scale household environment
AI 理解论文
溯源树
样例
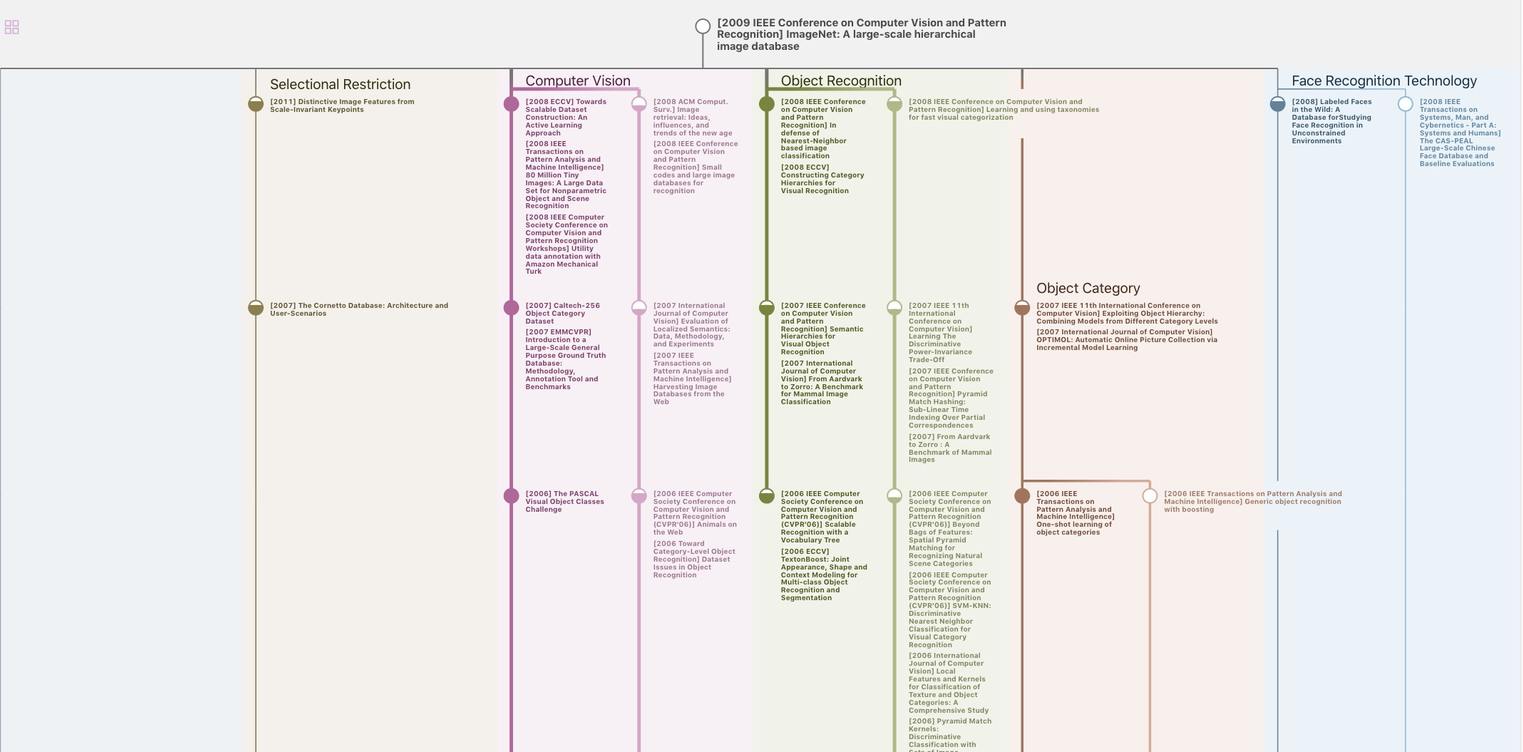
生成溯源树,研究论文发展脉络
Chat Paper
正在生成论文摘要